A Practical Online Incremental Learning Framework for Precipitation Nowcasting
IEEE TRANSACTIONS ON GEOSCIENCE AND REMOTE SENSING(2024)
摘要
Precipitation nowcasting plays an important role in our life. Many deep learning-based methods are proposed for precipitation nowcasting by predicting radar echo sequence over the past years, and achieving better performance than traditional approaches. However, all of them are based on a static model, which is trained in offline learning and does not adapt to real-time changing precipitation data. Recently, online incremental learning (OIL) has been proposed to dynamically update the model by continually learning new data and preventing the forgetting of historical knowledge in an online fashion. While effective, existing OIL approaches that focus on a classification task are not suitable for the regression task of precipitation nowcasting. To fill this gap, we try to propose a novel OIL framework for precipitation nowcasting. By analyzing its characteristics, we find three challenges: 1) the distributions of radar echo maps in different rainfall events are different; 2) in each rainfall event, there is always an inevitable delay between the timestamps of the training and testing samples; and 3) the real-time requirement for model prediction is very high, which has strict limitations on the training speed of the model. Based on these observations, we propose a practical OIL framework based on gradient activation mapping (GAM). It can be mainly divided into three components: 1) recall training strategy (RTS) is used to eliminate the interference caused by distributions of different events; 2) iterative approximation training (IAT) is designed to align the timestamps of the training and testing; and 3) moreover, we propose gradient activation mapping weight (GAMW) to improve the training effectiveness. Extensive experiments show that the proposed framework can improve the performance of the model stably and effectively. Especially in those heavy rainfall regions where it usually causes more threat to human activity, the improvement is significant.
更多查看译文
关键词
Online incremental learning (OIL),precipitation nowcasting,spatial-temporal prediction
AI 理解论文
溯源树
样例
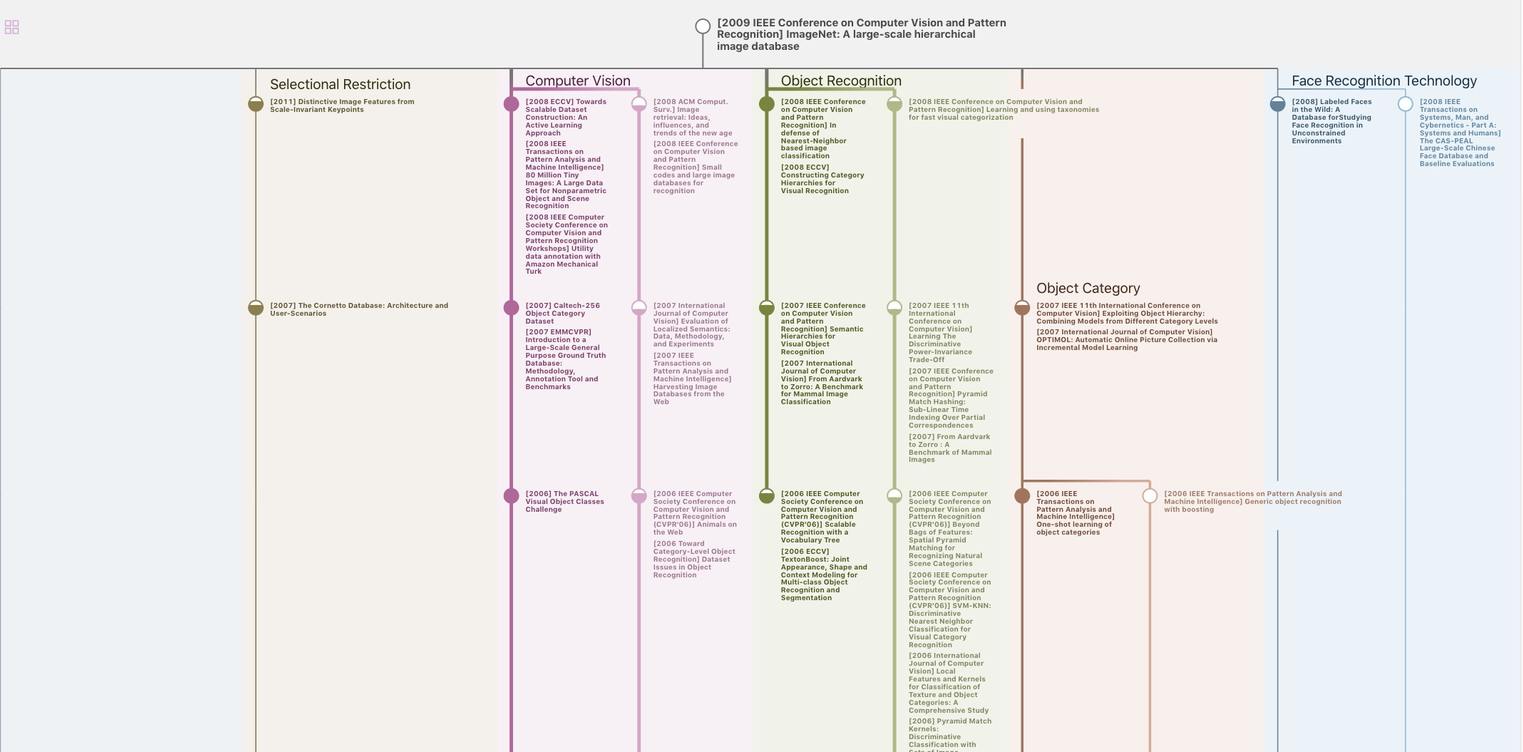
生成溯源树,研究论文发展脉络
Chat Paper
正在生成论文摘要