A Q-learning-based multi-objective evolutionary algorithm for integrated green production and distribution scheduling problems
ENGINEERING APPLICATIONS OF ARTIFICIAL INTELLIGENCE(2024)
摘要
In recent years, integrated production and distribution scheduling (IPDS) has received growing attention from academia and industry. With increasingly global economic activities, it is vital to extend IPDS to distributed environments. Besides, the production and distribution systems are the main contributors to carbon emissions due to their high energy consumption. Driven by this, this work introduces an integrated green distributed production and distribution scheduling problem with the objectives of total tardiness and total carbon emissions minimization. To provide a clear definition, a mixed-integer mathematical programming model is created. Considering the problem's NP-hard nature, a Q-learning-based multi-objective evolutionary algorithm (Q-MEA) is designed to handle it. To evaluate the performance of Q-MEA, a comparison pool including four variants, five meta-heuristics, and an exact optimizer named CPLEX is constructed to implement numerical experiments. The results demonstrate that Q-MEA has clear advantages over its rivals in tackling our problems. Furthermore, comparing the integrated model with a separate one shows that the former can achieve an average decrease of about 13.10% in total tardiness and 21.02% in total carbon emissions.
更多查看译文
关键词
Integrated scheduling of production and distribution,Distributed flow shop,Vehicle routing,Green scheduling,Meta-heuristic,Q-learning
AI 理解论文
溯源树
样例
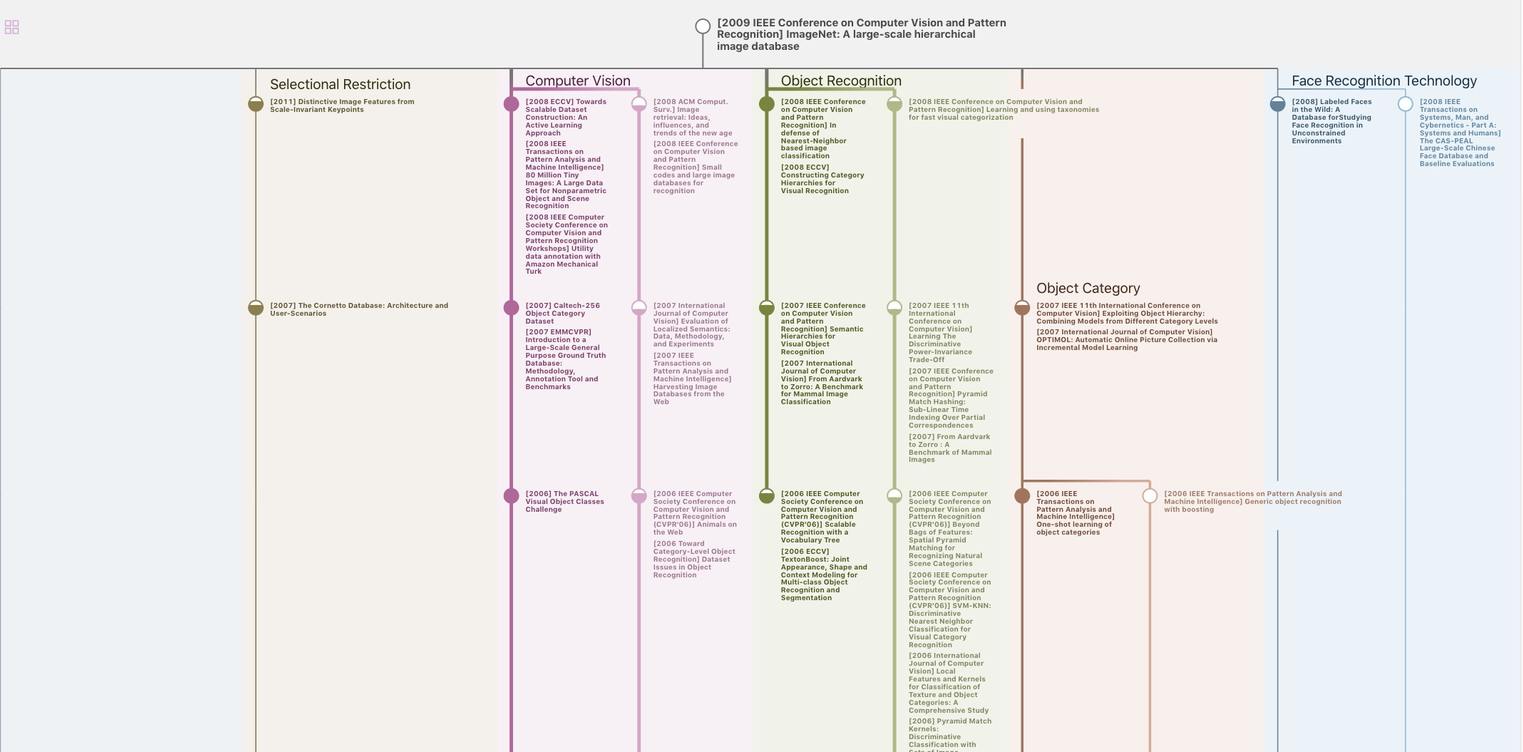
生成溯源树,研究论文发展脉络
Chat Paper
正在生成论文摘要