A Network Traffic Classification Method Based on Dual-Mode Feature Extraction and Hybrid Neural Networks
IEEE TRANSACTIONS ON NETWORK AND SERVICE MANAGEMENT(2023)
摘要
Network traffic classification is a key foundation of traffic management and network security. With the development of traffic encryption technologies and more attention given to user privacy, traditional rule-based and payload-based traffic classification methods have become less effective. To address this problem, recent studies have introduced deep learning-based methods. However, most of these studies do not consider both the flow-level and packet-level characteristics, which we believe are significant in network traffic classification. To further improve the accuracy of traffic classification, this paper proposed DM-HNN, a hybrid neural network based on dual-mode features. First, we treat the packet length sequence as the flow-level feature and the initial byte of the packet as the packet-level feature. Then, we diverge into two paths to analyze the dual-mode features using neural networks. Finally, we combine the two-path features and output the final classification results. We have performed the experiments on public datasets, the results comparing to single-mode and dual-mode traffic classifiers indicate that DM-HNN can achieve excellent performance and has certain effectiveness.
更多查看译文
关键词
Feature extraction,Telecommunication traffic,Neural networks,Switches,Logic gates,Training,Protocols,Dual-mode features,hybrid neural networks,network traffic classification
AI 理解论文
溯源树
样例
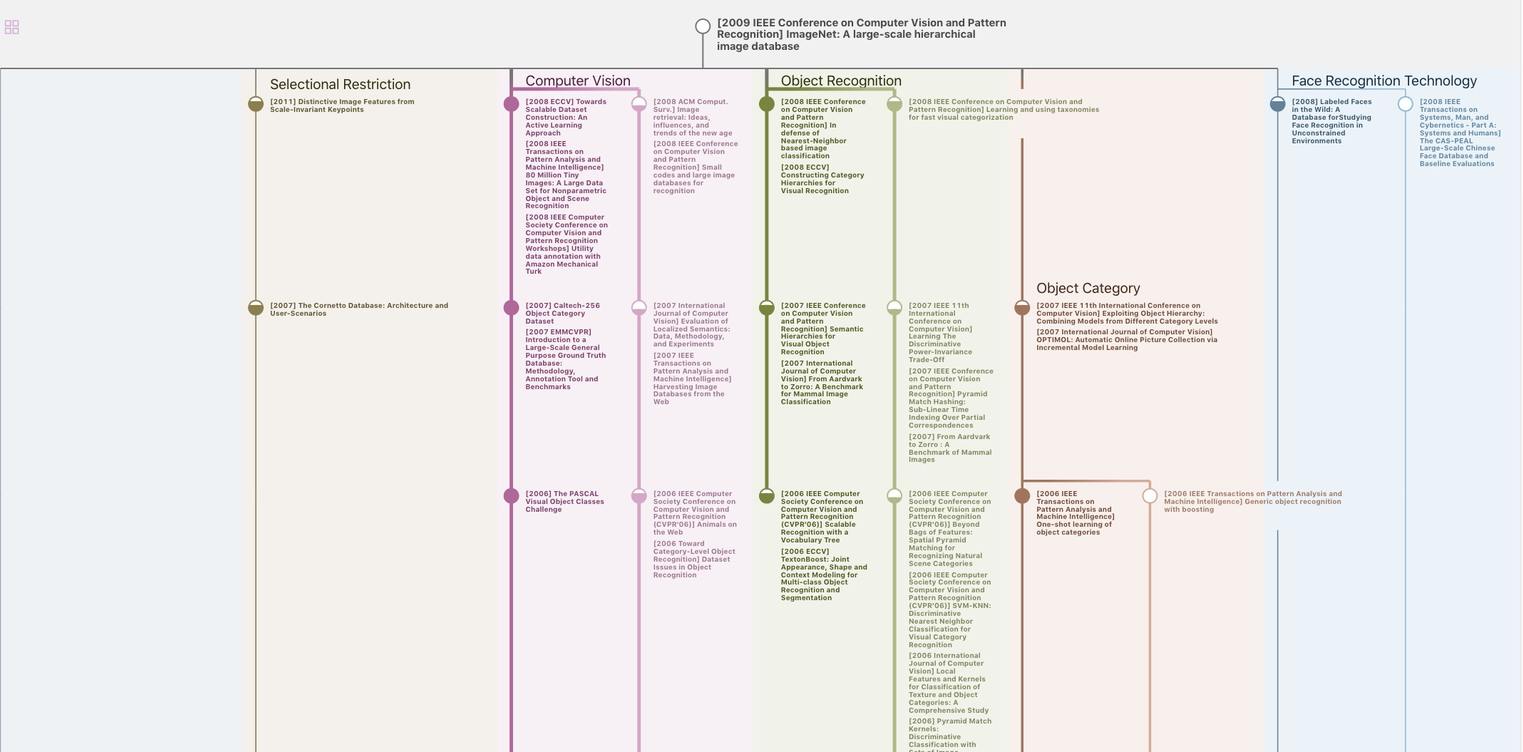
生成溯源树,研究论文发展脉络
Chat Paper
正在生成论文摘要