Seismic data denoising through dual attention residual network
Research Square (Research Square)(2023)
摘要
Signal-to-noise ratio enhancement is conducive to seismic data imaging and interpretation. Denoising is the main way to solve this problem. In recent years, deep learning has made some achievements in data denoising. However, many methods based on deep learning do not consider the characteristics of seismic data, resulting in the loss of weak signals. This paper proposes dual attention residual convolutional neural network (DARN) for seismic data denoising. It consists of a number of dual attention residual blocks (DARBs). The main components of DARB are channel attention residual block (CARB) and spatial attention residual block (SARB). CARB is designed to learn the characteristics of random noise in different channels. It can preserves and transmits characteristic information about noise from different channels. SARB is designed to learn the distributional of random noise. It generates a spatial attention map of noise by combining different pooling methods and recalibrates its input feature map. Loss function with residual learning converts the learning target into noise to improve the training efficiency of the network. Randomized leaky rectified linear unit can retain negative information adaptively and efficiently. The well-trained network is applied to synthetic data and field data to verify its effectiveness. Comparison with other methods demonstrated that DARN can effectively suppress random noise and retain weak effective signal.
更多查看译文
关键词
seismic data,dual attention
AI 理解论文
溯源树
样例
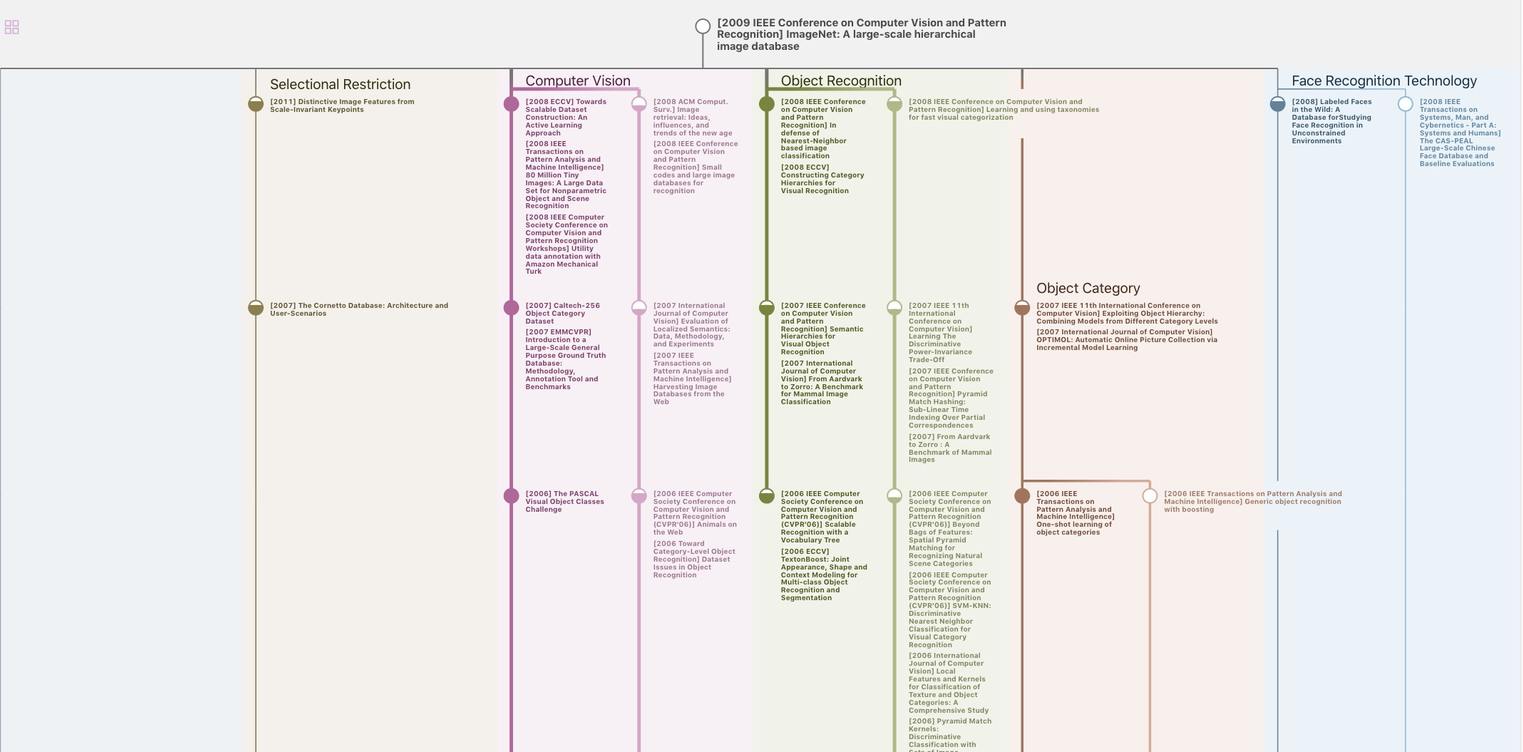
生成溯源树,研究论文发展脉络
Chat Paper
正在生成论文摘要