Reliability of an AI driven 3-dimensional markerless motion capture system for on the field sport applications
Gait & Posture(2023)
摘要
Motion capture technology is having an important impact on sports, on one side for optimizing athletes performance, on the other side for injuries prevention and recovery. Different options are available, from markerbased stereophotogrammetric systems, video based tracking technologies and markerless motion capture techniques [1,2]. Among these AI driven markerless motion captures have been recently introduced with the potential to surpass limitations associated with the requirement of wearing special equipment (i.e. inertial measurement units or markers), requiring sophisticated laboratories (i.e. stereophotogrammetry) or long processing time for tracking (video based tracking systems) [3]. Deep learning-based pose estimation involves detecting sets of learned features that describe subjects pose, and they are mostly trained using publicly available 3-dimensional (3D) human pose datasets [4]. The aim of this study was to assess the reliability and repeatability of a convolutional neural network based markerless motion capture system (CNN_markerless) trained with a proprietary dataset of 2D labels according to iorgait [1] markerset acquired in the wild while subjects were performing different tasks (i.e. gait, squat, drop, axel jump, side cut, landing). Ten healthy subjects (mean age and BMI were respectively 26.9±3.1 years, 22.5±2.7 kg/m^2) were simultaneously acquired through 8 GoProHero® cameras (v3-7, 30-240 Hz) and 6 BTS (60-120) cameras while performing 3 consecutive bilateral squats within the BiomovLab gait analysis laboratory (10×4.43 m). All subjects gave written informed consent to The protocol approved by the local Ethics Committee. Skin markers were placed according to the modified IOR Gait protocol [1]. A deep learning method for multi-view 3D pose estimation was adopted that takes as input 2D images (524 frames training and 222 frames validation dataset) and provides pose heat maps for all views. the CNN_markerless generates sequences of 3D anatomical landmarks trajectories [1] and embeds the same anatomical reference frames and joint angle definitions. Agreement between different methodologies was determined by computing the root mean square error (RMSE) and the coefficient of multiple correlation (CMC)[5] between corresponding joint angles estimated via stereophotogrammetry, TrackOnField (BBSoF S.r.l) and CNN_markerless. Table 1. CMC and RMSE computed among joint angles computed by means of anatomical landmark trajectories reconstructed via stereophotogrammetry, TrackOnField and CNN_markerless.Download : Download high-res image (203KB)Download : Download full-size image Results of the present study showed very good correlation between trials at the level of all joint rotations, and very low RMSE. Best RMSE were obtained on trunk obliquity, while the worst were recorded on knee flexion-extension. This will offer the possibiliy to perform a biomechanical analysis on the athletes in their training environment. Niccolò Monaco is the developer of the CNN_markerless system and Federica Cibin is the product specialist at BBSoF S.r.l (spinoff of the University of Padova).
更多查看译文
关键词
motion,ai
AI 理解论文
溯源树
样例
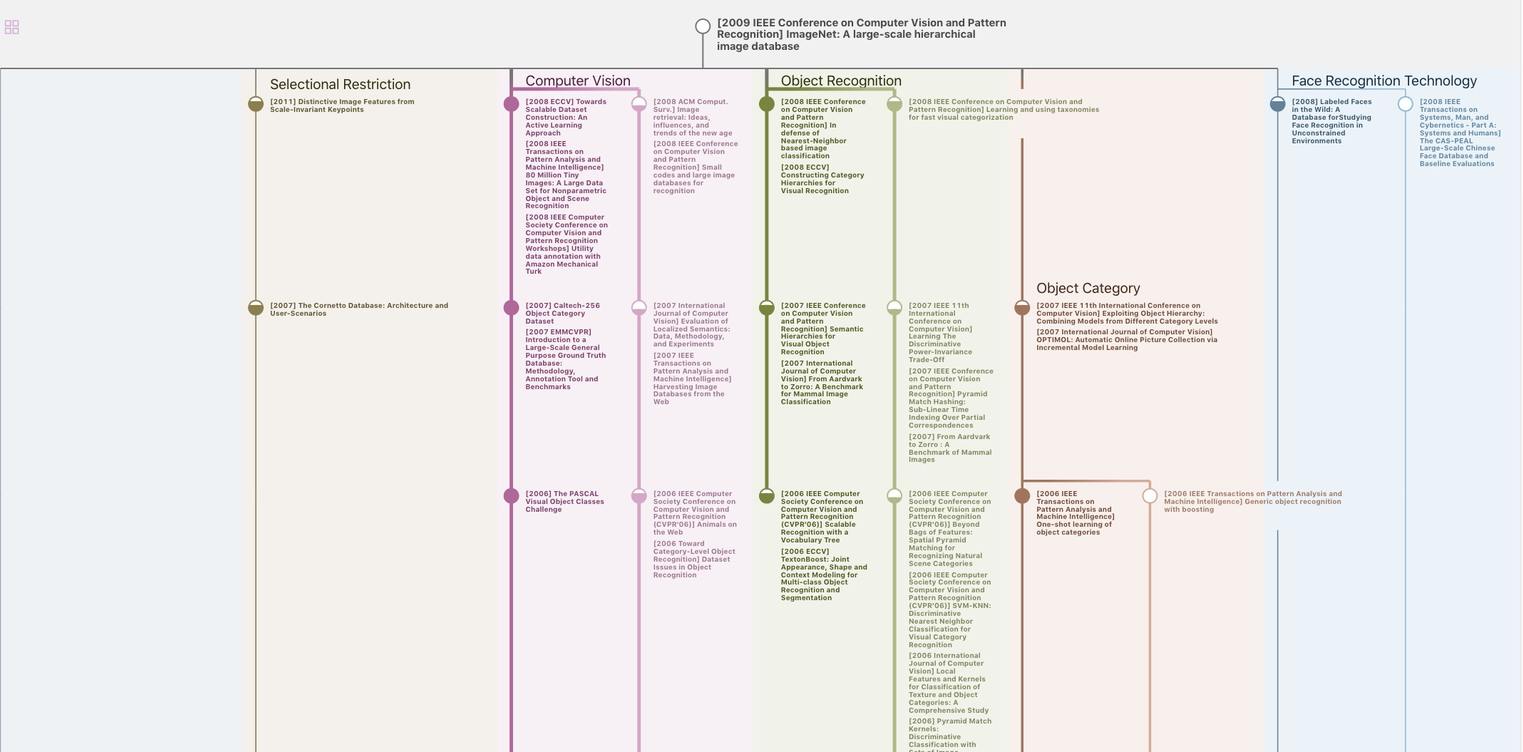
生成溯源树,研究论文发展脉络
Chat Paper
正在生成论文摘要