Vision-Based Driver Drowsiness Detection System
2023 2nd International Conference on Machine Learning, Cloud Computing and Intelligent Mining (MLCCIM)(2023)
摘要
This paper addresses the issue of missed detection and false alarms in the classic driver fatigue detection system by improving three sub-networks of the multitask convolutional neural network. Specifically, the P-Net introduces an additional 3×3 convolutional kernel. The fully connected layer in R-Net is replaced with a pooling layer. In O-Net, the first convolutional kernel is changed to 16×5×5, the second one to 48×3×3, the fourth one to 64×3×3, and the max pooling layer is changed to 2×2. After obtaining facial candidate boxes using the improved MTCNN, the 68 key facial landmarks are accurately localized using the trained ERT (Explicit Regression Tree) algorithm on the 300-W face database. Finally, a Support Vector Machine (SVM) is employed to classify the 68 precise facial feature points. By analyzing a large amount of collected fatigue driving data, a threshold of PERCLOS=22.5% is selected to accurately determine driver fatigue. Experimental results demonstrate that the improved algorithm achieves an average success rate of 93.65% on the collected fatigue driving video streams, an improvement of 2.43% compared to the original MTCNN. Furthermore, the fatigue detection algorithm is deployed on the Linux embedded platform for fatigue driving testing, where the average sensitivity of the fatigue detection system reaches 93.62%.
更多查看译文
关键词
driver fatigue,deep learning,facial features,Support Vector Machines
AI 理解论文
溯源树
样例
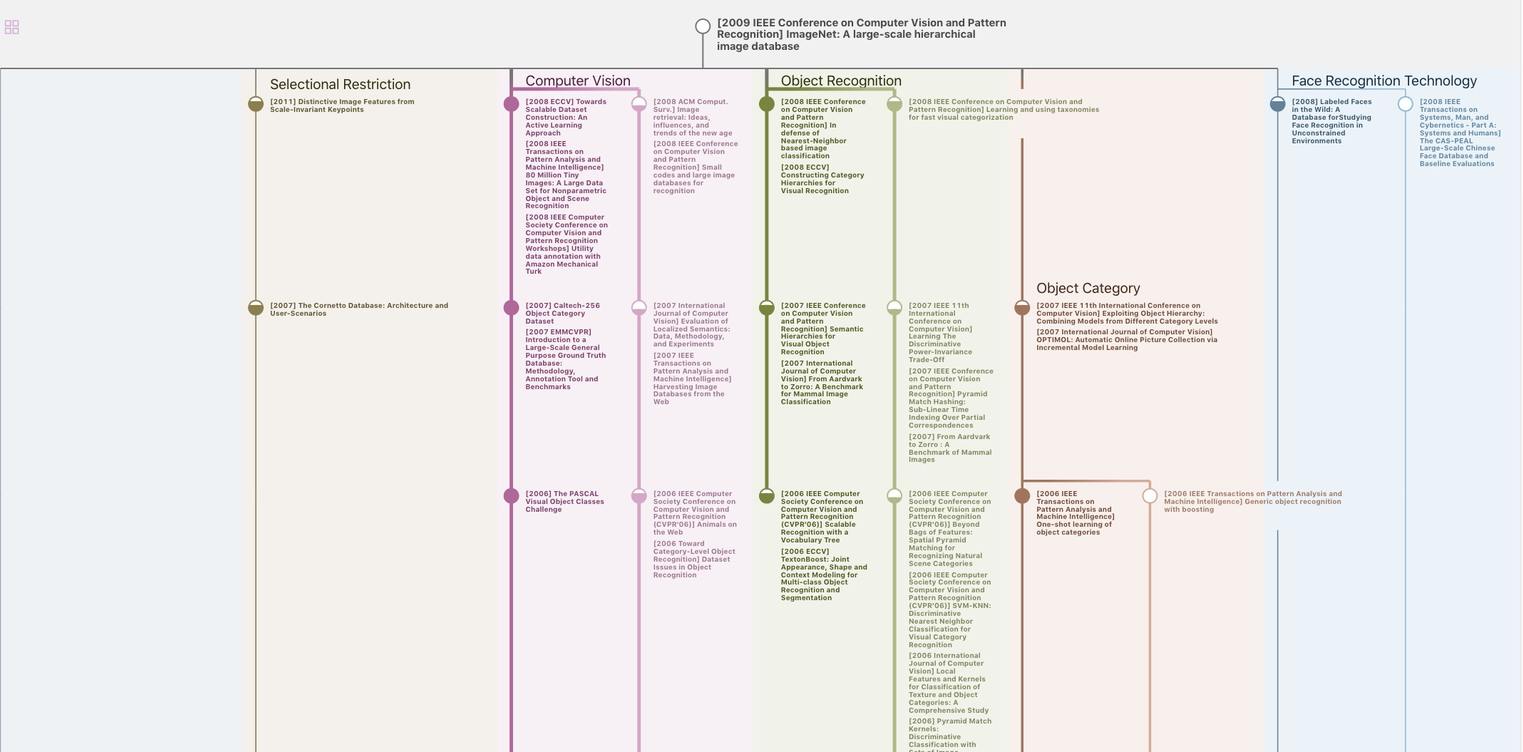
生成溯源树,研究论文发展脉络
Chat Paper
正在生成论文摘要