Evolution of Coal Microfracture by Cyclic Fracturing of Liquid Nitrogen Based on μCT and Convolutional Neural Networks
Rock Mechanics and Rock Engineering(2023)
摘要
Coalbed methane (CBM) is an important unconventional fuel source, and its efficient extraction is of great significance in reducing greenhouse gas emissions, energy requirements, and coal mine safety. Liquid nitrogen (LN2) fracturing is a popular super-cryogenic waterless fracturing method used for enhancing CBM recovery processes. Understanding the behavior of coal fractures under LN2 cyclic fracturing is crucial for revealing the fracture mechanism. In this study, we applied micro-computed tomography (μCT) and the deep learning method to quantify the evolution of volume size, spatial distribution, connectivity, and thickness of 3D fractures in coal under LN2 cyclic fracturing. In addition, the spatial topological and geometric distribution of 3D fractures were further characterized by the pore network model (PNM). A coal microfracture segmentation method from a 2D U-Net model to a 3D U-Net model was proposed to segment fractures automatically and accurately with an average Dice coefficient of 0.942. The results show that LN2 treatment can effectively damage the coal sample and promote the expansion and formation of fractures. The porosity, fracture connectivity, and thickness increase with the number of LN2 cycles, and the increase in the first cycle is significantly higher than in the subsequent cycles. The PNM analysis indicates that the number and equivalent diameter of pores and throats, as well as the coordination numbers, increase with the cycles while the average throat length decreases. Furthermore, the increase in the size of fractures and the formation of large fractures would greatly reduce the P-wave velocity and weaken the uniaxial compressive strength, which decreases by 26.5
更多查看译文
关键词
Coalbed methane (CBM),Liquid nitrogen (LN2),Cyclic fracturing,μCT scanning,Deep learning
AI 理解论文
溯源树
样例
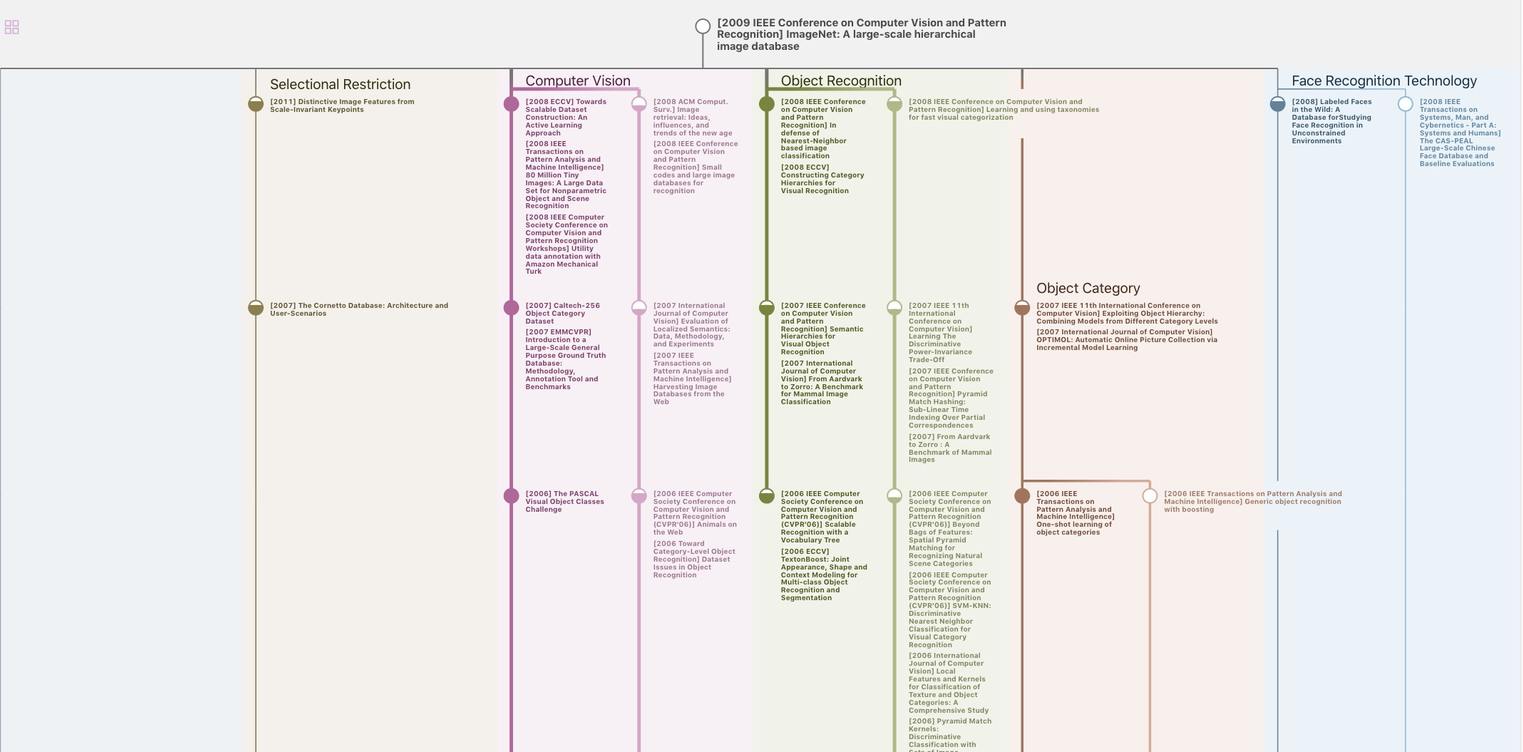
生成溯源树,研究论文发展脉络
Chat Paper
正在生成论文摘要