Enhanced Context Mining and Filtering for Learned Video Compression
IEEE TRANSACTIONS ON MULTIMEDIA(2024)
摘要
The Deep Contextual Video Compression framework (DCVC) utilizes a conditional coding paradigm, where the context is extracted and employed as a condition for the contextual encoder-decoder and entropy model. In this paper, we propose enhanced context mining and filtering to improve the compression efficiency of DCVC. Firstly, considering the context of DCVC is generated without supervision and redundancy may exist among context channels, an enhanced context mining model is proposed to mitigate redundancy across context channels to obtain superior context features. Then, we introduce a transformer-based enhancement network as a filtering module to capture long-distance dependencies and further enhance compression efficiency. The transformer-based enhancement adopts a full-resolution pipeline and calculates self-attention across channel dimensions. By combining the local modeling ability of the enhanced context mining model and the non-local modeling ability of the transformer-based enhancement network, our model outperforms LDP configurations of Versatile Video Coding (VVC), achieving an average bit savings of 6.7% in terms of MS-SSIM.
更多查看译文
关键词
Video compression,Context modeling,Transformers,Image coding,Entropy,Filtering,Codes,Learned video compression,end-to-end training approach,enhanced context mining,in loop filtering
AI 理解论文
溯源树
样例
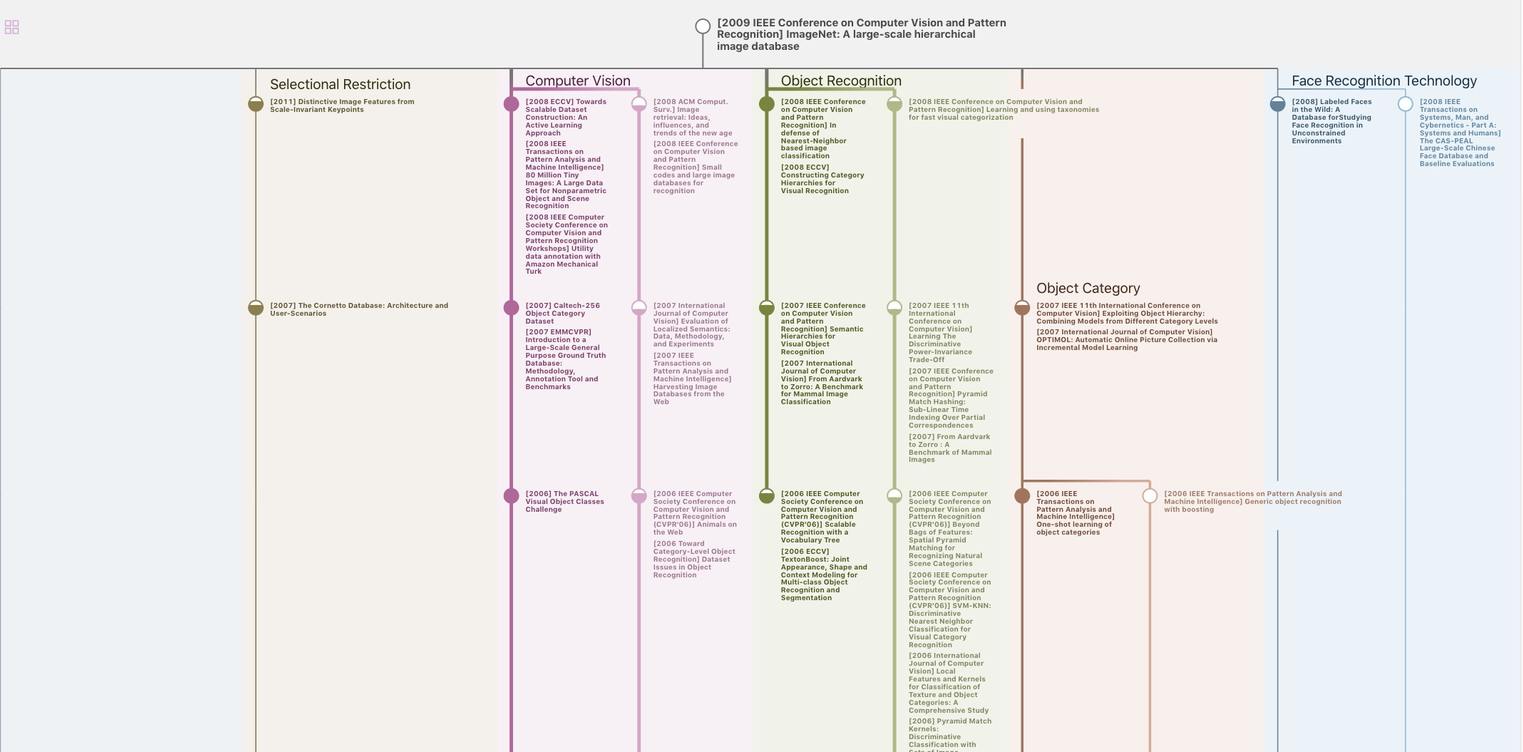
生成溯源树,研究论文发展脉络
Chat Paper
正在生成论文摘要