Physics-Dominated Neural Network for Spatiotemporal Modeling of Battery Thermal Process
IEEE TRANSACTIONS ON INDUSTRIAL INFORMATICS(2024)
摘要
Modeling the temperature distribution of a battery is critical to its safe operation. Data-based modeling methods are computationally efficient, but require a large number of sensors, whereas physics-based modeling methods have better generalization, but the unknown dynamics of the actual scene are ignored. A physics-dominated neural network is presented to integrate electric-thermal mechanism of the battery and data information through a weight adaptive function. The electric-thermal coupling equation of the battery under complex conditions is taken as the prior knowledge to update parameters of the network, whereas the characteristic data obtained by the unique sensor are used to compensate the unknown disturbance in the actual scene. A well-trained model can predict the temperature distribution of the battery over entire space with a single sensor, and can also provide reasonable predictions for longer periods of time under extreme conditions. Experiments show that the proposed method outperforms traditional methods that rely only on pure data or pure physics.
更多查看译文
关键词
Batteries,Temperature sensors,Temperature distribution,Sensors,Mathematical models,Behavioral sciences,Neural networks,Physics-dominated neural network,power and energy,sparse sensor data,thermal process modeling,transient heat source
AI 理解论文
溯源树
样例
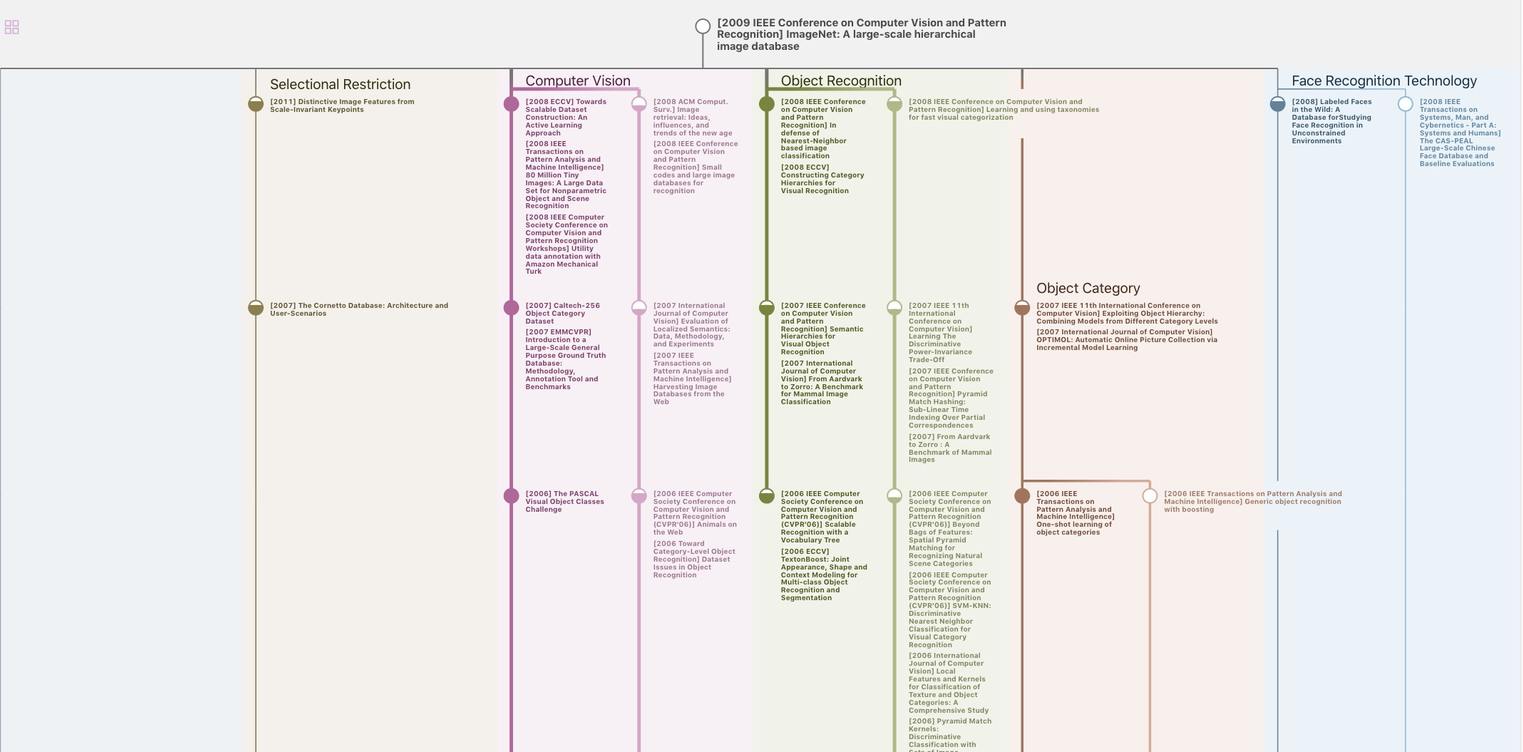
生成溯源树,研究论文发展脉络
Chat Paper
正在生成论文摘要