Deep Learning Parameterization of Vertical Wind Velocity Variability via Constrained Adversarial Training
Artificial Intelligence for the Earth Systems(2023)
Abstract
Abstract Atmospheric models with typical resolution in the tenths of kilometers cannot resolve the dynamics of air parcel ascent, which varies on scales ranging from tens to hundreds of meters. Small-scale wind fluctuations are thus characterized by a subgrid distribution of vertical wind velocity, W , with standard deviation σ W . The parameterization of σ W is fundamental to the representation of aerosol-cloud interactions, yet it is poorly constrained. Using a novel deep learning technique, this work develops a new parameterization for σ W merging data from global storm-resolving model simulations, high-frequency retrievals of W , and climate reanalysis products. The parameterization reproduces the observed statistics of σ W and leverages learned physical relations from the model simulations to guide extrapolation beyond the observed domain. Incorporating observational data during the training phase was found to be critical for its performance. The parameterization can be applied online within large-scale atmospheric models, or offline using output from weather forecasting and reanalysis products.
MoreTranslated text
Key words
vertical wind velocity variability
AI Read Science
Must-Reading Tree
Example
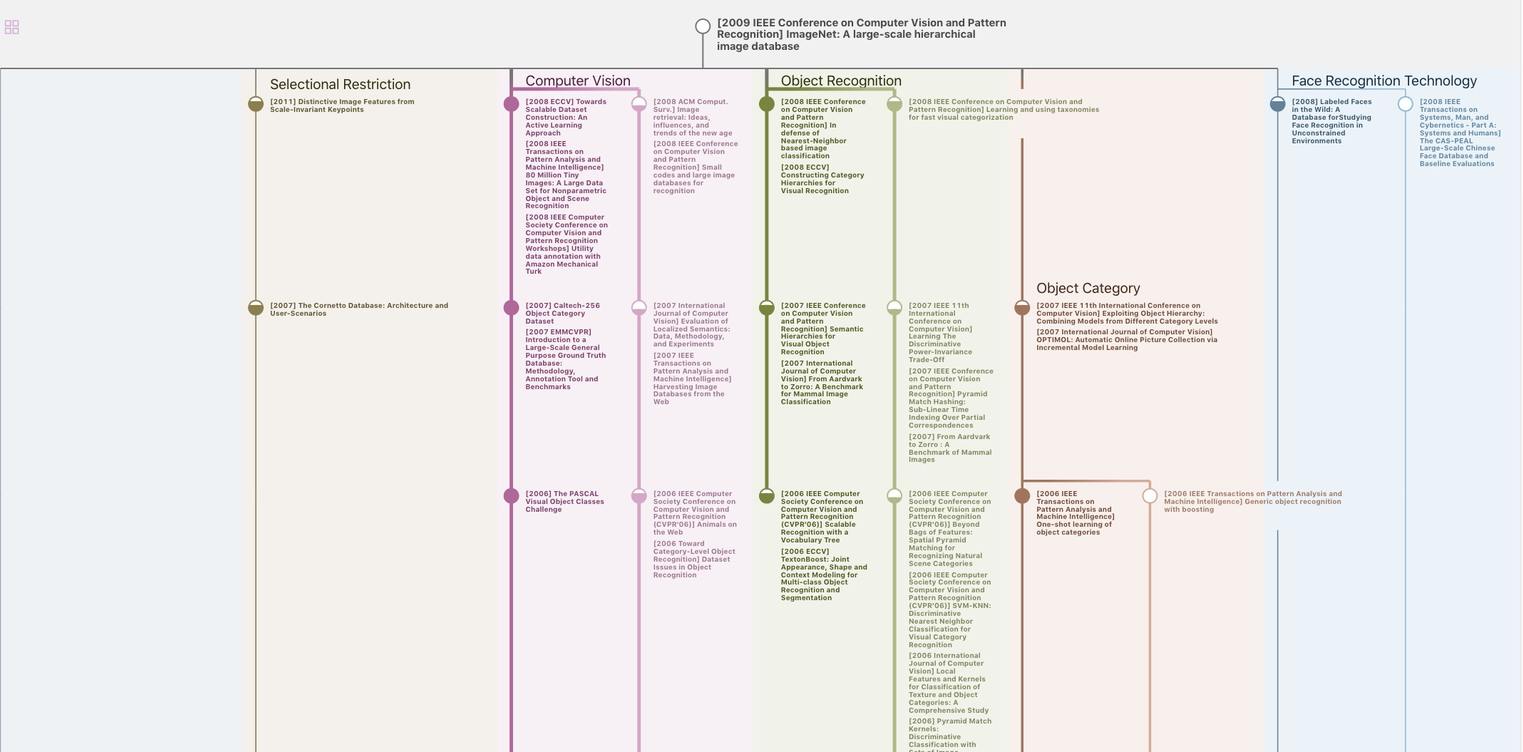
Generate MRT to find the research sequence of this paper
Chat Paper
Summary is being generated by the instructions you defined