An Empirical Study of Intrusion Detection by Combining Clustering and Classification Methods
Communications in computer and information science(2023)
摘要
Intrusion detection applications are becoming more critical, and the need for more advanced intrusion detection systems is increasing. Many existing machine learning and artificial intelligence applications are used for this task. Nevertheless, these models were claimed to operate better when the data was clustered before the classification process. In this work, we study the effect of clustering datasets related to attacks applied in different network environments on the classification results generated by many famous and repeatedly used machine learning models. Two different clustering methods were analyzed in this work combined with nine machine learning models; After two hundred and forty-three experiments, it has been concluded that using DBSCAN clustering before classification can slightly enhance the overall model performance. Nonetheless, the enhancement did not exceed 1% in the field of intrusion detection systems.
更多查看译文
关键词
intrusion detection,clustering,classification,empirical study
AI 理解论文
溯源树
样例
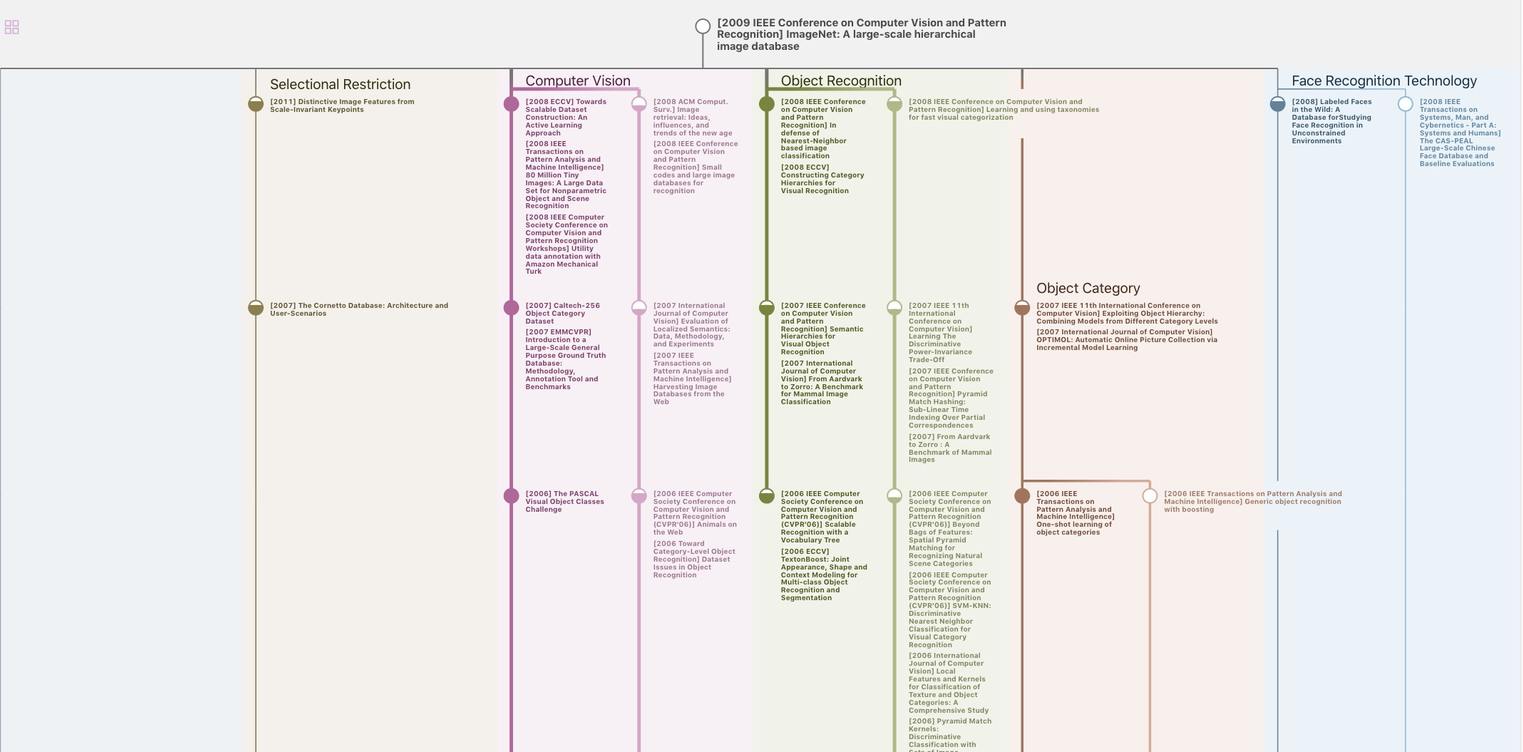
生成溯源树,研究论文发展脉络
Chat Paper
正在生成论文摘要