An Efficient and Distributed Framework for Real-Time Trajectory Stream Clustering
IEEE TRANSACTIONS ON KNOWLEDGE AND DATA ENGINEERING(2024)
摘要
With the explosive ubiquity of GPS-equipped devices, e.g., mobile phones, vehicles, and vessels, a massive amount of real-time, unbounded, and varying-sampling trajectory streams are being generated continuously. Clustering trajectory streams is useful in real-life applications, such as traffic congestion prediction, crowd flow detection, and moving behavior study. Although several sliding-window based algorithms (that adopt the classic two-phases online-offline processing framework) are proposed for trajectory stream clustering, three challenges exist to meet ever-increasing application demands for effective, efficient, and scalable online clustering: i) How to effectively model unbounded trajectory streams in the online settings for effective clustering? ii) How to achieve truly real-time online processing? iii) How to improve the scalable capability of the clustering algorithm to support large-scale moving trajectory streams? In this paper, we propose an efficient and distributed trajectory stream clustering framework that can: i) model trajectory streams dynamically and effectively in a self-adaptive manner, i.e., k-Segment, which considers both spatial and temporal aspects of trajectory streams, ii) support distributed indexing, processing, and workload balance, and iii) incrementally cluster trajectory streams in an efficient manner. Experiments on a wide range of real-world trajectory datasets show that our framework outperforms state-of-the-art baselines in terms of clustering quality, efficiency, and scalability.
更多查看译文
关键词
Trajectory,Real-time systems,Clustering algorithms,Market research,Scalability,Behavioral sciences,Measurement,DBSCAN,distributed online processing,grid partitioning,trajectory stream clustering
AI 理解论文
溯源树
样例
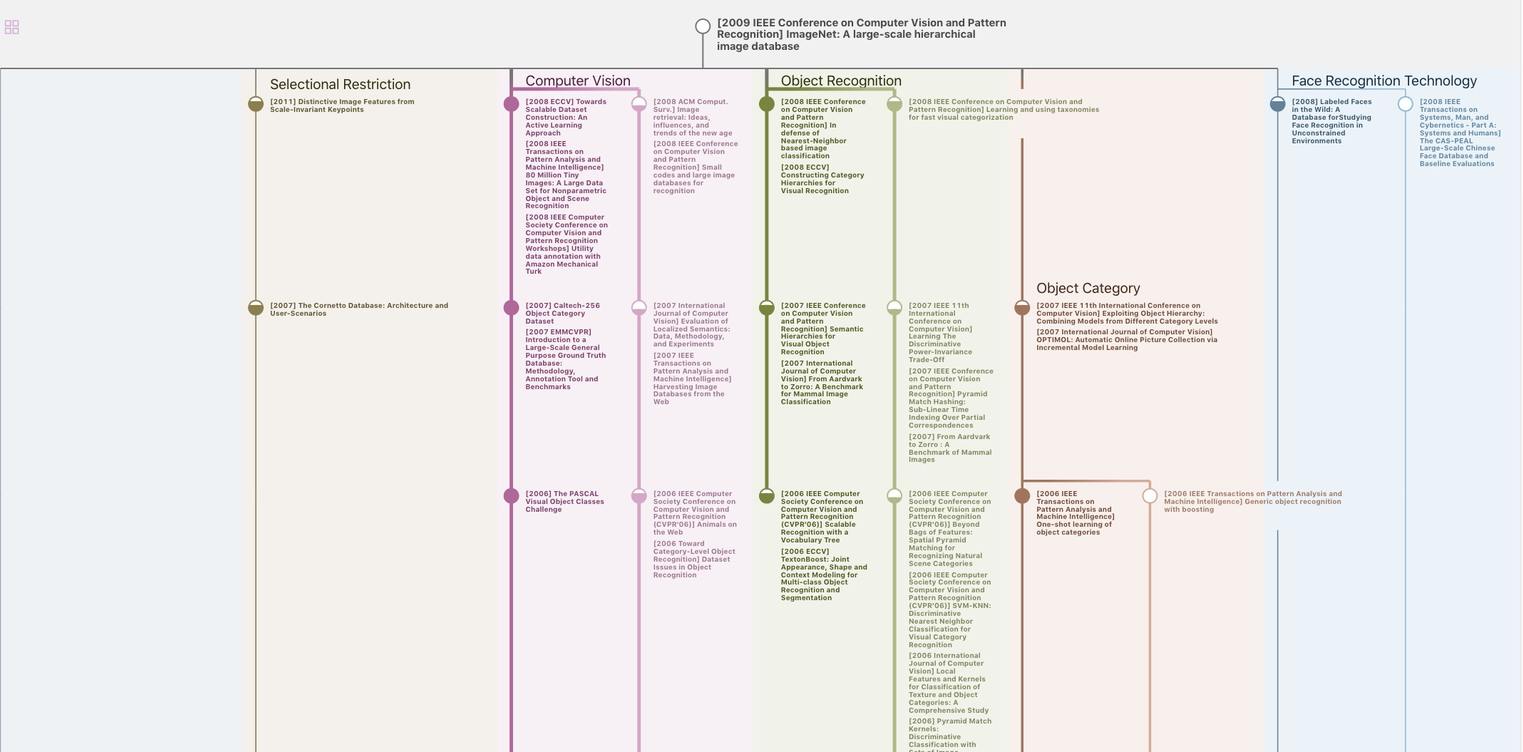
生成溯源树,研究论文发展脉络
Chat Paper
正在生成论文摘要