Computing Over the Sky: Joint UAV Trajectory and Task Offloading Scheme Based on Optimization-Embedding Multi-Agent Deep Reinforcement Learning
IEEE TRANSACTIONS ON COMMUNICATIONS(2024)
摘要
Unmanned aerial vehicle (UAV)-assisted mobile edge computing (MEC) has emerged to support computation-intensive tasks in 6G systems. Since the battery capacity of a UAV is limited, to serve as many users as possible, a joint design on UAV trajectory and offloading strategy with consideration for service fairness is essential to provide energy-efficient computation offloading to the users in UAV-MEC networks. Unfortunately, such a joint decision-making problem is not straightforward due to various task types required from users and various functionalities of different UAVs enabled by different application programs. Considering the above issues, we take energy efficiency and service fairness as the objective, and propose a Multi-Agent Energy-Efficient joint Trajectory and Computation Offloading (MA-ETCO) scheme. To adapt to dynamic demands of users, we develop an optimization-embedding multi-agent deep reinforcement learning (OMADRL) algorithm. Each UAV autonomously learns the trajectory control decision based on MADRL to adapt to dynamic demands. Then, it will obtain the optimal computation offloading decision by solving a mixed-integer nonlinear programming problem. The computation offloading result, in turn, will be used as an indicator to guide UAVs' trajectory design. Compared to relying solely on deep reinforcement learning, such an optimization-embedding way reduces action space dimension and improves convergence efficiency.
更多查看译文
关键词
Autonomous aerial vehicles,Task analysis,Trajectory,Heuristic algorithms,Delays,Reinforcement learning,Resource management,Unmanned aerial vehicle,mobile edge computing,computation offloading,trajectory control,reinforcement learning
AI 理解论文
溯源树
样例
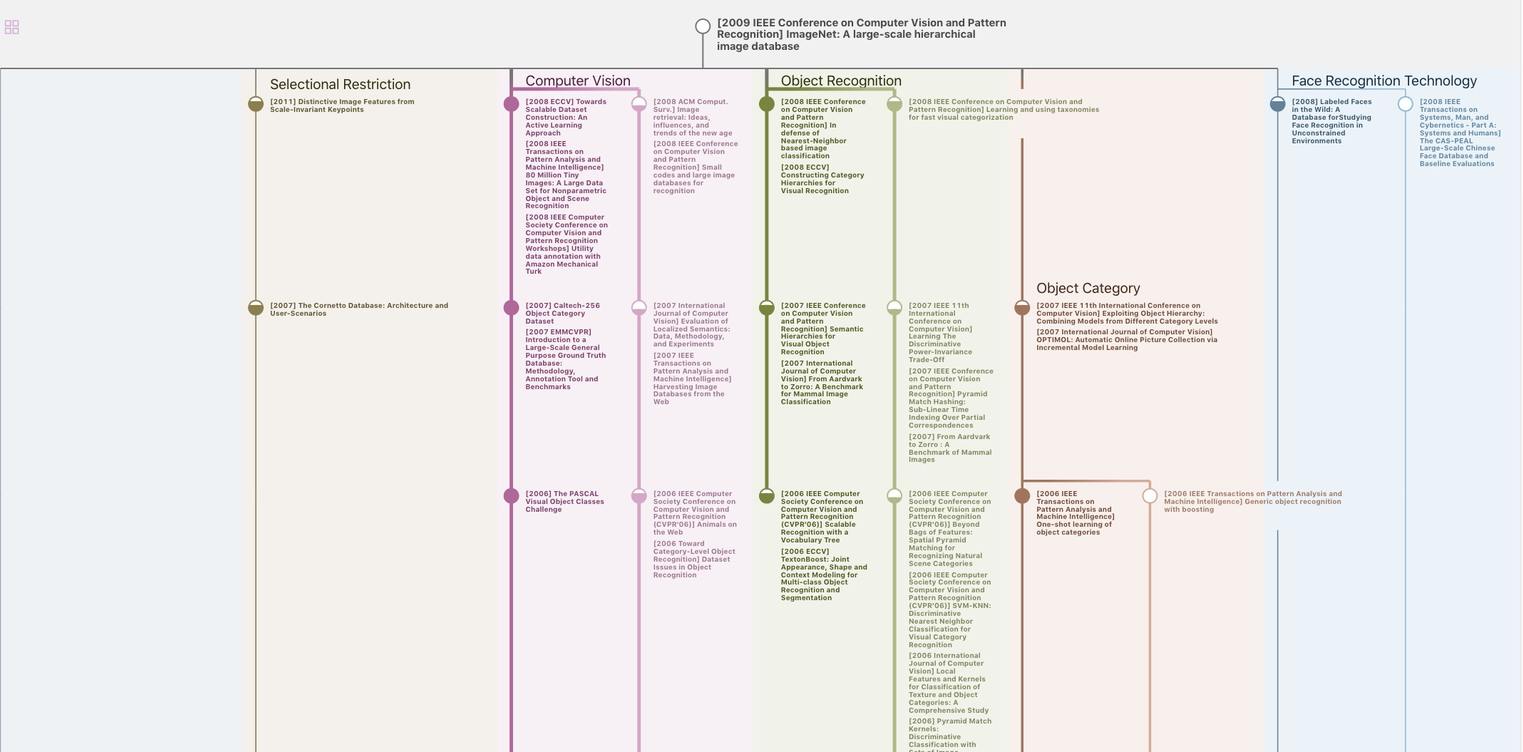
生成溯源树,研究论文发展脉络
Chat Paper
正在生成论文摘要