Drilling Stuck Probability Intelligent Prediction Based on LSTM Considering Local Interpretability
All Days(2023)
摘要
ABSTRACT Stuck pipe in the drilling can increase the non-productive time and lead to drilling risks such as buried tool and drilling tool fracture. Due to complex sequential feature analysis and the lag of downhole data transmission, traditional methods which are mostly used for post-drilling analyses have poor timeliness and low accuracy so that it cannot meet the requirements of field application. This study provides a data-driven prediction method for stuck pipe probability. First, the dataset is established combining drilling records and logging data from 108 wells, and 15 input features are selected. Then, the intelligent prediction model based on the Long Short Term Memory (LSTM) network is developed considering the timing characteristics of drilling parameters. Finally, Shapley Additive Explanations (SHAP) is used to calculate the quantitative contribution of input parameters to solve the problem of the black box. The results show that the accuracy of the LSTM model is 97%. Through local interpretation and analysis, the model accords with the natural physical law. The proposed model has great timeliness, high accuracy, high reliability and quantitative risk prediction. This study provides useful insights into predicting stuck pipe risk. INTRODUCTION Drilling is the only way to get oil and gas which is an uncertain undertaking. Due to poor underground conditions, complex conditions (lost circulation, well collapse, stuck pipe, etc.) often occur, among which complicated conditions of stuck pipe often occur in the drilling construction process. According to Chunbo Zhao (1998), stuck drilling accidents account for 40%∼50% of the total drilling complications in western oilfields, which seriously restricts safe, economical, and efficient drilling. Therefore, the timely prediction of stuck pipe events is considered a primary necessity to assist the drilling team in the decision-making process, so that appropriate countermeasures can be put into effect before the situation slips out of hand. Mudlog data shows that stuck pipe accidents occur in the process of tripping and drilling with varying degrees of severity. For example, in some highly deviated wells and horizontal wells, the stuck pipe accident is often accompanied by the process of tripping, but it is usually not serious. On the contrary, more attention is paid to the stuck pipe during drilling. Once the drill string is completely stuck, it can only be lifted by the explosion and a new side drilling scheme need to be redesigned, which seriously restricts the safety and efficiency of drilling. Therefore, we focus on the real-time prediction of stuck pipe in the process of drilling.
更多查看译文
关键词
lstm considering local interpretability,stuck probability intelligent prediction
AI 理解论文
溯源树
样例
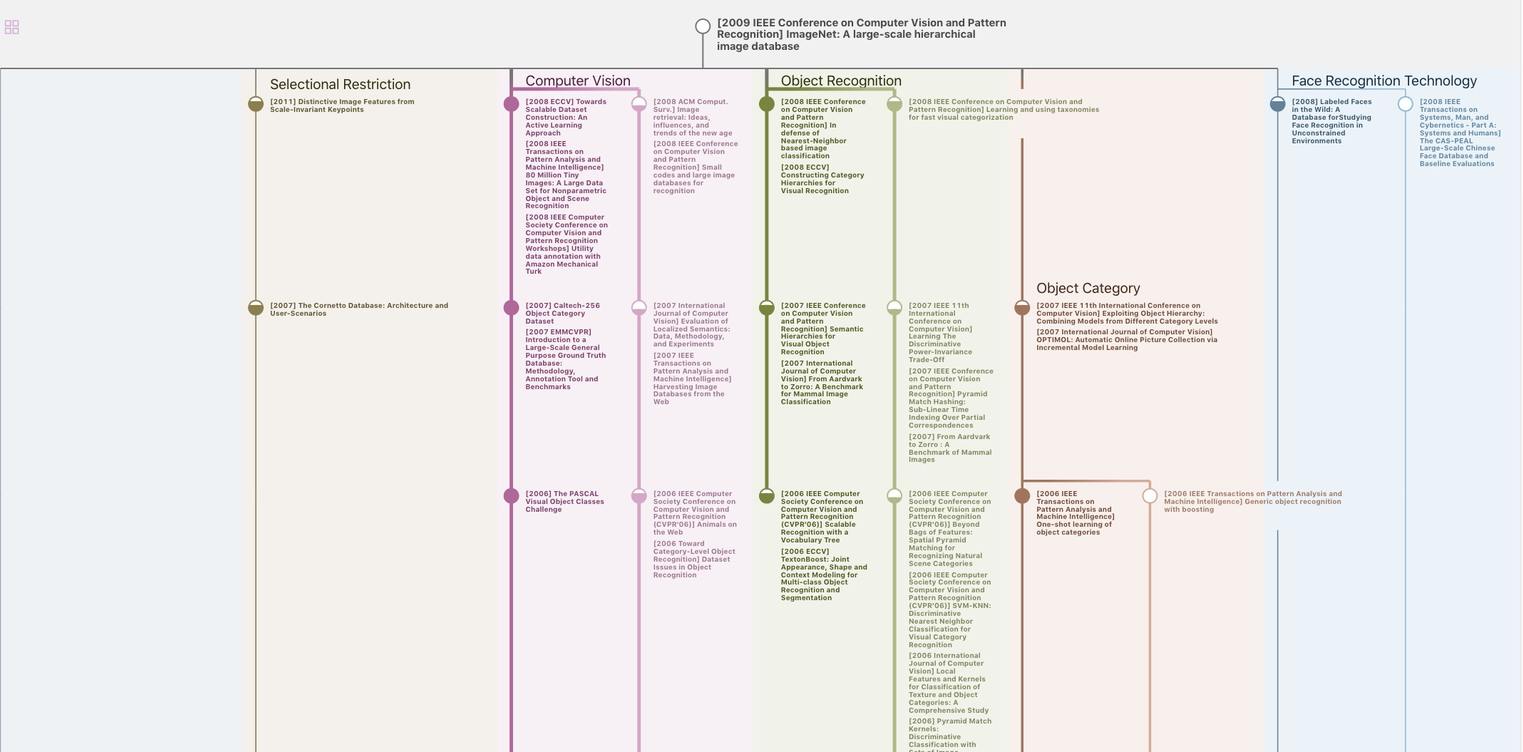
生成溯源树,研究论文发展脉络
Chat Paper
正在生成论文摘要