Unbiased organism-agnostic and highly sensitive signal peptide predictor with deep protein language model
NATURE COMPUTATIONAL SCIENCE(2023)
摘要
Signal peptides (SPs) are essential to target and transfer transmembrane and secreted proteins to the correct positions. Many existing computational tools for predicting SPs disregard the extreme data imbalance problem and rely on additional group information of proteins. Here we introduce Unbiased Organism-agnostic Signal Peptide Network (USPNet), an SP classification and cleavage-site prediction deep learning method. Extensive experimental results show that USPNet substantially outperforms previous methods on classification performance by 10%. An SP-discovering pipeline with USPNet is designed to explore unprecedented SPs from metagenomic data. It reveals 347 SP candidates, with the lowest sequence identity between our candidates and the closest SP in the training dataset at only 13%. In addition, the template modeling scores between candidates and SPs in the training set are mostly above 0.8. The results showcase that USPNet has learnt the SP structure with raw amino acid sequences and the large protein language model, thereby enabling the discovery of unknown SPs. Signal peptides (SPs) are vital for protein-transmembrane communication. In this work, the authors introduce USPNet, a deep learning method based on a protein language model for SP prediction that shows both high sensitivity and efficiency, thereby contributing to the identification of novel SPs.
更多查看译文
AI 理解论文
溯源树
样例
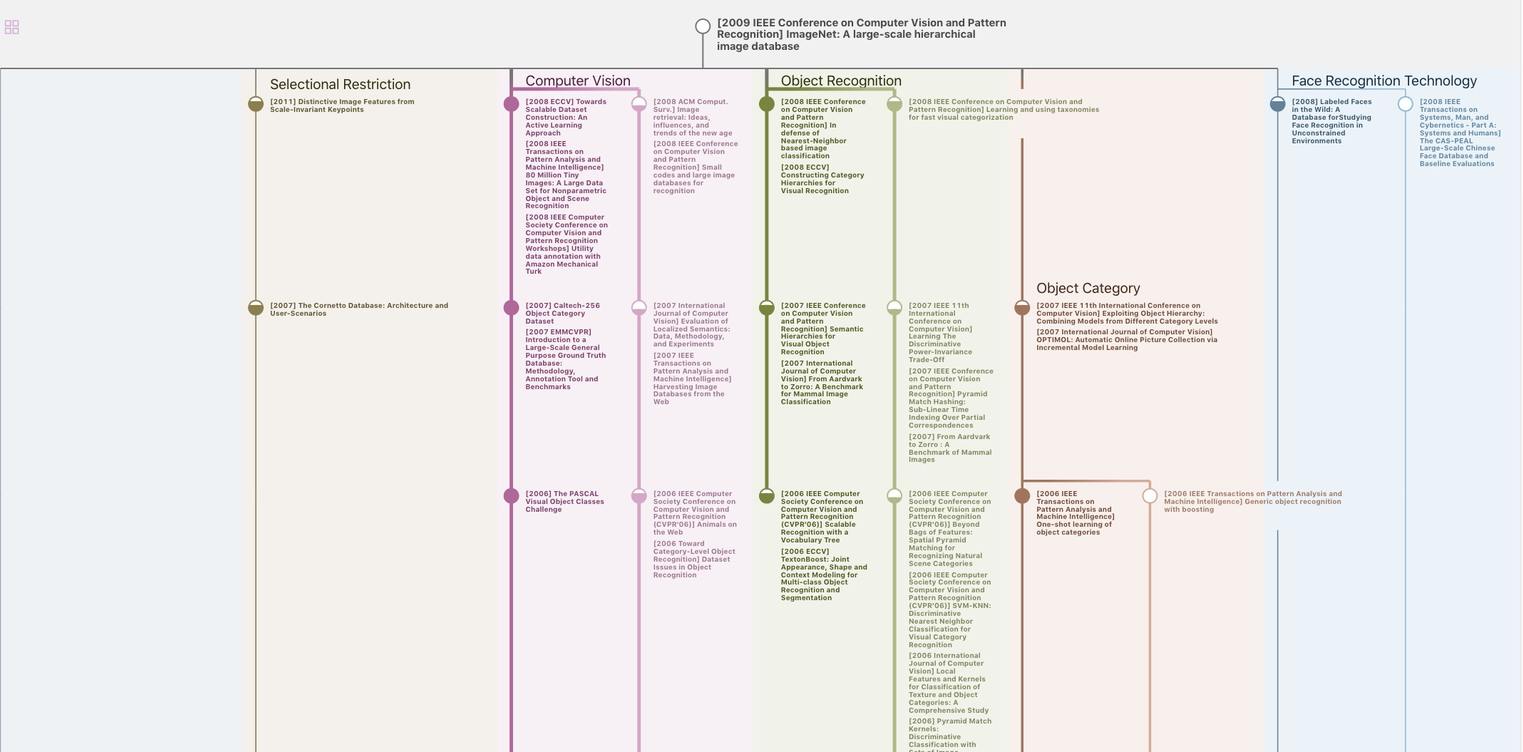
生成溯源树,研究论文发展脉络
Chat Paper
正在生成论文摘要