Attention Guided Multi-attribute Architecture For Deepfake Detection
2023 IEEE Western New York Image and Signal Processing Workshop (WNYISPW)(2023)
摘要
Deepfake content generated through visual and audio manipulations is speculated to become one of the most threatening artificial intelligence (AI) technologies capable of grave negative impact on society through identity fraud, reputation damage, and undermining of trust in large-scale political campaigns and criminal investigation procedures. A significant measure of the efforts towards solving this problem fails to generalize beyond the training data. We hypothesize that the primary reason behind the drawback is the dearth of efforts toward the exploitation of multiple channels of information from the data. Towards this goal, we investigate a multimodal paradigm exploiting an attention-informed feature generation procedure through a deep network. We further augment the supervisory signal using camera sensor fingerprints which contain additional corroboratory information for the decision-making process. We demonstrate that a non-linear transformation of the decision space augmented through multiple channels allows for a significant boost in the generalization capacity over unseen datasets or attacks. We illustrate the applicability of our framework on the widely known datasets of FaceForensics++ and CelebDF.
更多查看译文
关键词
Deepfake detection,Multimodal fusion,Multi-scale attention,Camera Fingerprint/Noiseprint
AI 理解论文
溯源树
样例
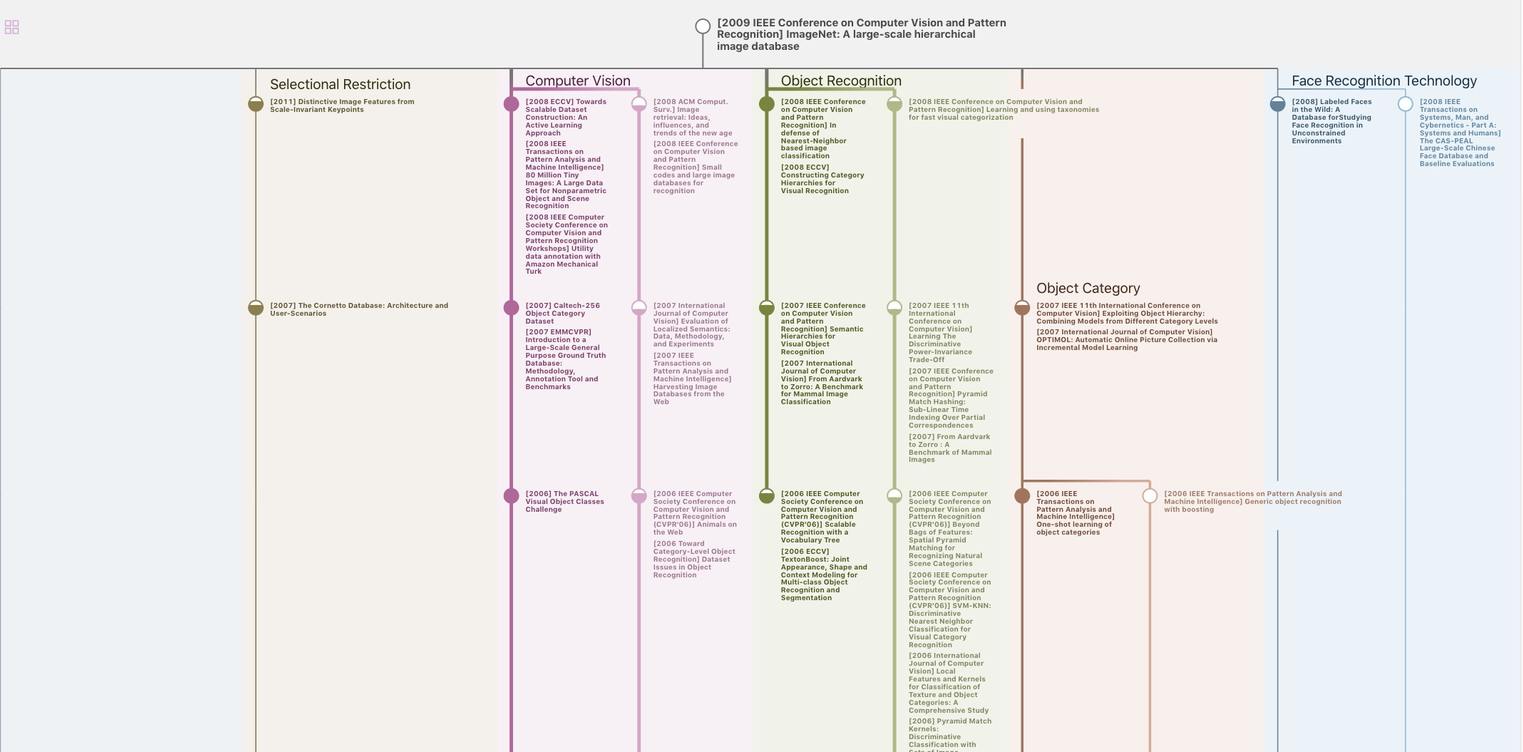
生成溯源树,研究论文发展脉络
Chat Paper
正在生成论文摘要