Optimized Material Removal and Tool Wear Rates in Milling API 5ST TS-90 Alloy: AI-Driven Optimization and Modelling with ANN, ANFIS, and RSM
Qeios(2023)
摘要
One of the performance measures and responses in manufacturing is the optimization of the Material Removal Rate (MRR) and Tool Wear Rate (TWR). This study used the response surface method (RSM) and AI-based models of artificial neural networks (ANNs) and adaptive neuro-fuzzy inference systems (ANFISs) to model and optimize the MRR and TWR for milling API 5ST TS-90 alloys. A ZX6350C milling machine was used to conduct twenty (20) experimental runs using a 10 mm HSS end-mill cutter. The experiment was designed using Central Composite Design (CCD) in Design Expert 14 software. RSM, ANN, and ANFIS models were applied in the predictive modelling of the milling process with a coefficient of determination above 0.85. Comparatively, ANFIS and RSM were marginally better than ANN in the predicted MRR for the milling process. The ANFIS and RSM were slightly better than ANN in the predicted TWR for the milling operation. Generally, ANFIS showed a better predictive capability than RSM and ANN for both MRR and TWR. The optimum process milling parameters for the alloy were a 720-rpm spindle speed, 24 mm/min feed rate and 0.979 mm depth of cut to achieve an optimized predicted MRR of 1272.163 mm3/min and TWR of 0.781 mm/min. The optimum milling process was validated at the suggested experimental conditions by carrying out the milling experiment at those conditions, and the results of 1270.05 mm3/min for MRR and 0.77 mm/min for TWR showed a close correlation between the predicted and validated optimum. The optimum milling parameters from this study would enhance production at reduced tooling costs associated with tool wear.
更多查看译文
关键词
tool wear rates,milling api,alloy,material removal,optimization,ai-driven
AI 理解论文
溯源树
样例
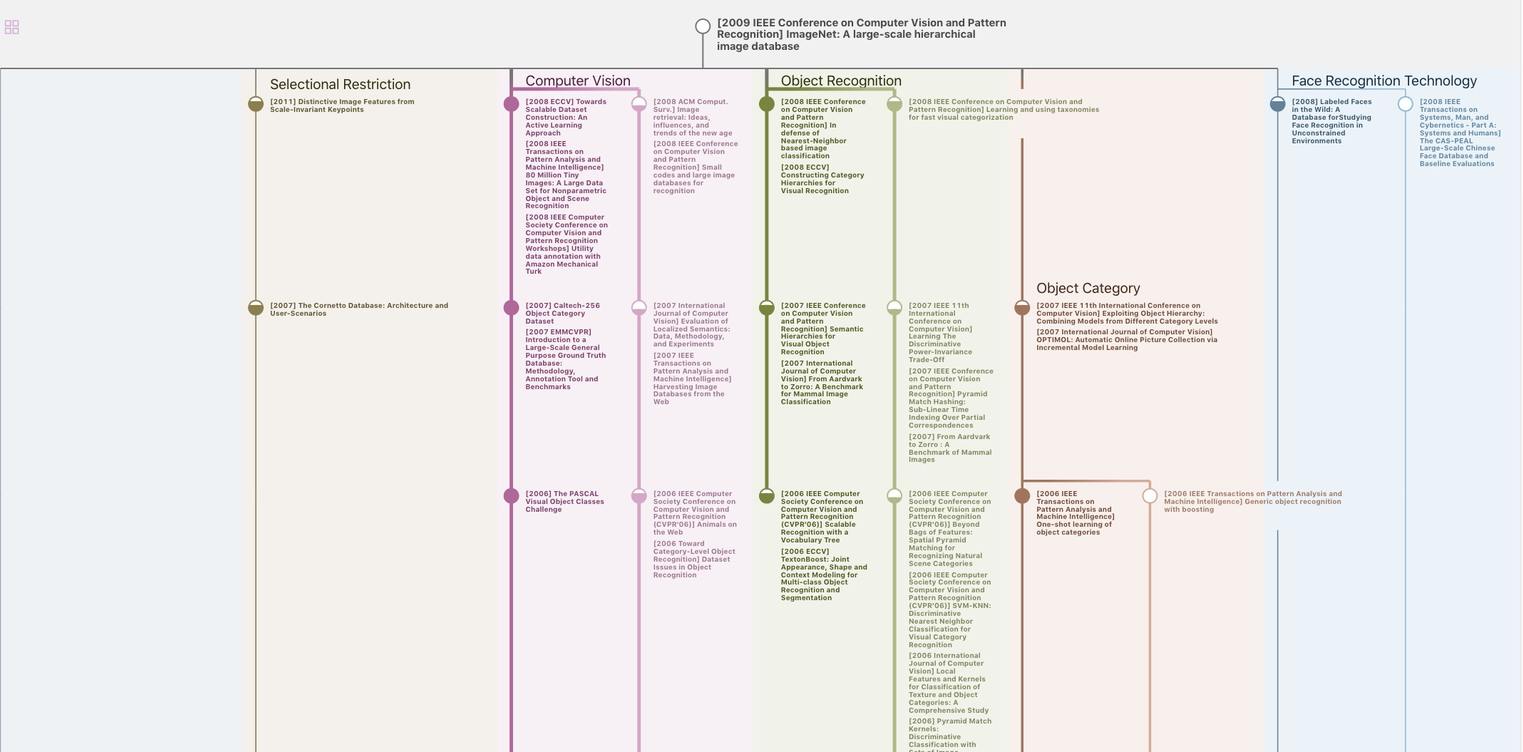
生成溯源树,研究论文发展脉络
Chat Paper
正在生成论文摘要