Automated region of interest placement on cervical ultrasound images for assessing preterm birth risk
Journal of the Acoustical Society of America(2023)
摘要
Spontaneous preterm birth (sPTB) is one of the leading causes of infant morbidity. Medical interventions can prevent death caused by preterm birth if the risk is predicted at early stages. Quantitative ultrasound (QUS) is found valuable for predicting sPTB risk with a limitation of requiring a medically trained image analyst to manually draw a region of interest (ROI) on the cervix of a B-mode ultrasound image. An automated ROI placement algorithm was designed and trained to reduce the reliance on human annotations. The algorithm utilized a deep neural network with an optimized U-Net architecture to locate cervical tissues. A total of 8670 ultrasound images with sonographer-drawn ROIs were used for algorithm training and testing, followed by several postprocessing steps to yield the final ROIs. Quantitative comparison between algorithm-generated and sonographer-drawn ROIs yielded an average pixel accuracy of 96% and a dice coefficient of 88%. In addition, the QUS’s attenuation coefficient (AC) and backscatter coefficient (BSC) obtained from the algorithm-generated ROIs were highly correlated to those obtained from the sonographer-drawn ROIs with a Pearson correlation coefficient of 0.93 and 0.85, respectively. The results support the feasibility of automating QUS imaging for sPTB risk assessment. [Work supported by NIHR01HD089935.]
更多查看译文
关键词
cervical ultrasound images,preterm birth risk,interest placement
AI 理解论文
溯源树
样例
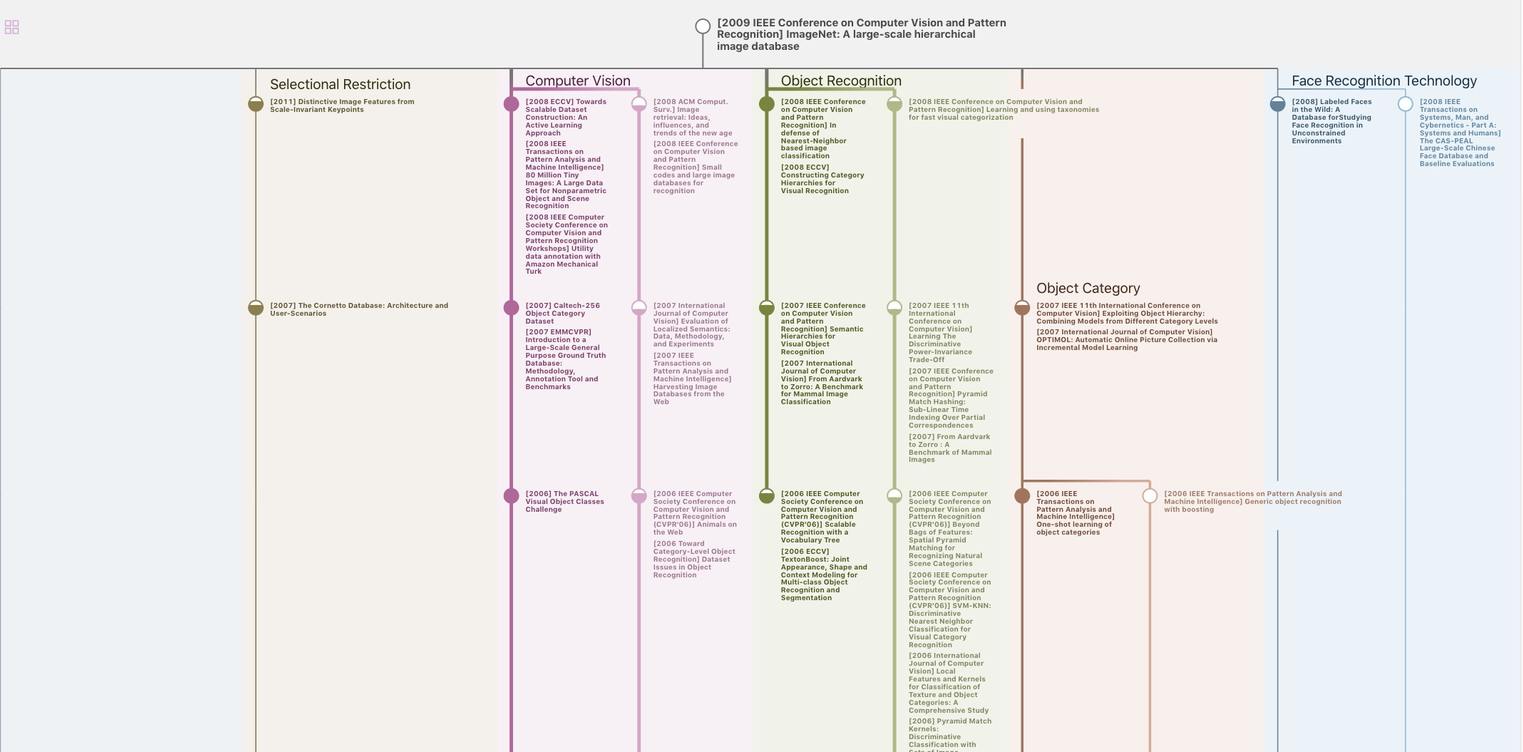
生成溯源树,研究论文发展脉络
Chat Paper
正在生成论文摘要