A survey of inductive knowledge graph completion
Neural Computing and Applications(2024)
摘要
Knowledge graph completion (KGC) can enhance the completeness of the knowledge graph (KG). Traditional transductive KGC assumes that all entities and relations employed during testing have been seen in the training phase. As real-world KGs constantly evolve, it becomes imperative to retrain the KG whenever unseen entities or relations appear. Inductive knowledge graph completion (IKGC) aims to complete missing triples involving unseen entities or relations without retraining and has garnered substantial attention recently. This paper is the first one that provides a comprehensive review of IKGC from both technical and theoretical perspectives. Technically, IKGC is categorized into two groups: structural information-based IKGC and additional information-based IKGC. Theoretically, IKGC is divided into two categories: semi-inductive setting and fully-inductive setting. In particular, each category is further subdivided into distinct granularities, and theoretical scenarios are incorporated into the technical methods to facilitate comparison and analysis. Finally, future research directions are prospected.
更多查看译文
关键词
Inductive,Knowledge graph completion,Unseen entities,Unseen relations
AI 理解论文
溯源树
样例
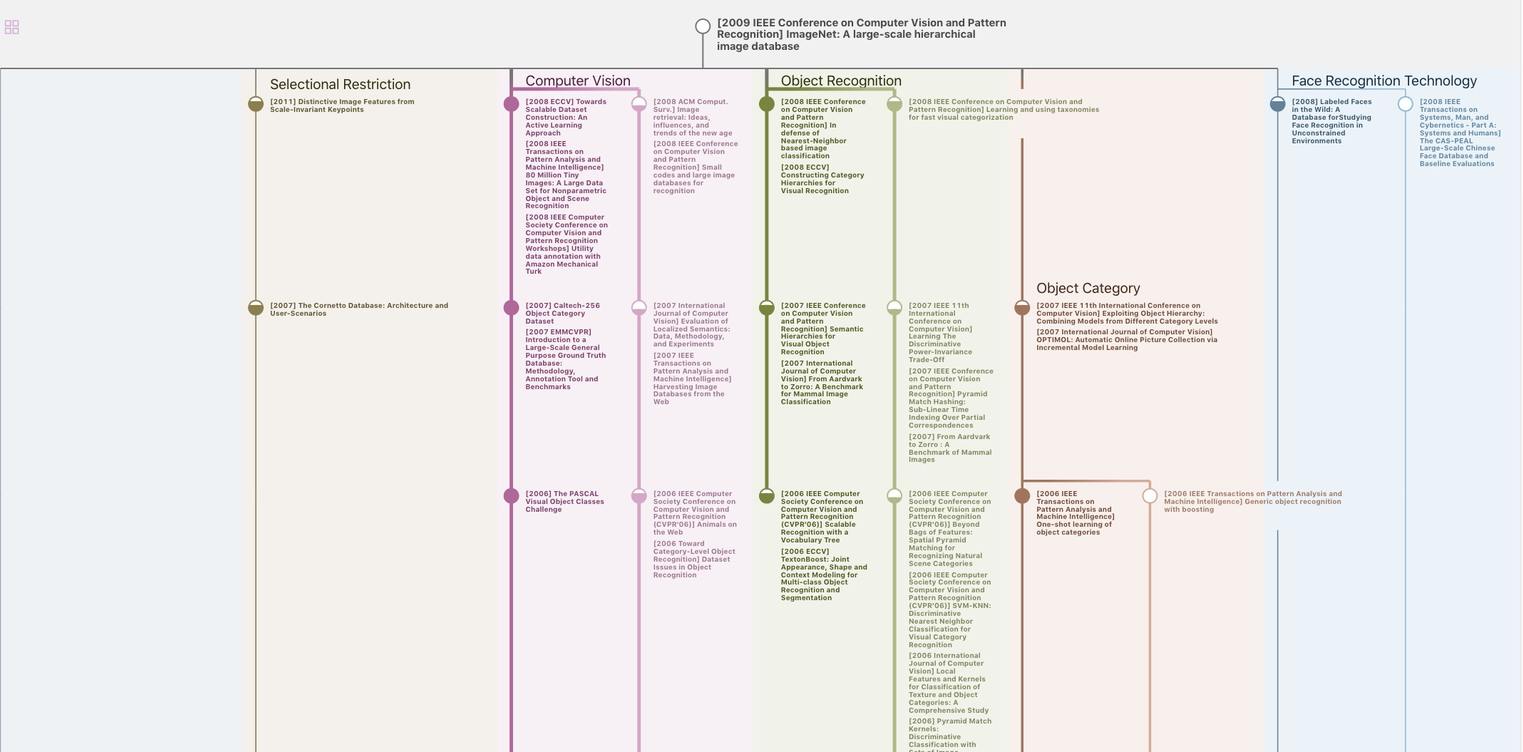
生成溯源树,研究论文发展脉络
Chat Paper
正在生成论文摘要