Chemical composition of arsenic-based acid mine drainage in the downstream of a gold mine: Fuzzy regression and clustering analysis.
Journal of hazardous materials(2023)
摘要
This study employs fuzzy regression and fuzzy multivariate clustering techniques to analyze arsenic-polluted water samples originating from acid rock drainage in waste rock dumps. The research focuses on understanding the complex relationships between variables associated with arsenic contamination, such as water arsenic concentration, pH levels, and soil characteristics. To this end, fuzzy regression models were developed to estimate the relationships between water arsenic concentration and independent variables, thus, incorporating the inherent uncertainties into the analysis. Furthermore, multivariate fuzzy k-means clustering analysis facilitated the identification of fuzzy-based clusters within the dataset, providing insights into spatial patterns and potential sources of arsenic pollution. The pairwise comparisons indicated the strongest correlation of 0.62 between soil total arsenic and pH, while the weakest correlation of 0.13 was observed between soil-soluble arsenic and soil iron, providing valuable insights into their relationships and impact on water arsenic levels. The associated uncertainties in the relationships among the variables were determined based on the degree of belongingness of each data point to various fuzzy sets. Three distinct clusters emerged from the analysis: Cluster 1 comprised Points 5, 6, and 7; Cluster 2 included Points 1, 2, 3, 4, 8, and 9; and Cluster 3 consisted of Points 10, 11, 12, and 13. The findings enhance our understanding of the factors influencing arsenic contamination to provide an effective mitigation strategy in acid rock drainage scenarios. This research also demonstrates the applicability and effectiveness of fuzzy regression and fuzzy multivariate clustering in the analysis of arsenic-polluted water samples.
更多查看译文
AI 理解论文
溯源树
样例
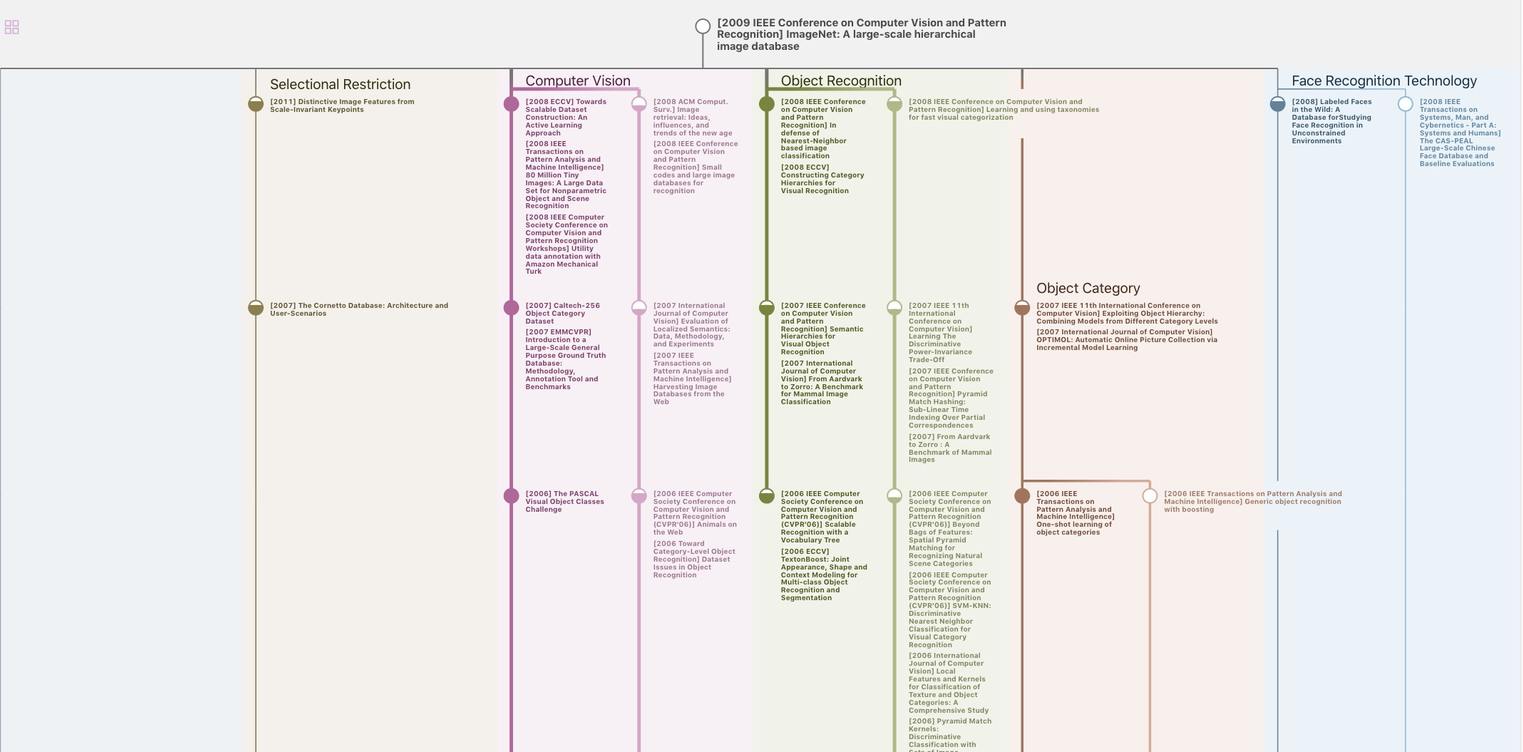
生成溯源树,研究论文发展脉络
Chat Paper
正在生成论文摘要