Graph Neural Networks for Quality of Service Improvement in Interference-Limited Ultra-Reliable and Low-Latency Communications
IEEE TRANSACTIONS ON VEHICULAR TECHNOLOGY(2024)
Abstract
This paper aims to improve the Quality-of-Service (QoS) of Ultra-Reliability and Low-Latency Communications (URLLC) in interference-limited wireless networks. To obtain time diversity within the channel coherence time, we first put forward a random repetition scheme that randomizes the interference power. Then, we optimize the number of reserved slots and the number of repetitions for each packet to minimize the QoS violation probability, defined as the percentage of users that cannot achieve URLLC. We build a cascaded Random Edge Graph Neural Network (REGNN) to represent the repetition scheme and develop a model-free unsupervised learning method to train it. To obtain some analytical results, we derive approximations of the QoS violation probability using stochastic geometry in a Poisson bipolar network and apply a model-based Exhaustive Search (ES) method to find the optimal repetition scheme. Based on the analytical results, a model-assisted initialization is developed for the cascaded REGNN to improve the training efficiency. Simulation results show that the cascaded REGNN can achieve a lower QoS violation probability than the cascaded fully connected neural network, and generalizes well in wireless networks with different scales, network topologies, cell densities, and frequency reuse factors.
MoreTranslated text
Key words
Ultra-reliable and low-latency communications,graph neural network,quality-of-service,interference-limited wireless networks,stochastic geometry
AI Read Science
Must-Reading Tree
Example
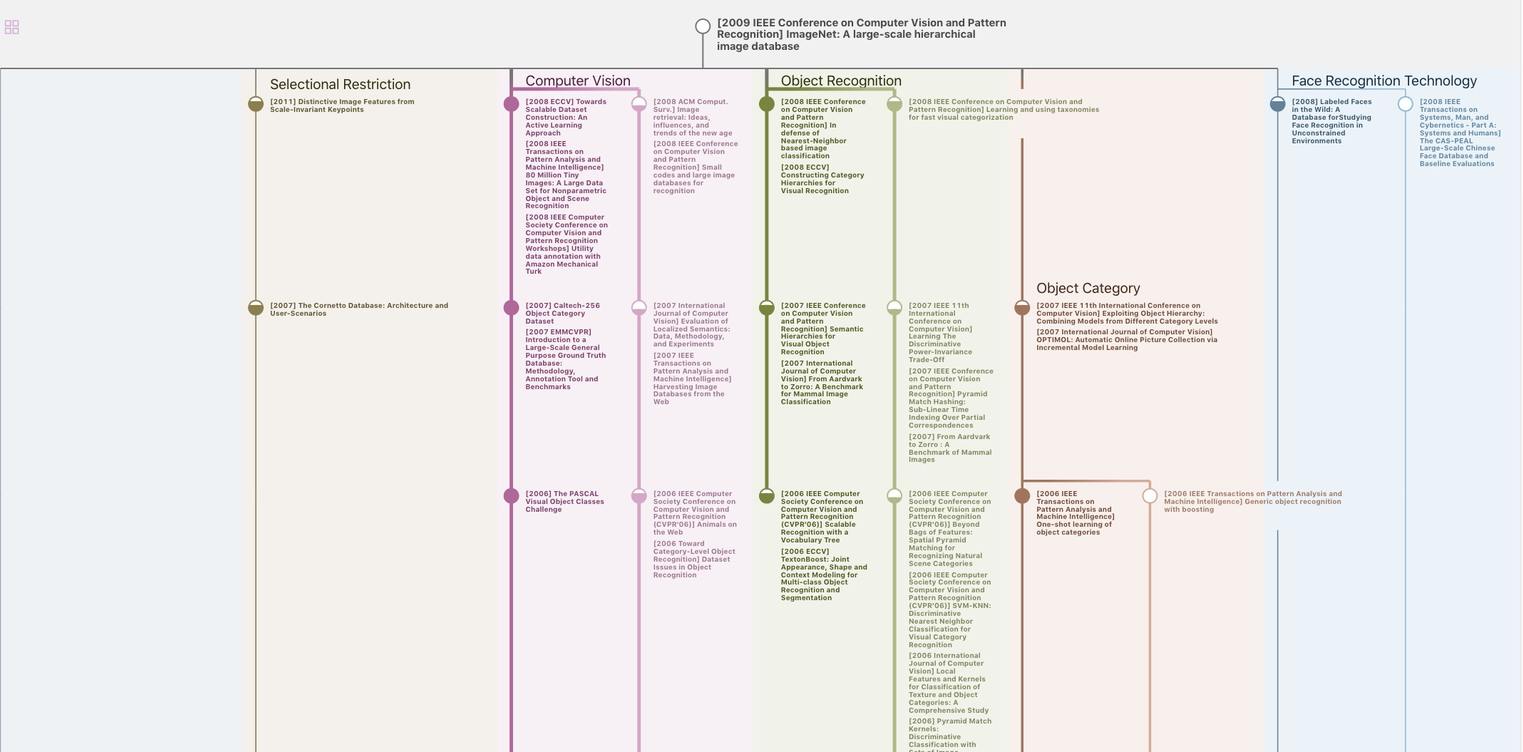
Generate MRT to find the research sequence of this paper
Chat Paper
Summary is being generated by the instructions you defined