Greedy variable selection for high-dimensional cox models
STATISTICA SINICA(2023)
摘要
We examine the problem of variable selection for high-dimensional sparse Cox models. We propose using a computationally efficient procedure, the Cheby-shev greedy algorithm (CGA), to sequentially include variables, and derive its con-vergence rate under a weak sparsity condition. When we assume a strong sparsity condition, we use a high-dimensional information criterion (HDIC) and the CGA to achieve variable selection consistency. We further devise a greedier version of the CGA (gCGA). With the help of the HDIC, the gCGA not only enjoys selec-tion consistency, but also exhibits superior finite-sample performance in detecting marginally weak, but jointly strong signals over that of the original CGA and other related high-dimensional methods, such as conditional sure independence screen-ing. We demonstrate the proposed methods using real data from a cytogenetically normal acute myeloid leukaemia (CN-AML) data set.
更多查看译文
关键词
Chebyshev greedy algorithm,high-dimensional information criterion,sure screening,variable selection consistency
AI 理解论文
溯源树
样例
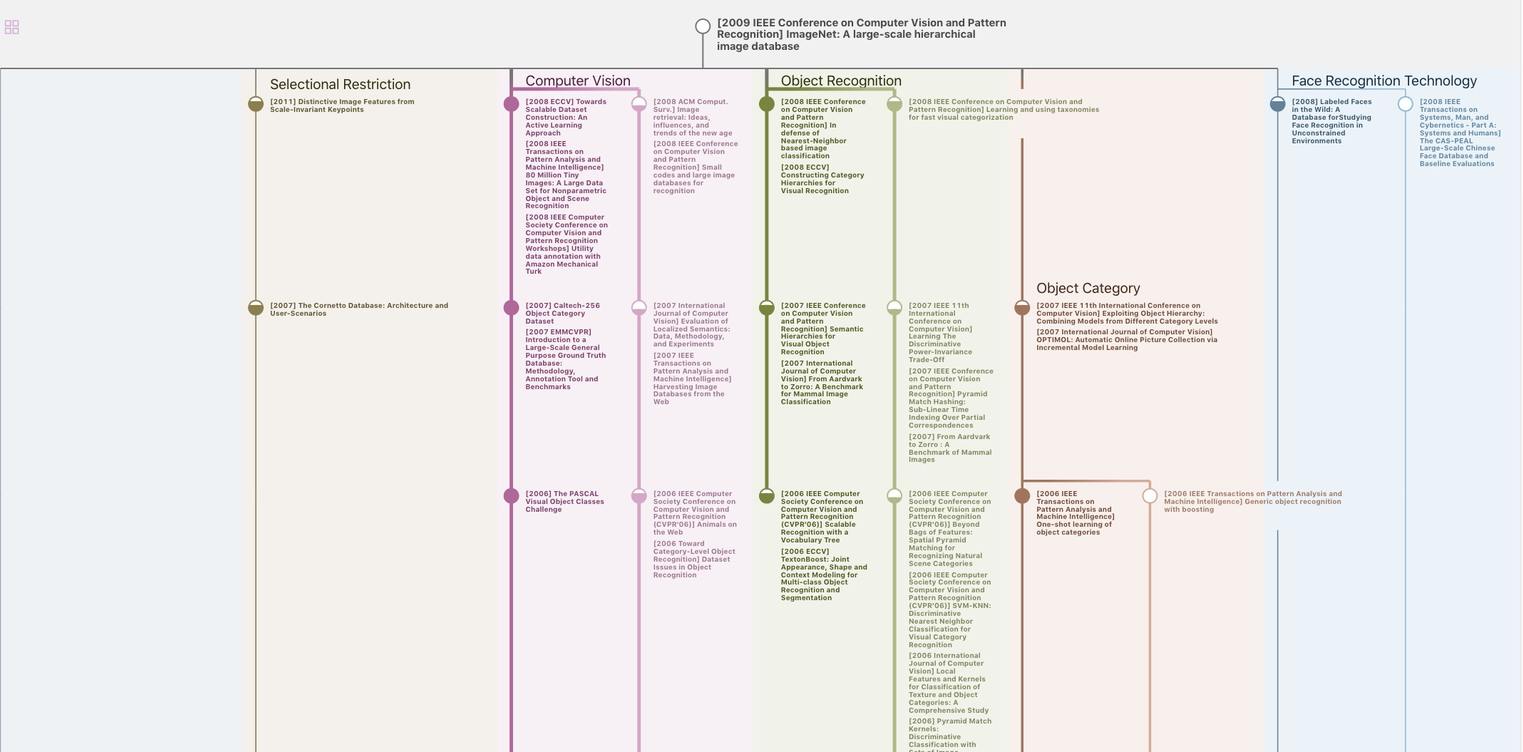
生成溯源树,研究论文发展脉络
Chat Paper
正在生成论文摘要