Safe optimal robust control of nonlinear systems with asymmetric input constraints using reinforcement learning
Applied Intelligence(2024)
摘要
External disturbances and asymmetric input constraints may cause a major problem to the optimal control of the system. Aiming at such problem, this article presents a safe and optimal robust control method based on adaptive dynamic programming (ADP) to ensure the system operated in a safe region and with the optimal performance. Initially, a novel nonquadratic form cost function is imported for the system to address the asymmetric input constraints. Then, to ensure the safety of the system, a control barrier function (CBF) is appended to the cost function to penalize the unsafe behavior. And a damping factor is also introduced to the CBF to balance safety and optimality. Finally, one single critic network is utilized to simplify the complex computational steps, which is different from the traditional actor-critic networks to address the Hamilton-Jacobi-Bellman Equation (HJBE) for obtaining the optimal neural controller. Additionally, based on Lyapunov method, all signals in the closed-loop system are proven to be uniformly ultimately bounded (UUB). At last, the experimental results confirm the effectiveness of the designed approach.
更多查看译文
关键词
Safety,Optimal robust control,Asymmetric input constraints,Adaptive dynamic programming (ADP),Nonlinear systems
AI 理解论文
溯源树
样例
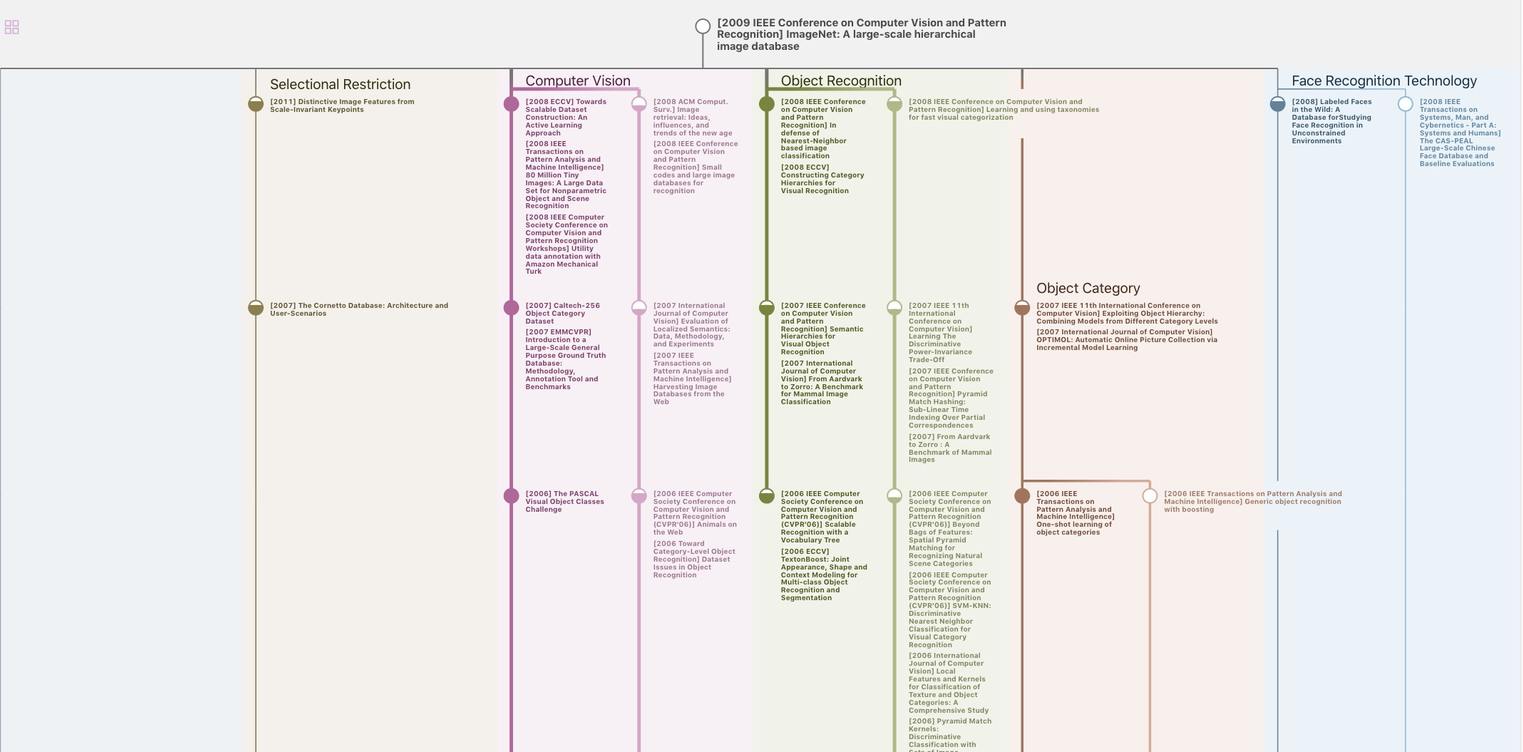
生成溯源树,研究论文发展脉络
Chat Paper
正在生成论文摘要