The Domain Adversarial and Spatial Fusion Semi-Supervised Seismic Impedance Inversion
IEEE TRANSACTIONS ON GEOSCIENCE AND REMOTE SENSING(2024)
摘要
The application of artificial intelligence in seismic impedance inversion makes the prediction of stratigraphic information more efficient. Semi-supervised framework for impedance inversion is the latest breakthrough method in this field. However, the 1-D semi-supervised methods now in use are unable to extract the spatiotemporal properties of the data solely through the network itself. Moreover, the initial model, a critical input for this method, is typically derived through extrapolation and interpolation of well log data. This can lead to significant errors, especially when the well data are sparse and the subsurface structures are complex. Well data only provide information for a limited section of the reservoir, thereby making it challenging to capture the overall behavior accurately. As a result, the creation of an accurate initial model is often fraught with errors. A more desirable approach is to use seismic attribute-guided methods, such as neural networks, which incorporate both seismic and well log data, leading to a more accurate low-frequency model with lateral variations. In this article, we develop a semi-supervised domain adversarial and spatial fusion (DASF) inversion framework. This method uses a 1-D convolutional neural network (CNN)-based global spatiotemporal analysis module and a 2-D CNN-based local spatiotemporal analysis module to complete the inversion and forward task simultaneously. Multiple spatiotemporal characteristics from two submodules can be successfully fused using an adaptive fusion approach. In this network, the step of extracting the initial model is incorporated into the learning process. Moreover, we adopt adversarial learning in the impedance domain to guide the training process, thereby reducing the network's dependence on labels. The experiments on the synthetic and field dataset show that the proposed method can efficiently improve the prediction accuracy of the inversion results compared with conventional methods. Meanwhile, the local spatiotemporal analysis module can be used to create a more trustworthy initial model that incorporates the characteristic of seismic and well-logging data.
更多查看译文
关键词
Impedance,Spatiotemporal phenomena,Feature extraction,Generative adversarial networks,Data models,Training,Pattern analysis,Generative adversarial network (GAN),seismic impedance inversion,semi-supervised learning
AI 理解论文
溯源树
样例
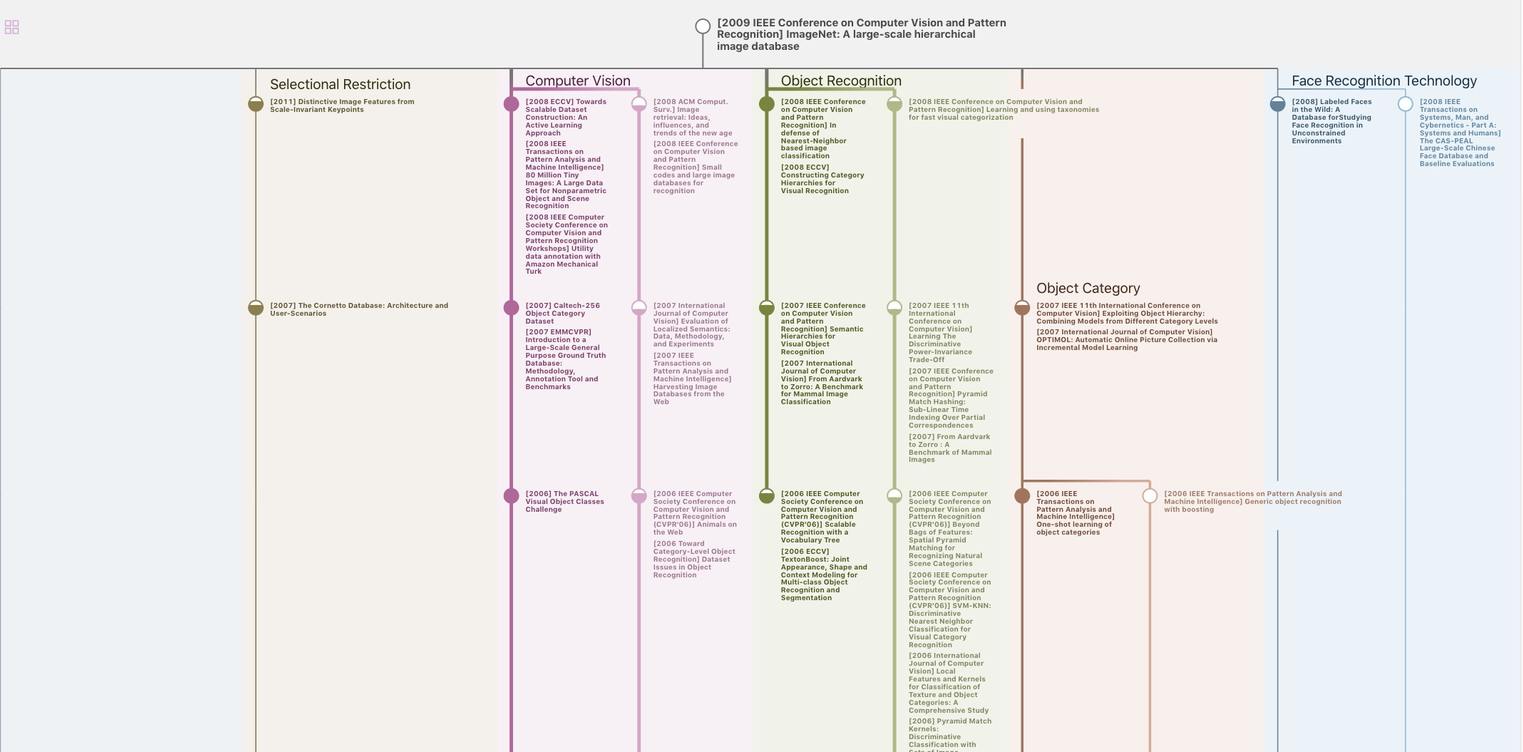
生成溯源树,研究论文发展脉络
Chat Paper
正在生成论文摘要