Idecomp: imbalance-aware decomposition for class-decomposed classification using conditional GANs
Discover Artificial Intelligence(2023)
摘要
Medical image classification tasks frequently encounter challenges associated with class imbalance, resulting in biased model training and suboptimal classification performance. To address this issue, the combination of class decomposition and transfer learning has proven to be effective in classifying imbalanced medical imaging datasets. Nevertheless, in order to further augment the performance gains achieved through the utilisation of class decomposition within deep learning frameworks, we propose a novel model coined imbalance-Aware Decomposition for Class-Decomposed Classification (iDeComp) model. By incorporating a conditional Generative Adversarial Network (GAN) model, iDeComp is capable of generating additional samples specifically tailored to underrepresented decomposed subclasses. This paper investigates the application of iDeComp using two different medical imaging datasets. iDeComp selects underrepresented samples from the training set of the sublevel classes within each dataset, which are then employed to train separate conditional Deep Convolutional GAN (DCGAN) models and verification models. The conditional DCGAN model is responsible for generating additional samples, while the verification model critically evaluates the appropriateness of the synthesised images. Subsequently, the resulting augmented samples are utilized to train the classification model. To assess the effectiveness of iDeComp, we employ various evaluation metrics including accuracy, precision, recall, and F1 score. The results obtained from our experiments clearly indicate that iDeComp outperforms existing approaches in terms of classifying both imbalanced datasets.
更多查看译文
关键词
conditional gans,classification,decomposition,imbalance-aware,class-decomposed
AI 理解论文
溯源树
样例
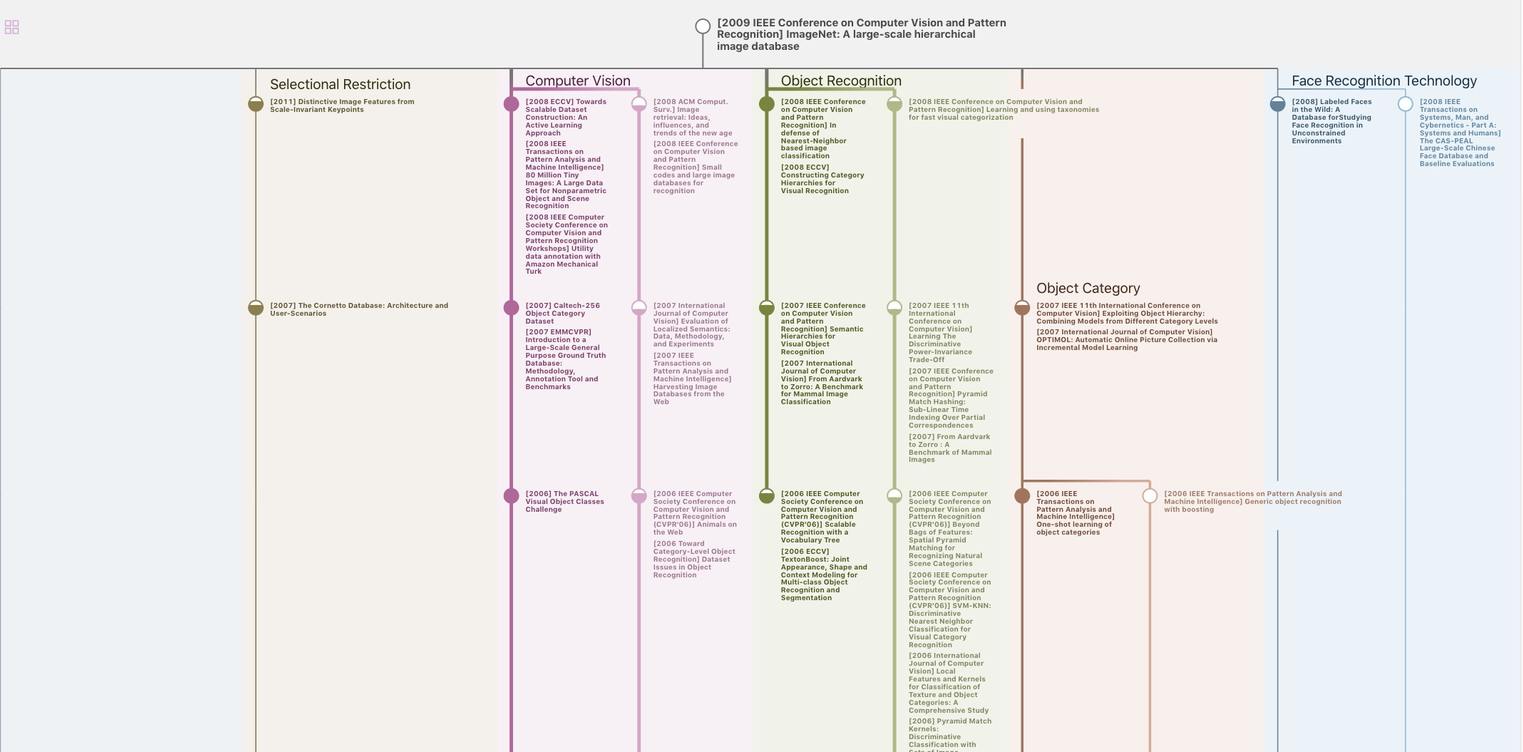
生成溯源树,研究论文发展脉络
Chat Paper
正在生成论文摘要