Deep Learning Based Signal Detector for OFDM Systems
CHINA COMMUNICATIONS(2023)
摘要
In this paper, we propose a novel deep learning (DL)-based receiver design for orthogonal frequency division multiplexing (OFDM) systems. The entire process of channel estimation, equalization, and signal detection is replaced by a neural network (NN), and hence, the detector is called a NN detector (N2D). First, an OFDM signal model is established. We analyze both temporal and spectral characteristics of OFDM signals, which are the motivation for DL. Then, the generated data based on the simulation of channel statistics is used for offline training of bi-directional long short-term memory (Bi-LSTM) NN. Especially, a discriminator (F ) is added to the input of Bi-LSTM NN to look for subcarrier transmission data with optimal channel gain (OCG), which can greatly improve the performance of the detector. Finally, the trained N2D is used for online recovery of OFDM symbols. The performance of the proposed N2D is analyzed theoretically in terms of bit error rate (BER) by Monte Carlo simulation under different parameter scenarios. The simulation results demonstrate that the BER of N2D is obviously lower than other algorithms, especially at high signal-to-noise ratios (SNRs). Meanwhile, the proposed N2D is robust to the fluctuation of parameter values.
更多查看译文
关键词
channel estimation,deep learning,OFDM,optimal channel gain,signal detection
AI 理解论文
溯源树
样例
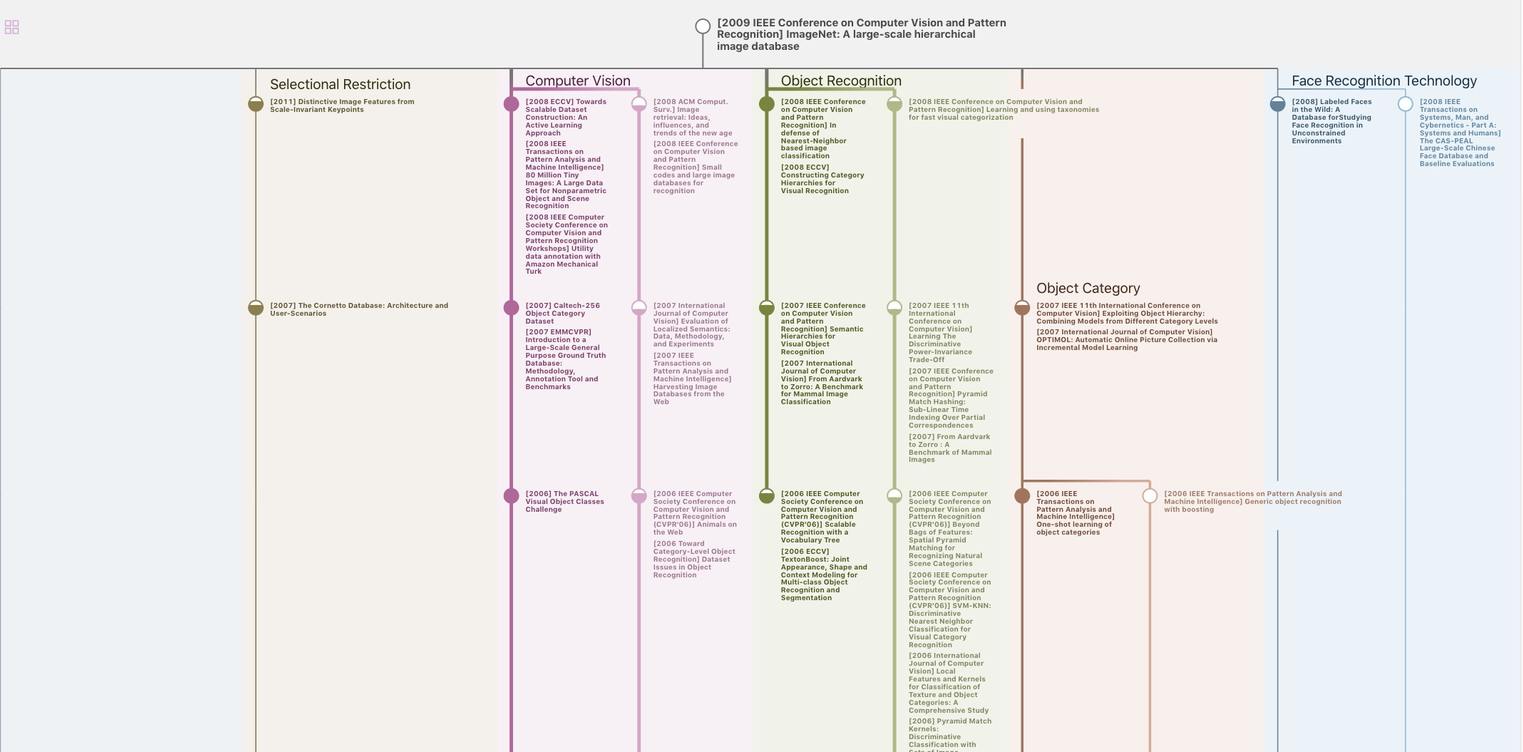
生成溯源树,研究论文发展脉络
Chat Paper
正在生成论文摘要