Deep Learning Inversion for Multivariate Magnetic Data
IEEE TRANSACTIONS ON GEOSCIENCE AND REMOTE SENSING(2024)
Abstract
Three-dimensional inversion of magnetic data can obtain the distribution of subsurface magnetic targets. Deep learning is an effective way to achieve 3-D inversion, which trains a neural network to learn the features of magnetic anomaly data and then generates a 3-D model based on these features. Large training samples are required to achieve persuasive results due to the limited observational data and the multisolution nature of the inverse problem. To reduce the nonuniqueness of the inversion, this article proposes a multivariate magnetic data-based deep learning 3-D inversion strategy. With the proposed strategy, more domain knowledge is incorporated into the training data of the neural network to improve the inversion accuracy. The input data of the neural network adopt multivariate observation data, including multiscale data and multitype data such as magnetic three-component data, magnetic gradient tensor data, and so on, and output a 3-D model to realize 3-D to 3-D mapping. Then, the neural network structure uses the 3-D convolution to extract 3-D spatial information. Both tests on simulation and measured data verify that the proposed strategy can effectively improve the accuracy of the 3-D magnetic inversion.
MoreTranslated text
Key words
Three-dimensional displays,Magnetic domains,Solid modeling,Data models,Neural networks,Mathematical models,Feature extraction,3-D convolution,3-D magnetic inversion,deep learning,multivariate data
AI Read Science
Must-Reading Tree
Example
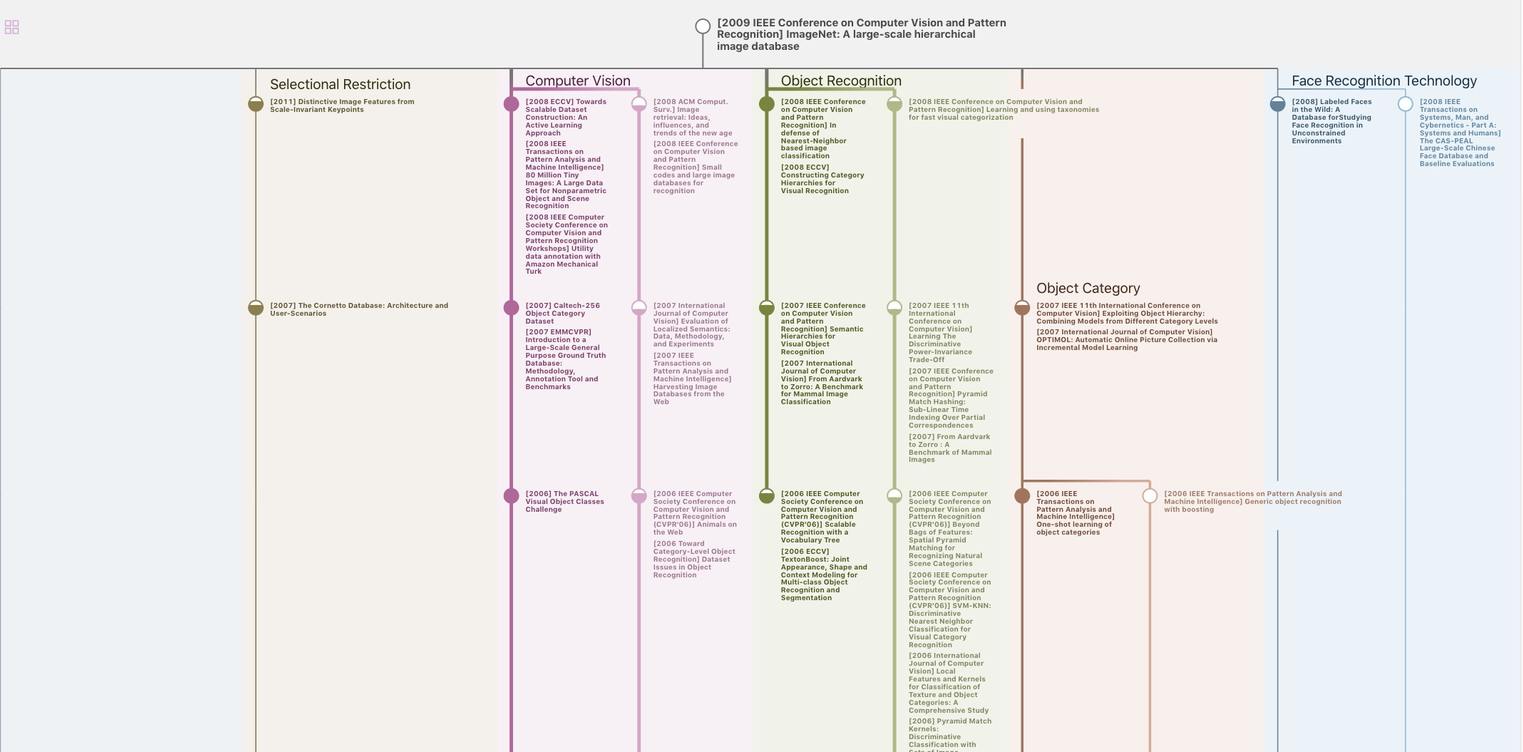
Generate MRT to find the research sequence of this paper
Chat Paper
Summary is being generated by the instructions you defined