Leveraging Deep Reinforcement Learning for Water Distribution Systems with Large Action Spaces and Uncertainties: DRL-EPANET for Pressure Control
JOURNAL OF WATER RESOURCES PLANNING AND MANAGEMENT(2024)
摘要
Deep reinforcement learning (DRL) has undergone a revolution in recent years, enabling researchers to tackle a variety of previously inaccessible sequential decision problems. However, its application to the control of water distribution systems (WDS) remains limited. This research demonstrates the successful application of DRL for pressure control in WDS by simulating an environment using EPANET version 2.2, a popular open-source hydraulic simulator. We highlight the ability of DRL-EPANET to handle large action spaces, with more than 1 million possible actions in each time step, and its capacity to deal with uncertainties such as random pipe breaks. We employ the Branching Dueling Q-Network (BDQ) algorithm, which can learn in this context, and enhance it with an algorithmic modification called BDQ with fixed actions (BDQF) that achieves better rewards, especially when manipulated actions are sparse. The proposed methodology was validated using the hydraulic models of 10 real WDS, one of which integrated transmission and distribution systems operated by Hidralia, and the rest of which were operated by Aigues de Barcelona. This research presents the DRL-EPANET framework, which combines deep reinforcement learning and EPANET to optimize water distribution systems. Although the focus of this paper is on pressure control, the approach is highly versatile and can be applied to various sequential decision-making problems within WDS, such as pump optimization, energy management, and water quality control. DRL-EPANET was tested and proven effective on 10 real-world WDS, resulting in as much as 26% improvement in mean pressure compared with the reference solutions. The framework offers real-time control solutions, enabling water utility operators to react quickly to changes in the network. Additionally, it is capable of handling stochastic scenarios, such as random pipe bursts, demand uncertainty, contamination, and component failures, making it a valuable tool for managing complex and unpredictable situations. This method can be developed more with the use of model-based deep reinforcement learning for enhanced sample efficiency, graph neural networks for better representation, and the quantification of agent action uncertainty for improved decision-making in uncharted situations. Overall, DRL-EPANET has the potential to revolutionize the management and operation of water distribution systems, leading to more-efficient use of resources and improved service for consumers.
更多查看译文
AI 理解论文
溯源树
样例
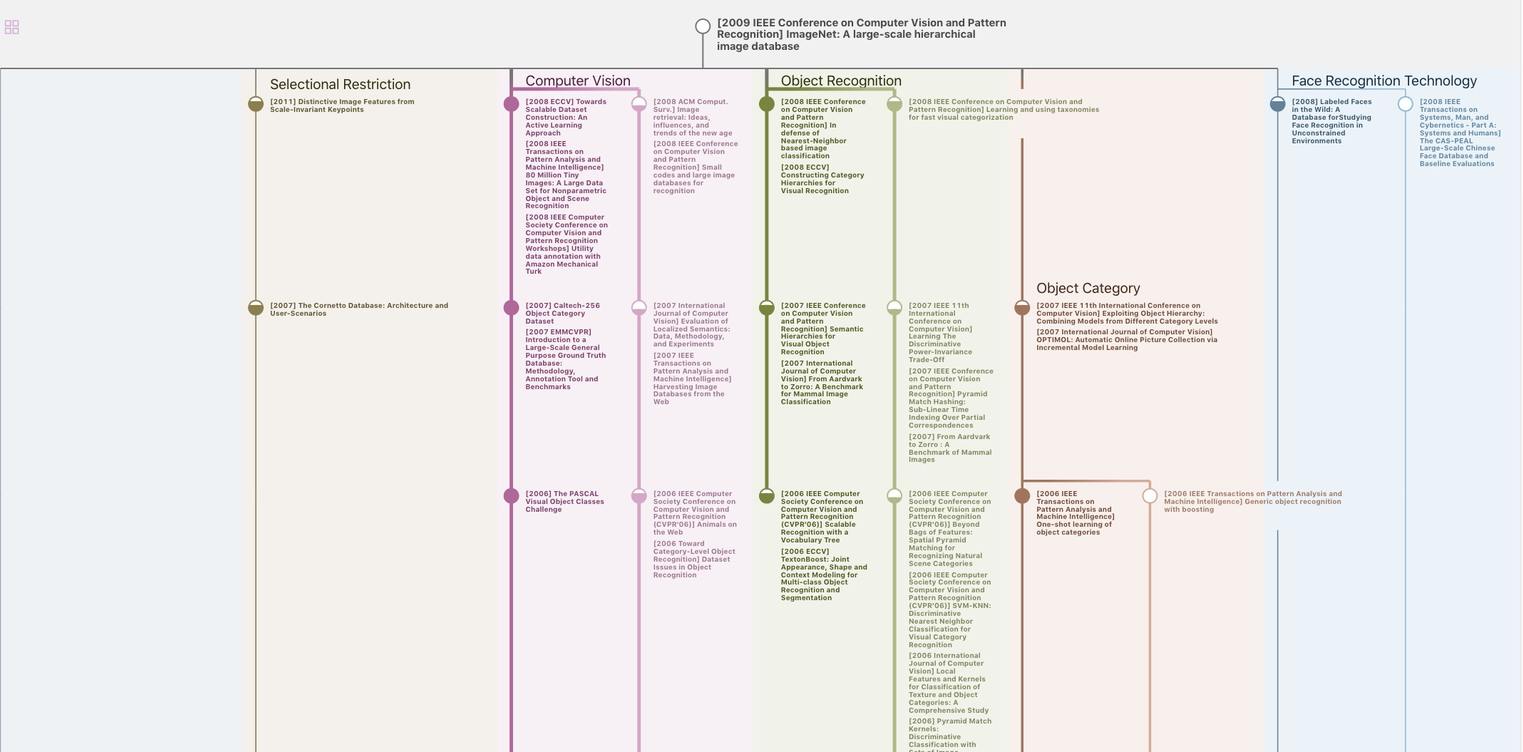
生成溯源树,研究论文发展脉络
Chat Paper
正在生成论文摘要