ToolBot: Learning Oriented Keypoints for Tool Usage From Self-Supervision
IEEE TRANSACTIONS ON INDUSTRIAL INFORMATICS(2024)
摘要
Tool usage is critical for enabling robots to complete challenging tasks that exceed their innate capabilities. Task-oriented grasp and manipulation are two primitive actions in tool usage tasks. In this article, we present an end-to-end framework for jointly inferring two primitive actions through self-supervision, which can guide robots to complete tool usage tasks. We formulate primitive actions as oriented keypoint representations so that the existing pose-based policies can be easily used to achieve tool usage tasks. To address the low task completion rates in self-supervision, we propose a novel technique based on self-supervision properties and forward kinematic models to generate additional effective training samples. The resulting system, ToolBot, is evaluated with the following four different kinds of tools: hammer, knife, screwdriver, and wrench, and it achieves an average task success rate of 82.88% in simulation for four tools and 77.72% in real-world experiments.
更多查看译文
关键词
Data-efficient robot learning,self-superv ision,tool usage,visuomotor policy
AI 理解论文
溯源树
样例
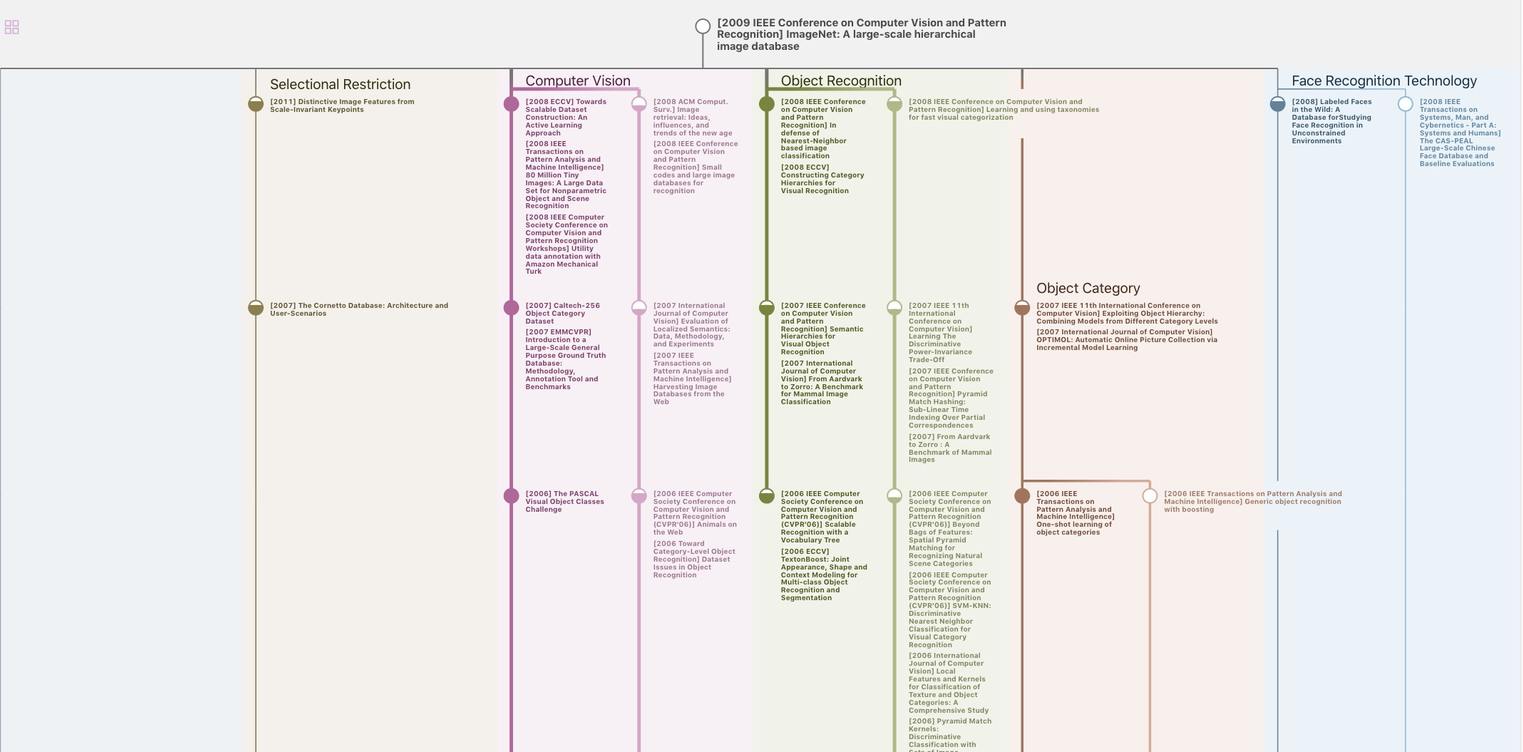
生成溯源树,研究论文发展脉络
Chat Paper
正在生成论文摘要