Extending Whisper with prompt tuning to target-speaker ASR
CoRR(2023)
摘要
Target-speaker automatic speech recognition (ASR) aims to transcribe the
desired speech of a target speaker from multi-talker overlapped utterances.
Most of the existing target-speaker ASR (TS-ASR) methods involve either
training from scratch or fully fine-tuning a pre-trained model, leading to
significant training costs and becoming inapplicable to large foundation
models. This work leverages prompt tuning, a parameter-efficient fine-tuning
approach, to extend Whisper, a large-scale single-talker ASR model, to TS-ASR.
Experimental results show that prompt tuning can achieve performance comparable
to state-of-the-art full fine-tuning approaches while only requiring about 1%
of task-specific model parameters. Notably, the original Whisper's features,
such as inverse text normalization and timestamp prediction, are retained in
target-speaker ASR, keeping the generated transcriptions natural and
informative.
更多查看译文
关键词
target-speaker ASR,prompt tuning,inverse text normalization,timestamp prediction
AI 理解论文
溯源树
样例
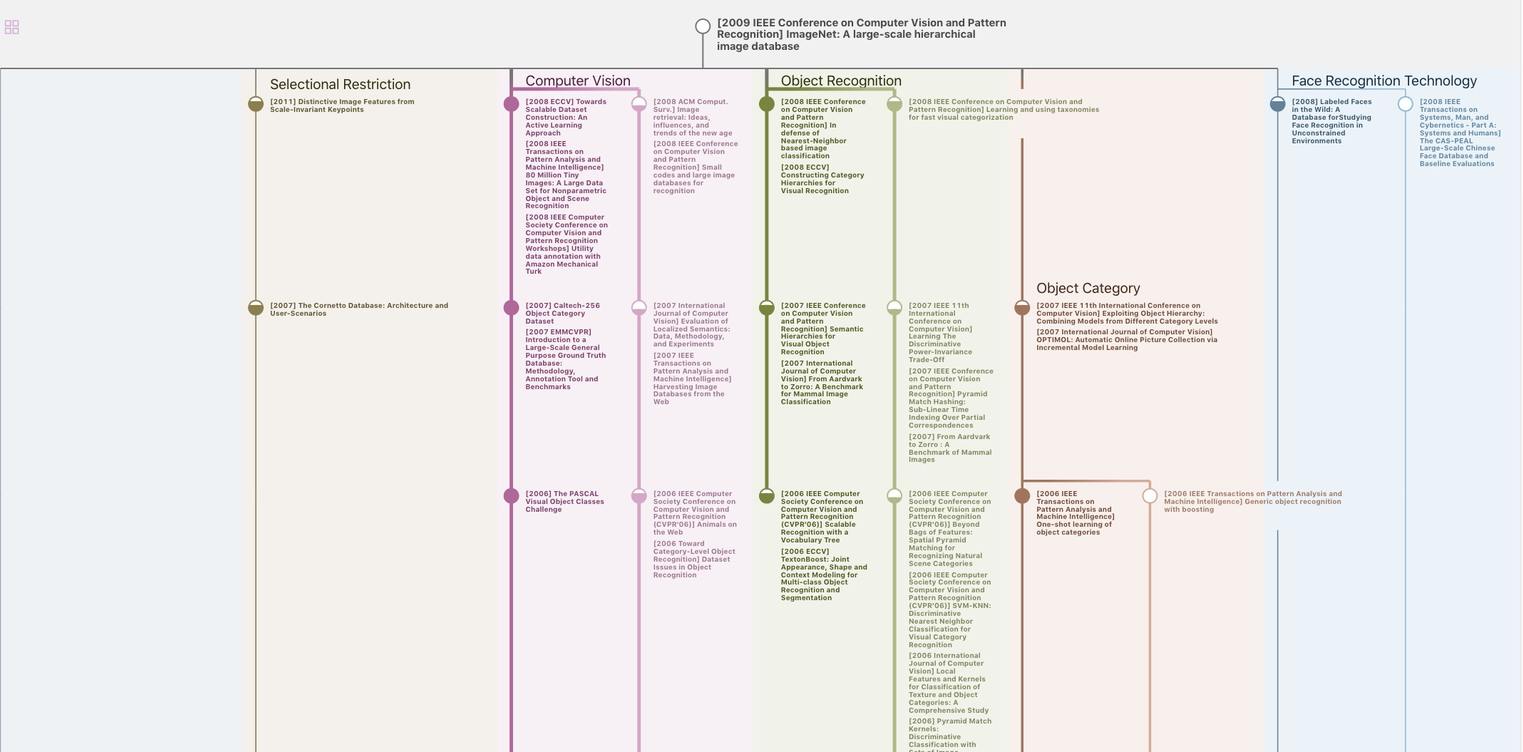
生成溯源树,研究论文发展脉络
Chat Paper
正在生成论文摘要