A data-driven approach to predict decision point choice during normal and evacuation wayfinding in multi-story buildings
arXiv (Cornell University)(2023)
摘要
Understanding pedestrian route choice behavior in complex buildings is important to ensure pedestrian safety. Previous studies have mostly used traditional data collection methods and discrete choice modeling to understand the influence of different factors on pedestrian route and exit choice, particularly in simple indoor environments. However, research on pedestrian route choice in complex buildings is still limited. This paper presents a data-driven approach for understanding and predicting the pedestrian decision point choice during normal and emergency wayfinding in a multi-story building. For this, we first built an indoor network representation and proposed a data mapping technique to map VR coordinates to the indoor representation. We then used a well-established machine learning algorithm, namely the random forest (RF) model to predict pedestrian decision point choice along a route during four wayfinding tasks in a multi-story building. Pedestrian behavioral data in a multi-story building was collected by a Virtual Reality experiment. The results show a much higher prediction accuracy of decision points using the RF model (i.e., 93% on average) compared to the logistic regression model. The highest prediction accuracy was 96% for task 3. Additionally, we tested the model performance combining personal characteristics and we found that personal characteristics did not affect decision point choice. This paper demonstrates the potential of applying a machine learning algorithm to study pedestrian route choice behavior in complex indoor buildings.
更多查看译文
关键词
evacuation,decision point choice,data-driven,multi-story
AI 理解论文
溯源树
样例
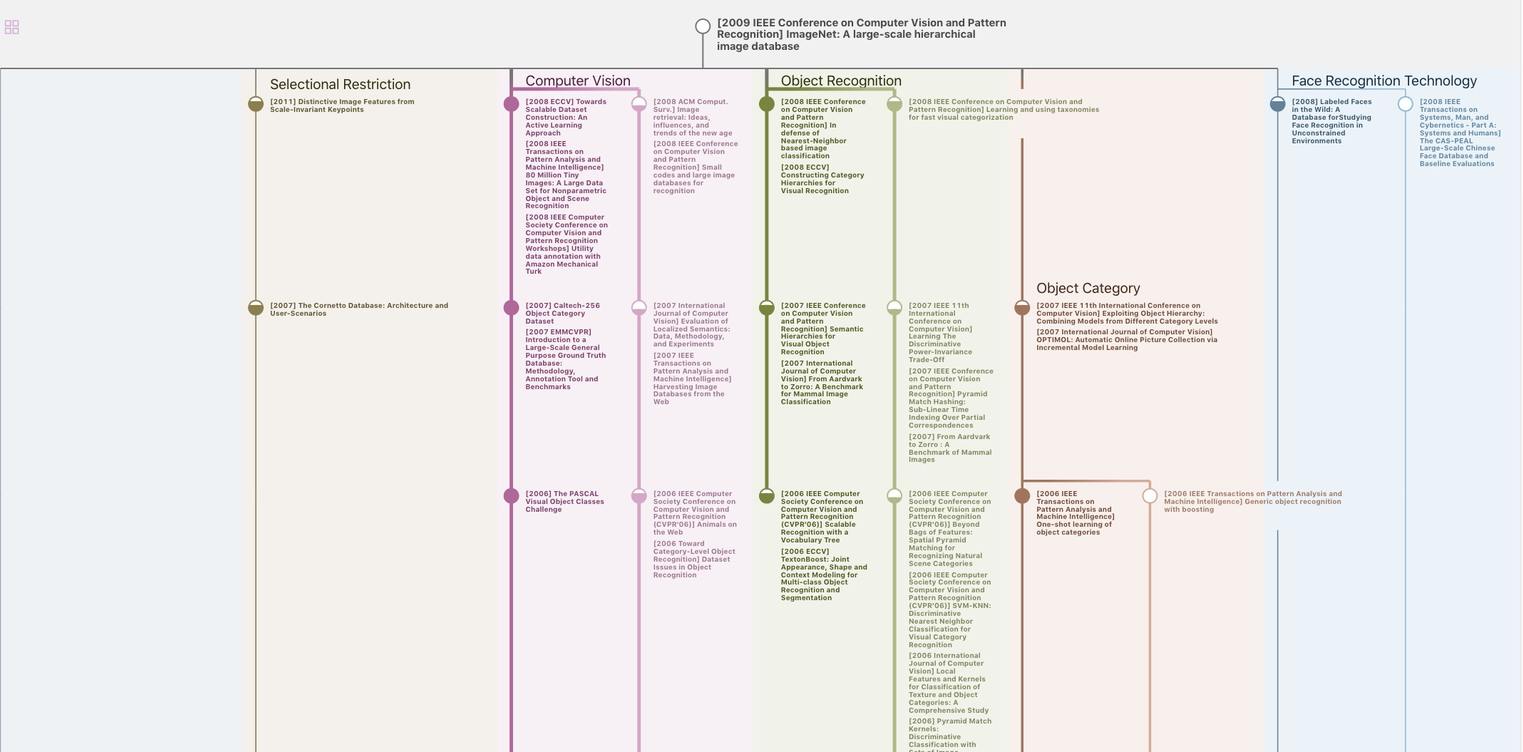
生成溯源树,研究论文发展脉络
Chat Paper
正在生成论文摘要