A Knowledge Graph Embedding Model Based on Inception Structure with Channel Attention
2023 16th International Conference on Advanced Computer Theory and Engineering (ICACTE)(2023)
摘要
In this paper, we propose an improved knowledge graph embedding model, InceSE, by replacing the convolution in Inception with the dilated convolution to improve the ability to capture feature interaction information. InceSE uses the channel attention mechanism SE-Block to adaptively recalibrate the channel-level feature responses by modeling the interdependencies between channels, enhancing useful features while suppressing useless features to improve the performance of the KGE model. The experiments used the benchmark dataset FB15k-237 and WN18RR to validate the link prediction validity of the model. Compared with ConvE, InceSE's Hits@1 increased by 5.44% and 6%, Hits@10 increased by 4.89% and 8.08%, respectively, and MRR increased respectively 8.22% and 9.77%, and MR increased by 17.48% and 58.87% respectively.
更多查看译文
关键词
Knowledge Graph Embedding (KGE),Inception,Channel Attention Mechanism
AI 理解论文
溯源树
样例
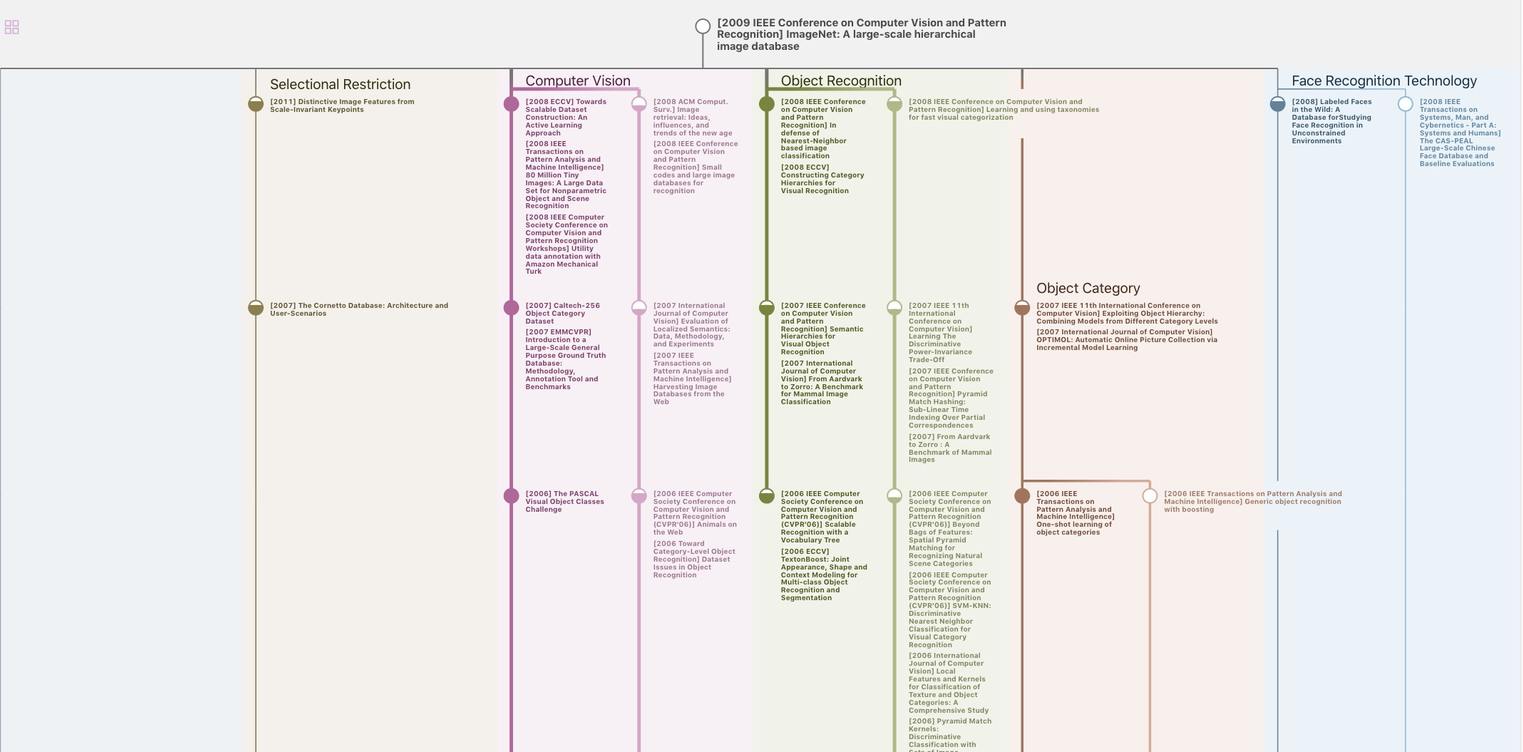
生成溯源树,研究论文发展脉络
Chat Paper
正在生成论文摘要