Meta-learning Based Domain Generalization Framework for Fault Diagnosis With Gradient Aligning and Semantic Matching
IEEE TRANSACTIONS ON INDUSTRIAL INFORMATICS(2024)
摘要
Intelligent fault diagnosis models have de- monstrated superior performance in industrial prognostics health management scenarios. However, these models may struggle to generalize in complicated industrial environments, when encountering new working conditions and handling low-resource and heterogeneous data. To cope with the aforementioned issues, we focus on constructing a universal training framework with a domain generalization technique that will encourage fault diagnosis models to generalize well in unseen working conditions. First, a model-agnostic meta-learning-based training framework called Meta-GENE is proposed for homogeneous and heterogeneous domain generalization. Second, a gradient aligning algorithm is introduced in a meta-learning framework to learn a domain-invariant strategy for robust prediction under unseen working conditions. Third, a semantic matching technique is proposed for utilizing heterogeneous data to alleviate low-resource problems. Our method has yielded excellent performance on the PHM09 fault diagnosis dataset and achieved superior results on a set of generalization tasks across various working conditions.
更多查看译文
关键词
Domain generalization (DG),fault diagnosis,industrial intelligence,meta-learning
AI 理解论文
溯源树
样例
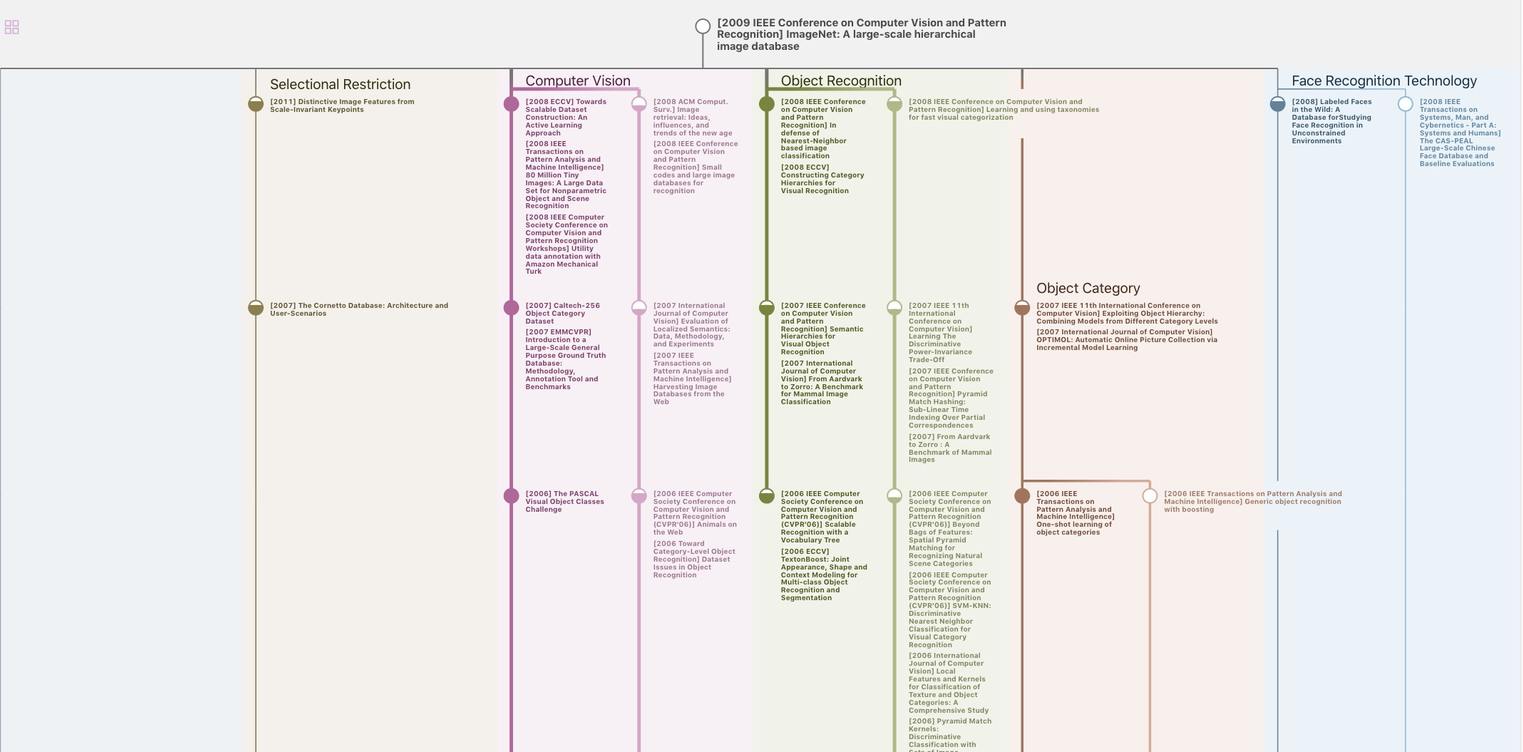
生成溯源树,研究论文发展脉络
Chat Paper
正在生成论文摘要