A Functional Approach to Interpreting the Role of the Adjoint Equation in Machine Learning
Results in Mathematics(2024)
Abstract
The connection between numerical methods for solving differential equations and machine learning has been revealed recently. Differential equations have been proposed as continuous analogues of deep neural networks, and then used in handling certain tasks, such as image recognition, where the training of a model includes learning the parameters of systems of ODEs from certain points along their trajectories. Treating this inverse problem of determining the parameters of a dynamical system that minimize the difference between data and trajectory by a gradient-based optimization method presents the solution of the adjoint equation as the continuous analogue of backpropagation that yields the appropriate gradients. The paper explores an abstract approach that can be used to construct a family of loss functions with the aim of fitting the solution of an initial value problem to a set of discrete or continuous measurements. It is shown, that an extension of the adjoint equation can be used to derive the gradient of the loss function as a continuous analogue of backpropagation in machine learning. Numerical evidence is presented that under reasonably controlled circumstances the gradients obtained this way can be used in a gradient descent to fit the solution of an initial value problem to a set of continuous noisy measurements, and a set of discrete noisy measurements that are recorded at uncertain times.
MoreTranslated text
Key words
Continuous backpropagation,adjoint equation,parameter learning
AI Read Science
Must-Reading Tree
Example
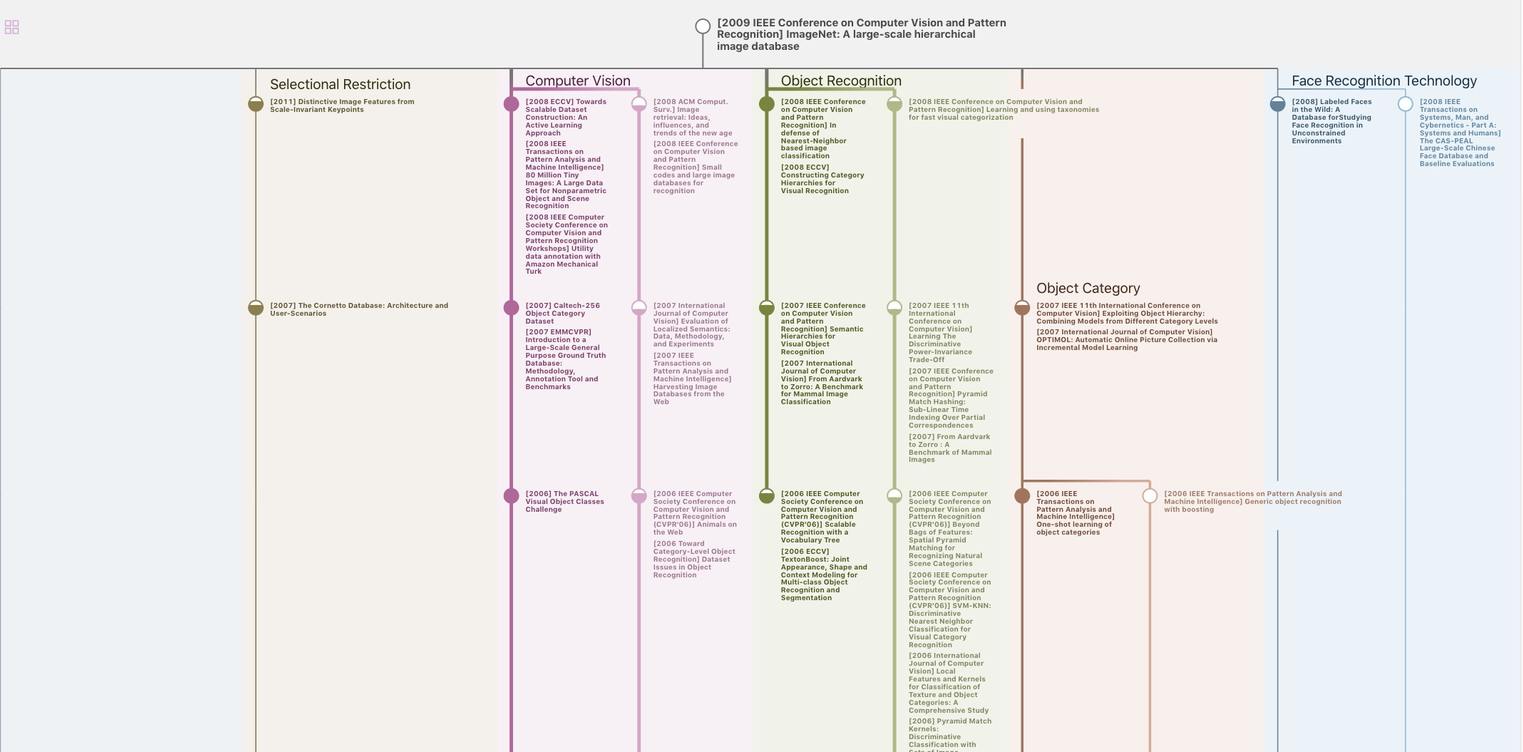
Generate MRT to find the research sequence of this paper
Chat Paper
Summary is being generated by the instructions you defined