Estimating Causal Impacts of Scaling a Voluntary Policy Intervention
arxiv(2023)
摘要
Evaluations often inform future program implementation decisions. However,
the implementation context may differ, sometimes substantially, from the
evaluation study context. This difference leads to uncertainty regarding the
relevance of evaluation findings to future decisions. Voluntary interventions
pose another challenge to generalizability, as we do not know precisely who
will volunteer for the intervention in the future. We present a novel approach
for estimating target population average treatment effects among the treated by
generalizing results from an observational study to projected volunteers within
the target population (the treated group). Our estimation approach can
accommodate flexible outcome regression estimators such as Bayesian Additive
Regression Trees (BART) and Bayesian Causal Forests (BCF). Our generalizability
approach incorporates uncertainty regarding target population treatment status
into the posterior credible intervals to better reflect the uncertainty of
scaling a voluntary intervention. In a simulation based on real data, we
demonstrate that these flexible estimators (BCF and BART) improve performance
over estimators that rely on parametric regressions. We use our approach to
estimate impacts of scaling up Comprehensive Primary Care Plus, a health care
payment model intended to improve quality and efficiency of primary care, and
we demonstrate the promise of scaling to a targeted subgroup of practices.
更多查看译文
AI 理解论文
溯源树
样例
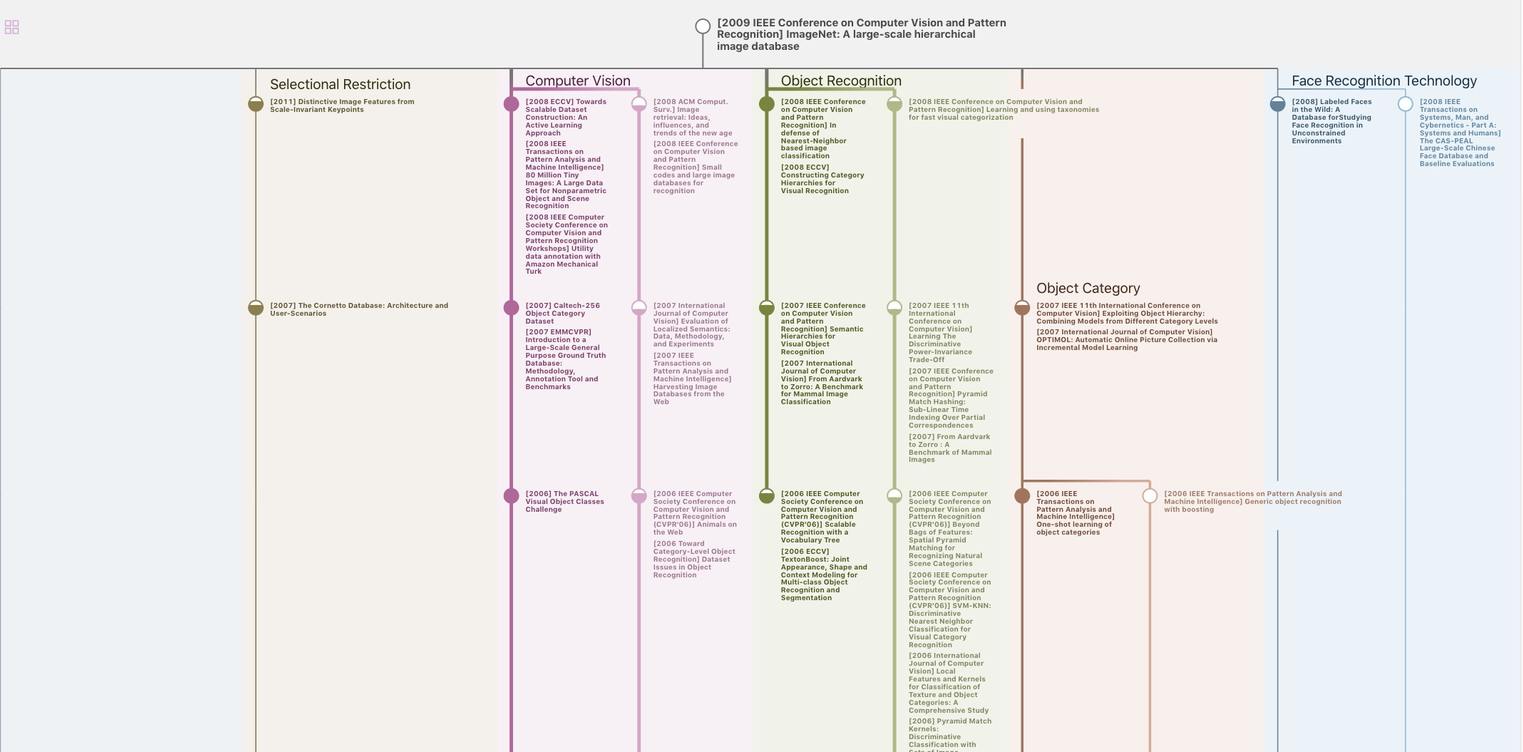
生成溯源树,研究论文发展脉络
Chat Paper
正在生成论文摘要