Deep Classification of Mammographic Breast Density: DCBARNet.
2023 38th International Conference on Image and Vision Computing New Zealand (IVCNZ)(2023)
摘要
Breast density plays a key part in the early prediction of breast cancer development. Experienced radiologists evaluate breast density from the mammographic images for a particular individual based on the Breast Imaging Reporting and Data System (BI-RADS) categories. While super-vised learning algorithms are increasingly finding application for this task, satisfying accuracy and requirement of human intervention remain two of the most challenging roadblocks. In this study, we introduce a novel attention-driven residual learning based method which adaptively pays attention to the selective features in both spatial and channel dimensions. The other feature of our approach is the use of generalized Global Average Pooling layers (utilizing the 2D Discrete Cosine Transform) to further enhance the classifier performance. The proposed method was validated on two publicly available datasets namely CBIS-DDSM and INBreast. Classification accuracies of 87.05% and 79.00% were obtained respectively for the two datasets with five-fold cross-validation. Our approach is fully automatic and the classification performance obtained is an improvement on the state-of-the-art algorithms. We also designed a dual-view network architecture explicitly for the purpose of leveraging the complementary information present in the two different views (CC and MLO) of a single breast. It was observed that the dual-view approach produces slightly better result than the single-view approach.
更多查看译文
关键词
Mammogram,density classification,residual learning,attention mechanism
AI 理解论文
溯源树
样例
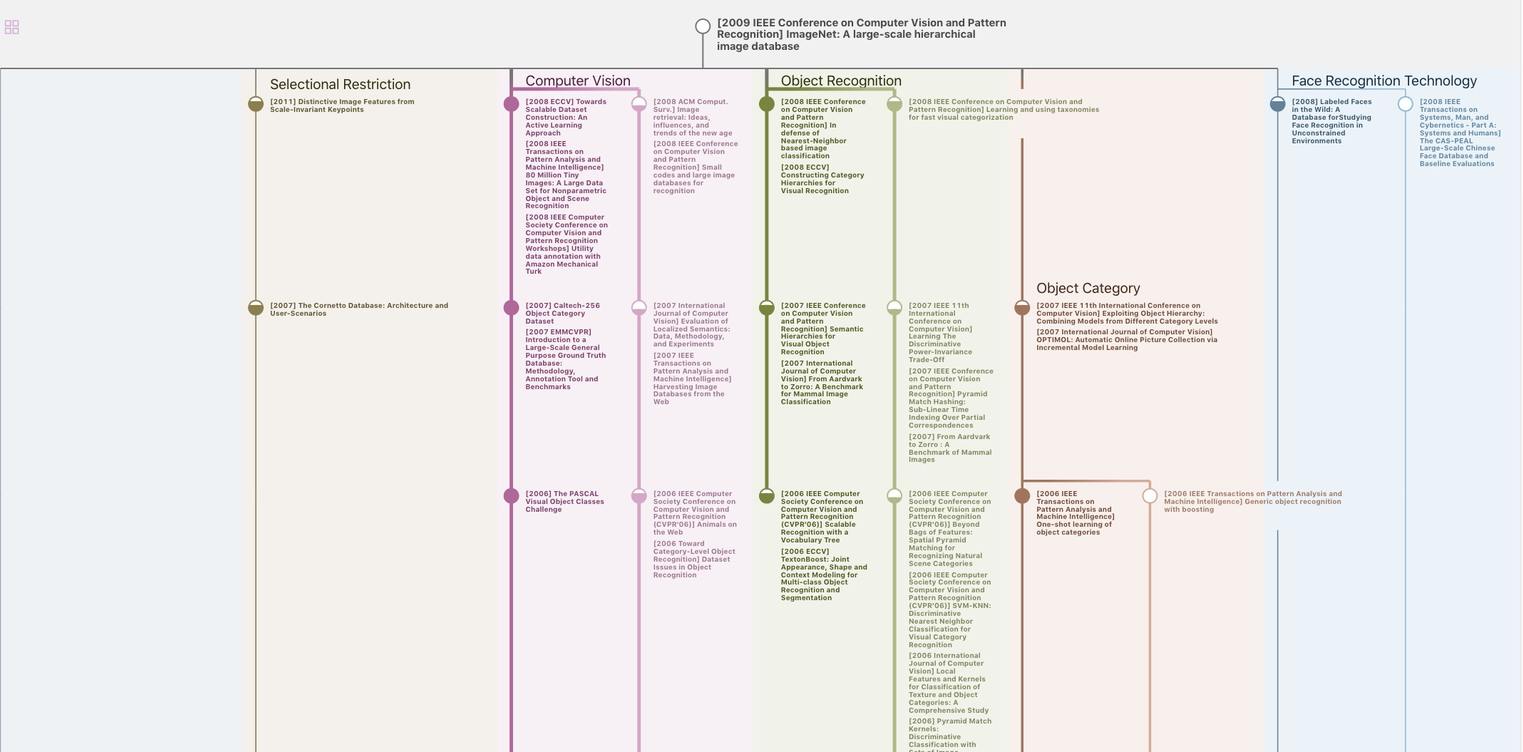
生成溯源树,研究论文发展脉络
Chat Paper
正在生成论文摘要