Application of machine learning methods to predict progression in patients with hormone-sensitive prostate cancer
Research Square (Research Square)(2023)
摘要
Objective Precise and appropriate diagnosis for prostate cancer patients can improve their quality of life. We sought to develop an innovative machine learning prognostic model to forecast the progression of hormone-sensitive prostate cancer (mHSPC). Methods A retrospective cohort study was conducted at Yunnan Cancer Hospital, including 533 patients diagnosed with hormone-sensitive prostate cancer between January 2017 and February 2023.In this machine learning model, K-proximity algorithm (KNN), naive Bayes, random forest algorithm, XGBoost and ADAboost were used to establish prediction models. The main evaluation indicators were the accuracy(ACC), precision༈PRE༉, specificity༈SPE༉, sensitivity༈SEN༉or regression rate ༈Recall༉and f1 score of the model. Results We established KNN, Naive Bayes, random forest algorithm, XGBoost and ADAboost models, and their accuracy rates were 75.4%, 71.1%, 88.02%, 86.6% and 85.2%, respectively.Among the generated models, XGboost has the highest accuracy of 88.02%. Conclusion Our model is more accurate and perfect than the predecessors, and can provide reference for clinical work.
更多查看译文
关键词
machine learning methods,prostate cancer,machine learning,hormone-sensitive
AI 理解论文
溯源树
样例
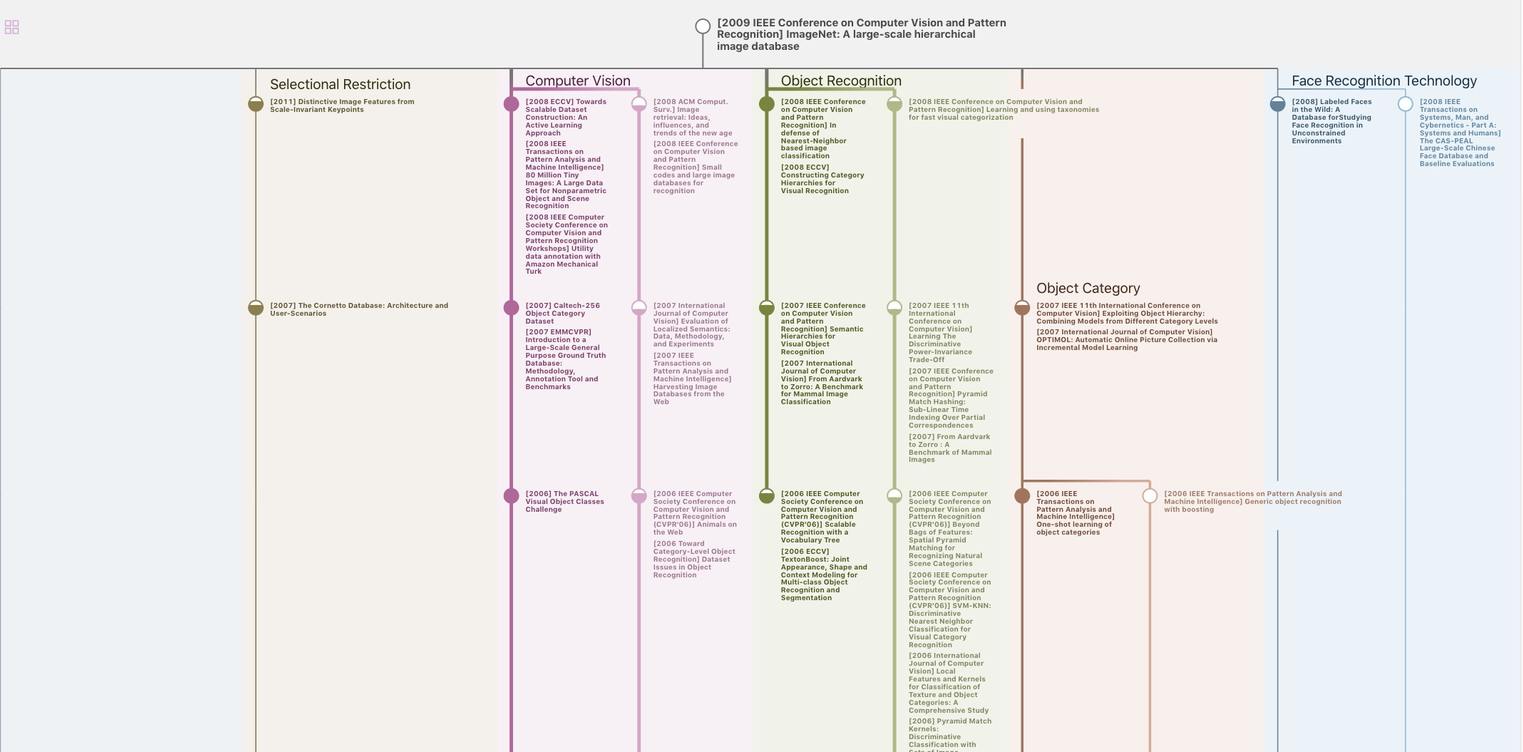
生成溯源树,研究论文发展脉络
Chat Paper
正在生成论文摘要