Endmembers compression based nonnegative matrix factorization for hyperspectral unmixing
INTERNATIONAL JOURNAL OF REMOTE SENSING(2023)
摘要
Hyperspectral unmixing (HU) has grown more important in hyperspectral images (HSIs) research in recent years. Many models based on nonnegative matrix factorization (NMF) and nonnegative tensor factorization (NTF) have been extensively used to tackle the HU problem. Most of these models allow a large and extensive exploration of abundance and provide promising unmixing results. However, the exploration of each endmember information is insufficient. In this article, we propose an unmixing model called endmembers compressionbased NMF (EC-NMF) for HU. Specifically, by an orthogonal projection, the EC-NMF enables distinct endmembers to be differentiated from each other. In this space, each compressed endmember represents the distinct characteristics of the corresponding endmember, which makes it easier for the endmembers separated. Furthermore, the EC-NMF model incorporates the reweighted nuclear norm on the abundance map to fully account for spatial information and to constrain its low-rank properties. The EC-NMF model is solved by an augmented multiplicative algorithm. In particular, we achieve the proposed model based on the matrix representation, which reduces the computational complexity compared with NTF-based methods. Extensive experiments on synthetic and real data give concrete evidence that the proposed method outperforms other known methods in both the unmixing performance and running time.
更多查看译文
关键词
hyperspectral unmixing,endmembers compression,nonnegative matrix factorization,hyperspectral images
AI 理解论文
溯源树
样例
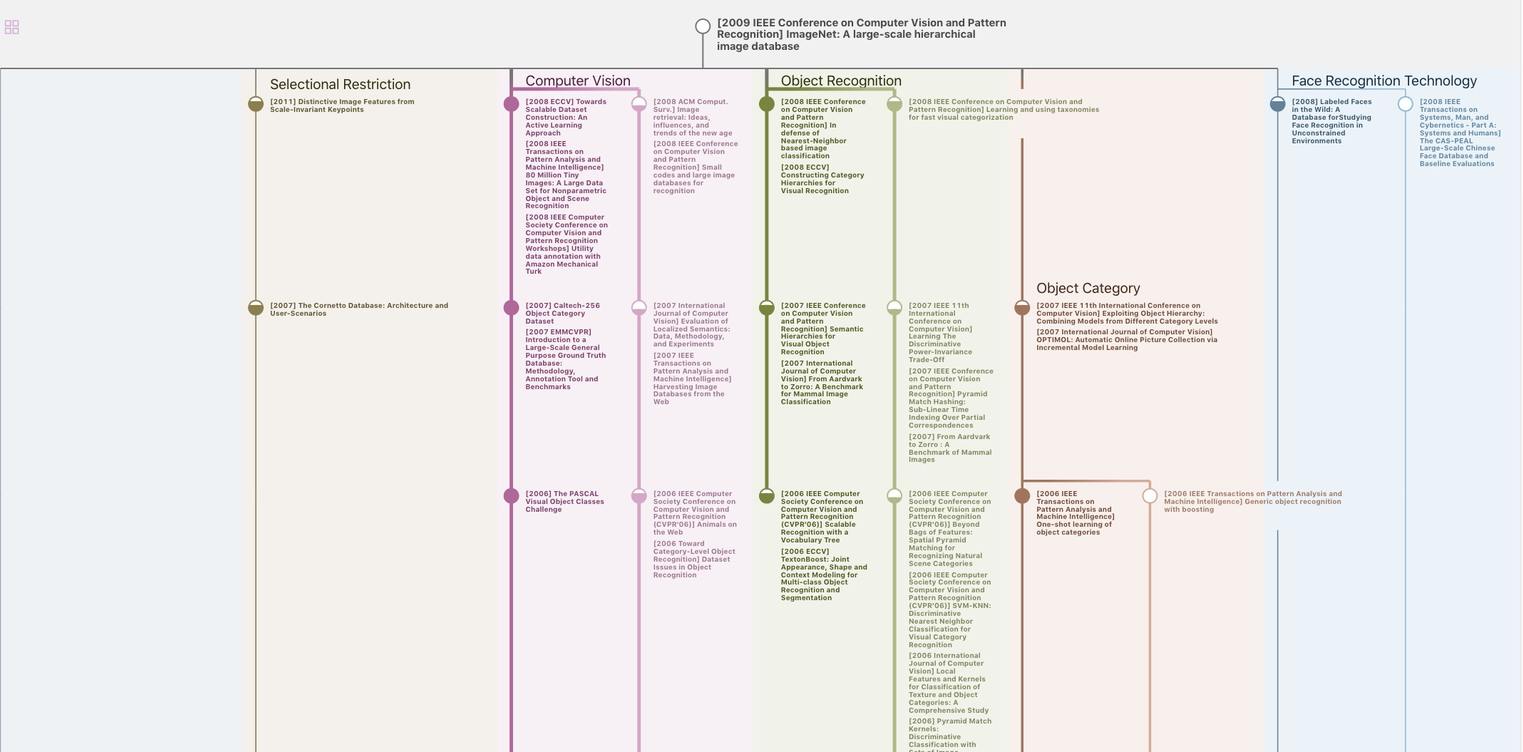
生成溯源树,研究论文发展脉络
Chat Paper
正在生成论文摘要