Screening for Severe Coronary Stenosis in Patientswith Apparently Normal Electrocardiograms Based Ondeep Learning
Research Square (Research Square)(2023)
摘要
Abstract Background Patients with severe coronary artery stenosis may have apparently normal electrocardiograms (ECGs), making it difficult to detect the adverse health conditions during screening or physical examinations, resulting in them missing the optimal window of treatment. Methods The goal of this study was to develop an effective model which can distinguish severe coronary stenosis from no or mild coronary stenosis in patients with apparently normal ECGs. Deep learning (DL) models trained from scratch and with pre-trained parameters (transfer learning) were tested on ECG alone as well as on ECG along with clinical information (age, sex, hypertension, diabetes, dyslipidemia and smoking status). Results We found that DL models trained from scratch with ECG alone can achieve a specificity of 0.746; however, they have low sensitivity, which is comparable to the performance of logistic regression with clinical data. Although adding clinical information to the ECG DL model trained from scratch can improve the sensitivity, it reduces the specificity. Combining clinical information with the ECG transfer learning model provides the best performance, with a 0.847 AUC, 0.848 sensitivity, and 0.704 specificity. Conclusion The findings not only demonstrate the effectiveness of using DL models to identify severe coronary stenosis with apparently normal ECGs, but also validate an efficient approach using existing ECG models. With transfer learning techniques, we can extract “deep features” that summarize the ECG’s inherent information with inexpensive calculations.
更多查看译文
关键词
severe coronary stenosis,screening
AI 理解论文
溯源树
样例
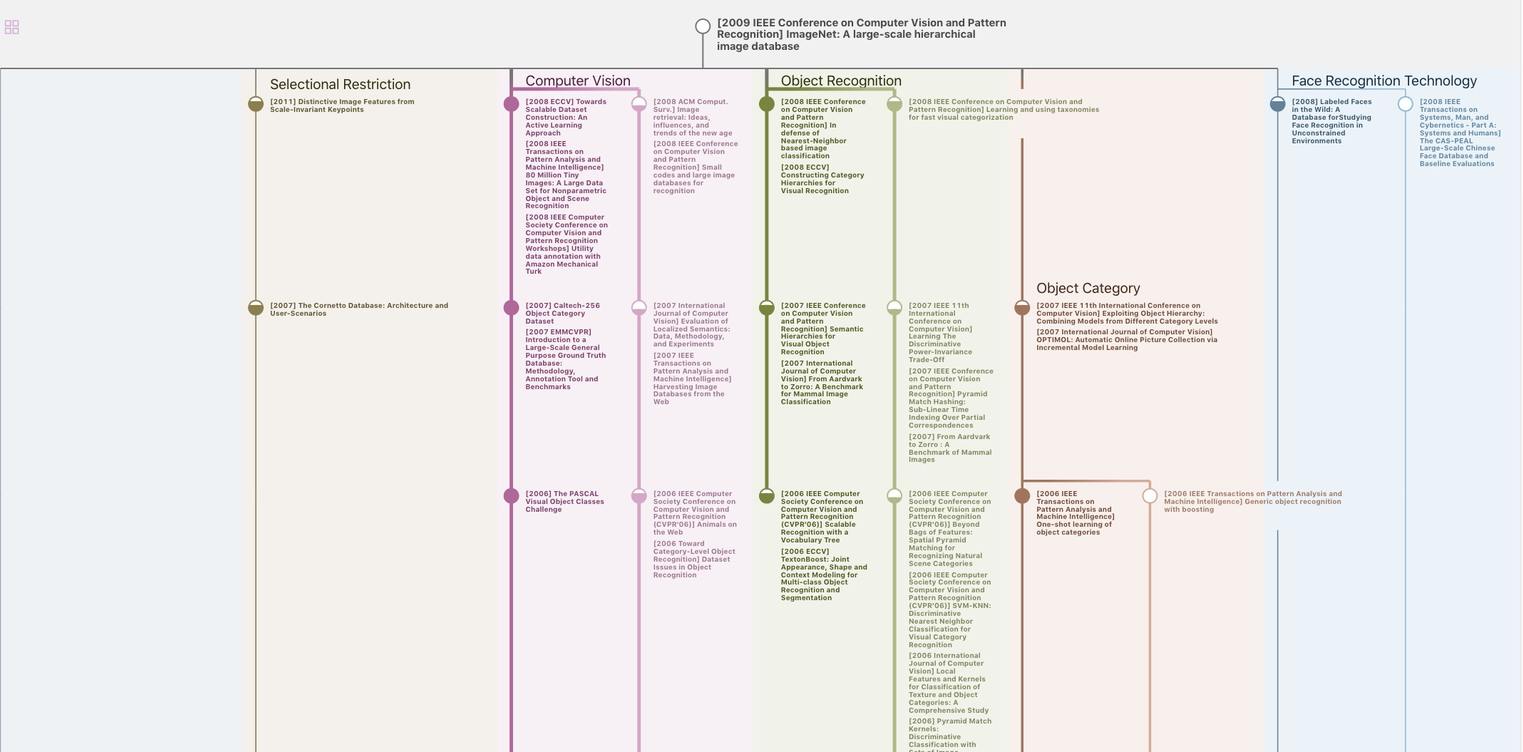
生成溯源树,研究论文发展脉络
Chat Paper
正在生成论文摘要