A novel HB-SC-MCCNN model for intelligent fault diagnosis of rolling bearing
Journal of Mechanical Science and Technology(2023)
摘要
The incompleteness and lack of bearing fault data have become important problems in bearing fault diagnosis. This paper presents an intelligent fault diagnosis method for rolling bearings based on a similarity clustering multi-channel convolution neural network with the hierarchical branch (HB-SC-MCCNN). First, the relevant features are extracted by MCCNN, and combined with the similarity clustering principle, the accurate binary classification is realized in the case of insufficient labeled data. Second, the similarity clustering module and additional loss are added to the SC-MCCNN network to form a hierarchical-branch network, which simplifies the problem of fault multi-classification into binary classification with multiple steps, and to reduces the dependence on the amount of label data in multi-classification. Finally, based on the self-learning characteristics of HB-SC-MCCNN, the unlabeled data and the missing fault types in the training set are re-labeled to realize the re-training of the network. On the benchmark dataset, the comparison experiment results with several salient deep learning models show that the method proposed in this paper successfully realizes the hierarchical diagnosis of bearing faults and presents more substantial competitiveness in the case of insufficient labeled data and missing fault types.
更多查看译文
关键词
Intelligent fault diagnosis of bearing,Insufficient labeled data,Missing fault types,Hierarchical branch structure,Multi-channel convolutional neural networks
AI 理解论文
溯源树
样例
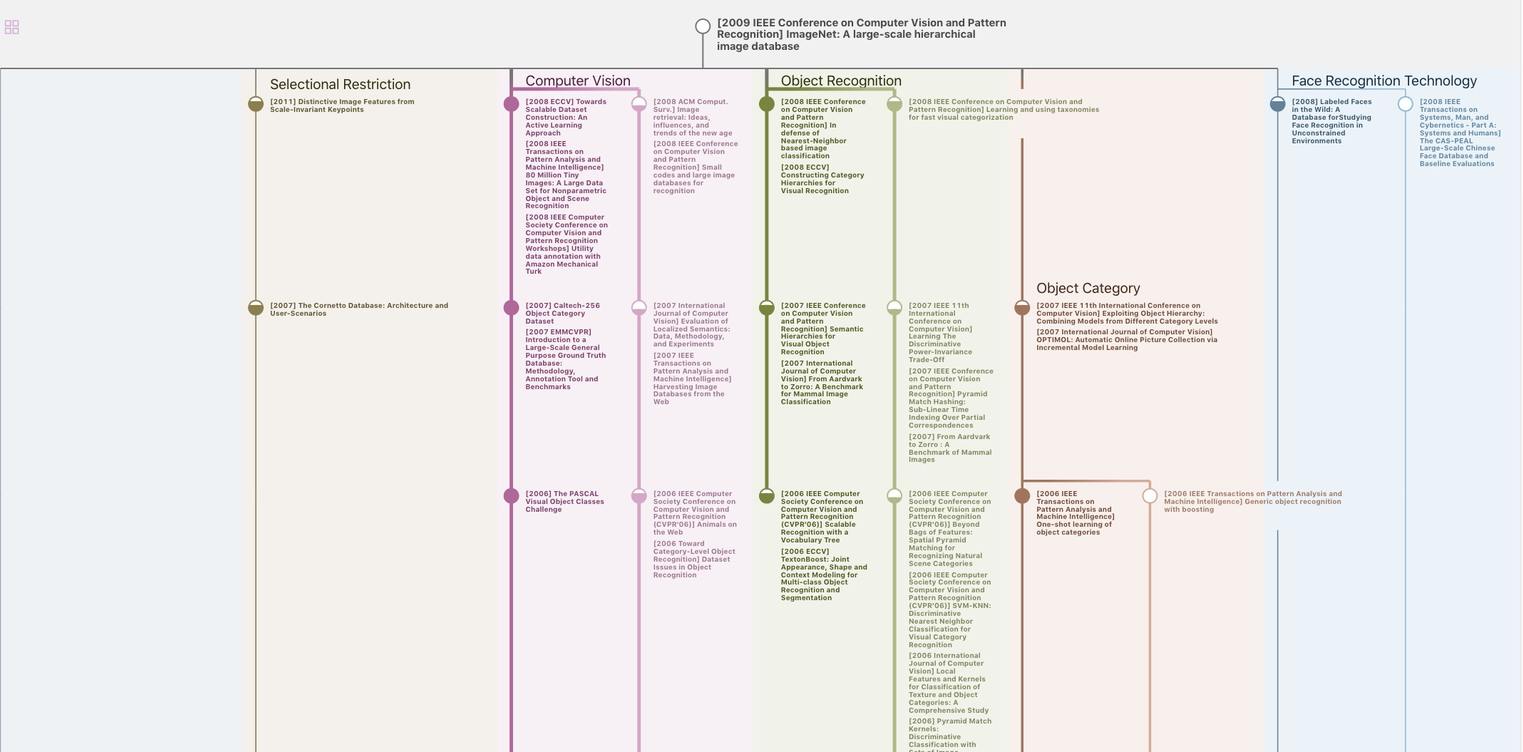
生成溯源树,研究论文发展脉络
Chat Paper
正在生成论文摘要