Investigating the Sample Weighting Mechanism Using an Interpretable Weighting Framework
IEEE TRANSACTIONS ON KNOWLEDGE AND DATA ENGINEERING(2024)
摘要
Training deep learning models with unequal sample weights has been shown to enhance model performance in various typical learning scenarios, particularly for imbalanced and noisy-label learning scenarios. A deep understanding of the weighting mechanism facilitates the application of existing weighting strategies and illuminates the design of new weighting strategies for real learning tasks. Scholars have focused on exploring existing weighting methods. However, their studies mainly establish how the weights of samples influence the model training. Little headway is made on the weighting mechanism, i.e., which and how the characteristics of a sample influence its weight. In this study, we adopt a data-driven approach to investigate the weighting mechanism by utilizing an interpretable weighting framework. First, a wide range of sample characteristics is extracted from the classifier network during training. Second, the extracted characteristics are fed into a new neural regression tree (NRT), which is a tree model implemented by a neural network, and its output is the weight of the input sample. Third, the NRT is trained using meta-learning within the whole training process. Once the NRT is learned, the weighting mechanism, including the importance of weighting characteristics, prior modes, and specific weighting rules, can be obtained. We conduct extensive experiments on benchmark noisy and imbalanced data corpora. A package of weighting mechanisms is derived from the learned NRT. Furthermore, our proposed interpretable weighting framework exhibits superior performance in comparison to existing weighting strategies.
更多查看译文
关键词
Sample weighting,interpretability,neural regression tree,meta-learning
AI 理解论文
溯源树
样例
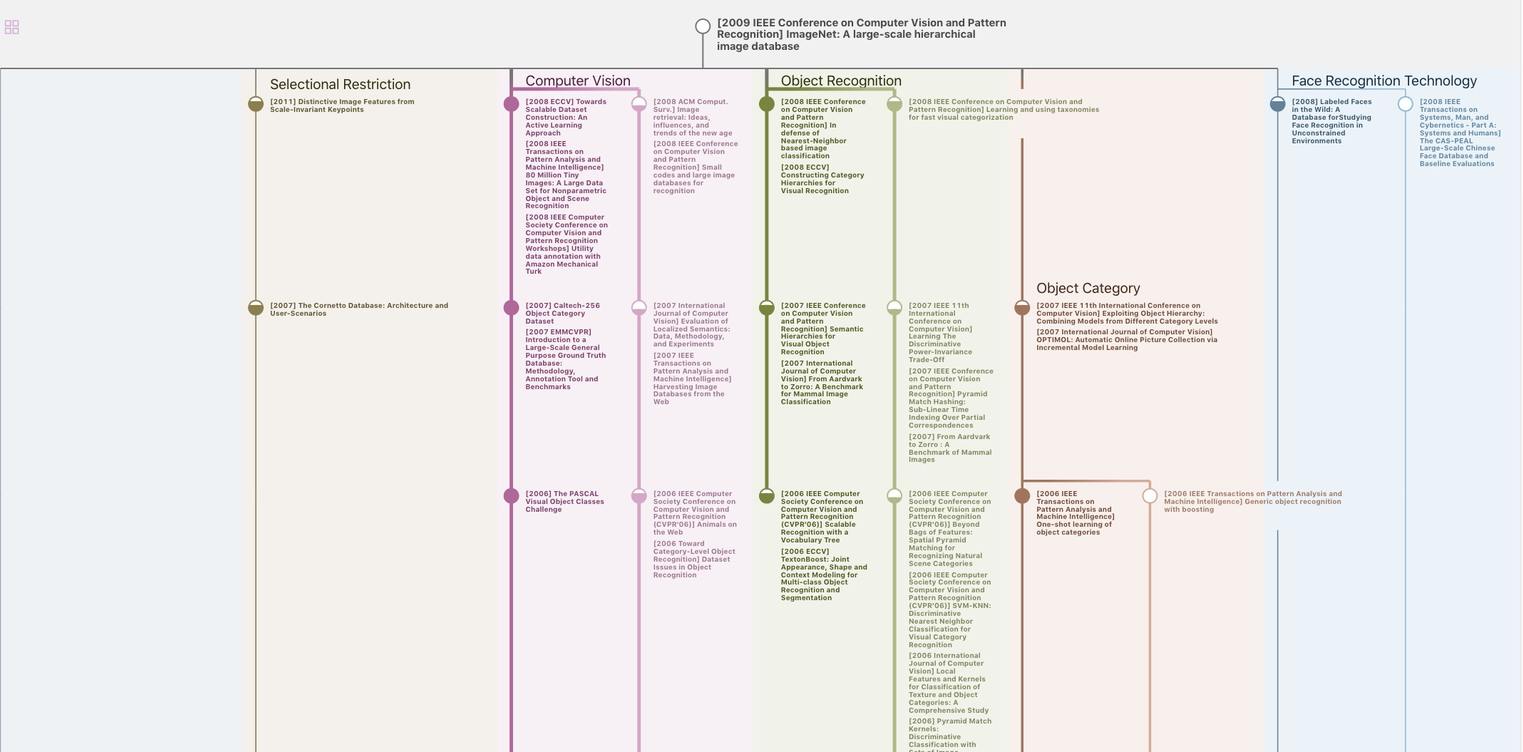
生成溯源树,研究论文发展脉络
Chat Paper
正在生成论文摘要