A novel approach to learning through categorical variables applicable to the classification of solitary pulmonary nodule malignancy
Research Square (Research Square)(2023)
摘要
Abstract Background One of the main drawbacks in constructing a classification model is that some or all of the covariates are categorical variables. Classical methods either assign labels to each output of a categorical variable or are summarised measures (frequencies and percentages), which can be interpreted as probabilities. Methods We adopted a novel mathematical procedure to construct a classification model from categorical variables based on a non-classical probability approach. More specifically, we codified the variables following the categorical data representation from the Discriminant Correspondence Analysis before constructing a non-classical probability matrix system that represents an entangled system of dependent-independent variables. We then developed a disentangled procedure to obtain an empirical density function for each representative class (minimum of two classes). Finally, we constructed our classification model using the density functions. Results We applied the proposed procedure to build a classification model of the malignancy of Solitary Pulmonary Nodule (SPN) after five years of follow up using routine clinical data. First, with 2/3 (270) of the sample of 404 patients with SPN, we constructed the classification model, and then validated it with the remaining 1/3 (134) we validated it. We tested the procedure’s stability by repeating the analysis randomly 1000 times. We obtained a model accuracy of 0.74, an F1 score of 0.58, a Cohen’s Kappa value of 0.41 and a Matthews Correlation Coefficient of 0.45. Finally, the area under the ROC curve was 0.86. Conclusion The proposed procedure provides a machine learning classification model with an acceptable performance of a classification model of solitary pulmonary nodule malignancy constructed from routine clinical data and mainly composed of categorical variables. It provides an acceptable performance, which could be used by clinicians as a tool to classify SPN malignancy in routine clinical practice.
更多查看译文
关键词
classification,malignancy,categorical variables
AI 理解论文
溯源树
样例
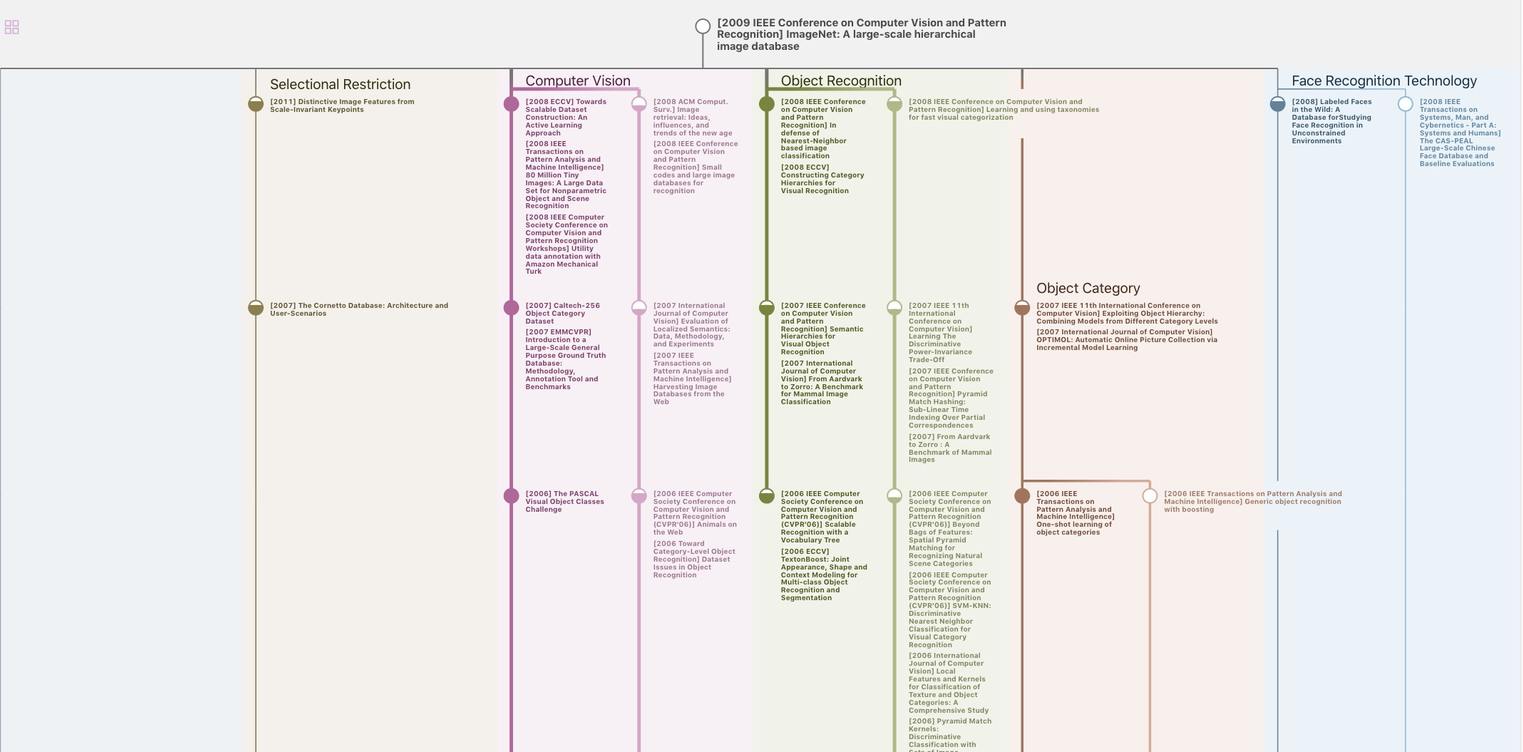
生成溯源树,研究论文发展脉络
Chat Paper
正在生成论文摘要