Ternary Data, Triangle Decoding, Three Tasks, a Multitask Learning Speech Translation Model
ARTIFICIAL NEURAL NETWORKS AND MACHINE LEARNING, ICANN 2023, PT III(2023)
摘要
Direct end-to-end approaches for speech translation (ST) are now competing with the traditional cascade solutions. However, end-to-end models still suffer from the challenge of ST data scarcity. How to effectively utilize the limited ST data or more text machine translation (MT) data is appealing but still an open problem. The end-to-end model requires the model to have both cross-modal and cross-language capabilities, which increases the mapping difficulty. In this paper, we propose a tightly tied multitask ST model. By adding a lightweight adapter, we make the ASR decoder also be the MT encoder, where they use one language model and share the source text semantic space. Thus, our model can utilize the MT data. Our end-to-end model can accomplish the ST, ASR and MT tasks simultaneously, and multitask learning can promote the overall performance of an ST model. Our method can make efficient and full use of the limited ternary ST data and even more intelligently utilize external data. When using the limited ternary data, our ST method can achieve state-of-the-art performance in end-to-end models. When adding the external data, our method shows a significant improvement on the strong baselines.
更多查看译文
关键词
Speech translation,speech,Machine translation,Cross-modal,Speech-to-text
AI 理解论文
溯源树
样例
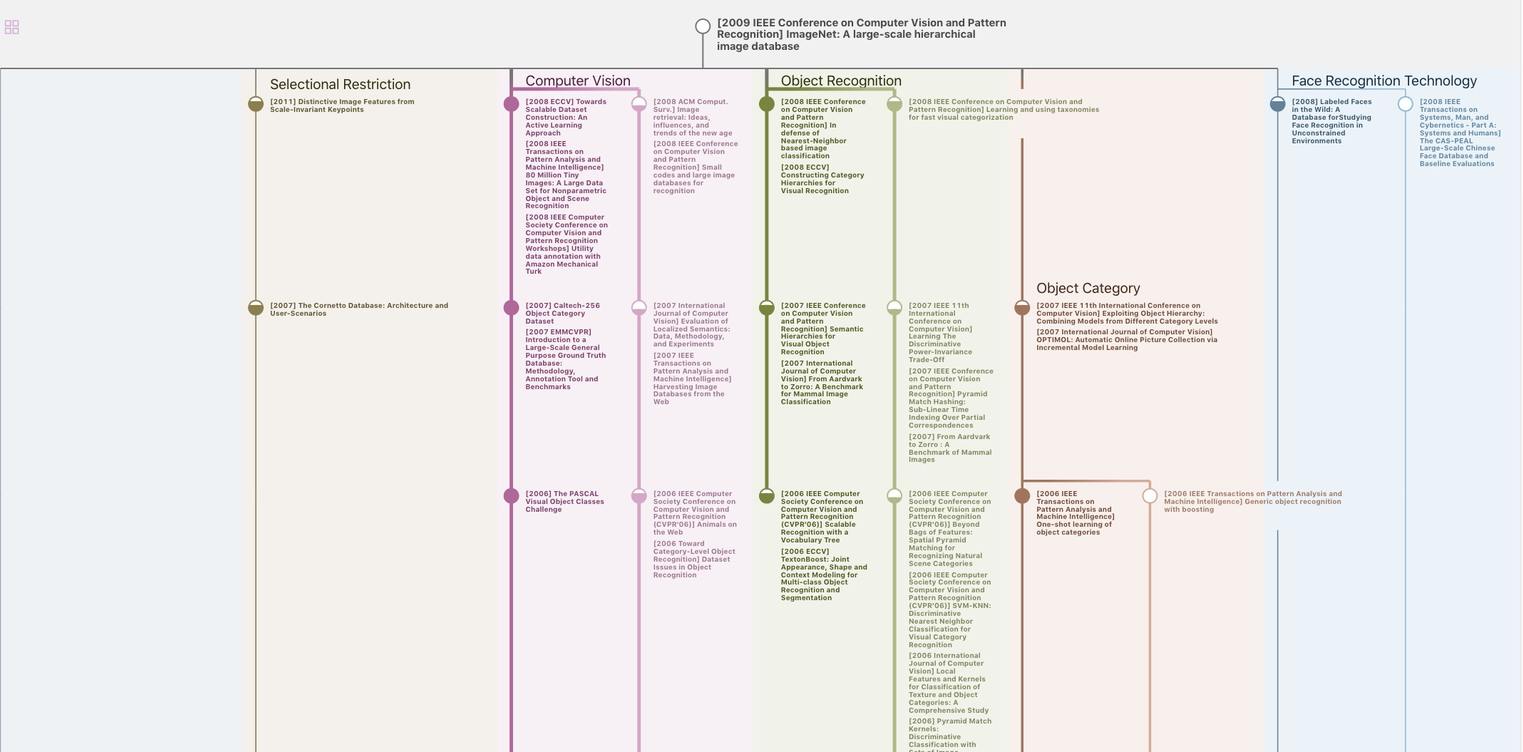
生成溯源树,研究论文发展脉络
Chat Paper
正在生成论文摘要