Causal inference of general treatment effects using neural networks with a diverging number of confounders
JOURNAL OF ECONOMETRICS(2024)
摘要
Semiparametric efficient estimation of various multi-valued causal effects, including quantile treatment effects, is important in economic, biomedical, and other social sciences. Under the unconfoundedness condition, adjustment for confounders requires estimating the nuisance functions relating outcome or treatment to confounders nonparametrically. This paper considers a generalized optimization framework for efficient estimation of general treatment effects using artificial neural networks (ANNs) to approximate the unknown nuisance function of growingdimensional confounders. We establish a new approximation error bound for the ANNs to the nuisance function belonging to a mixed smoothness class without a known sparsity structure. We show that the ANNs can alleviate the "curse of dimensionality" under this circumstance. We establish the root-n consistency and asymptotic normality of the proposed general treatment effects estimators, and apply a weighted bootstrap procedure for conducting inference. The proposed methods are illustrated via simulation studies and a real data application.
更多查看译文
关键词
Artificial neural networks,Barron space,Mixed smoothness class,ReLU,Diverging confounders,Propensity score,Quantile treatment effects,Weighted bootstrap
AI 理解论文
溯源树
样例
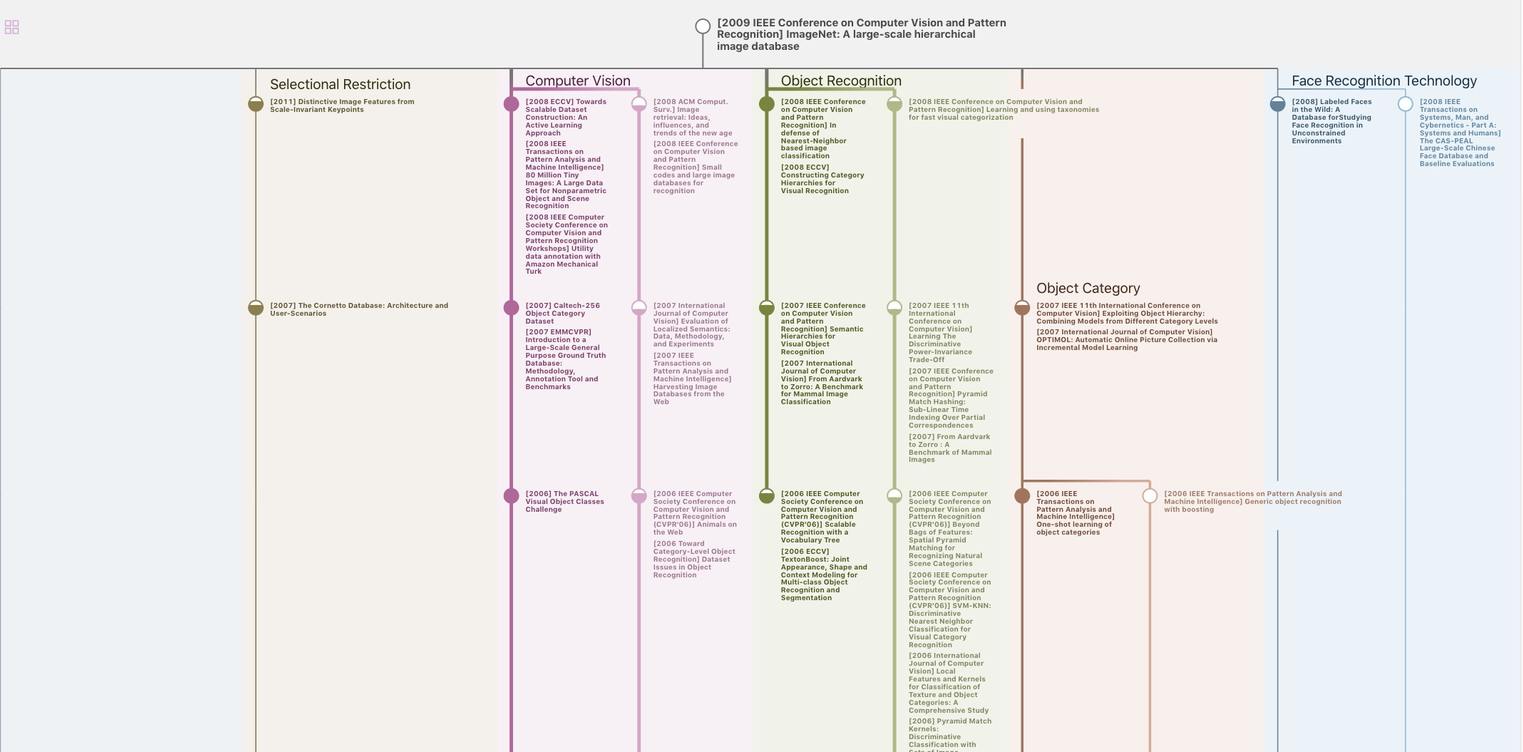
生成溯源树,研究论文发展脉络
Chat Paper
正在生成论文摘要