Artificial intelligence-based cancer progression prediction of oral premalignant lesions via self-supervised deep learning on histopathology.
JCO global oncology(2023)
摘要
90 Background: Premalignant lesions within the oral cavity demonstrate varied degrees of dysplasia. Oral stratified squamous epithelium is sensitive to carcinogenic insults and lesions progress to cancer at variable rates. The current diagnostic standard involves visual and tactile inspection followed by a biopsy. However, thus far, there is clinical ambiguity about which lesions may progress to carcinoma and there are insufficient prognostic tools available to guide clinical decision-making for follow-up. Deep learning is a form of artificial intelligence which can be applied to images. Self-supervised learning (SSL) and attention-based multiple instance learning (aMIL), are deep learning extensions which eliminate the need for pathologist annotations of histology and are more generalizable as the model learns general representations of unlabeled data, compared to traditional tile-based methods. We developed a novel artificial intelligence pipeline to predict progression to oral carcinoma using histological images from oral premalignant lesions. Methods: Digital histopathology images and clinical data cohorts were obtained from the US National Cancer Institute (n=561) and the University of Iowa (n=193) for training, and from the University of Chicago (n=214) for external validation. Patients were defined as progressing if initially non-malignant lesions had documented progression to carcinoma within 12 months of follow-up. Model training and evaluation was done in Python using our publicly available open-source Slideflow platform. We developed a deep learning pipeline utilizing SimCLR as an SSL method for feature extraction paired with an aMIL head for classification. The SimCLR feature generator was trained on only the training cohort, then used to generate features for both training and validation data. The aMIL model was trained on SimCLR-generated features. Trained model performance was evaluated on the external University of Chicago cohort and measured via areas under the receiver operating characteristic curve and precision recall curve (AUROC and AUPRC, respectively). During training and validation, model reliability was interpreted using Class Activation Mapping (Grad-CAM) and generative adversarial network (GAN) methods. Results: On training data, the model achieved average 3-fold cross validation AUROC of 0.86 with AUPRC of 0.83. In the external validation data from UC, the model achieved an AUROC of 0.80 with an AUPRC of 0.79. Deployed as a “rule-in” test for early progression prediction, the model achieved a validation data set specificity of 91% with a sensitivity of 40%. Conclusions: We developed a deep learning model to automatically predict cancer progression from premalignant lesions histopathology using a multi-institutional cohort, with promising external validation performance.
更多查看译文
关键词
cancer progression prediction,oral premalignant lesions,cancer progression,deep learning,intelligence-based,self-supervised
AI 理解论文
溯源树
样例
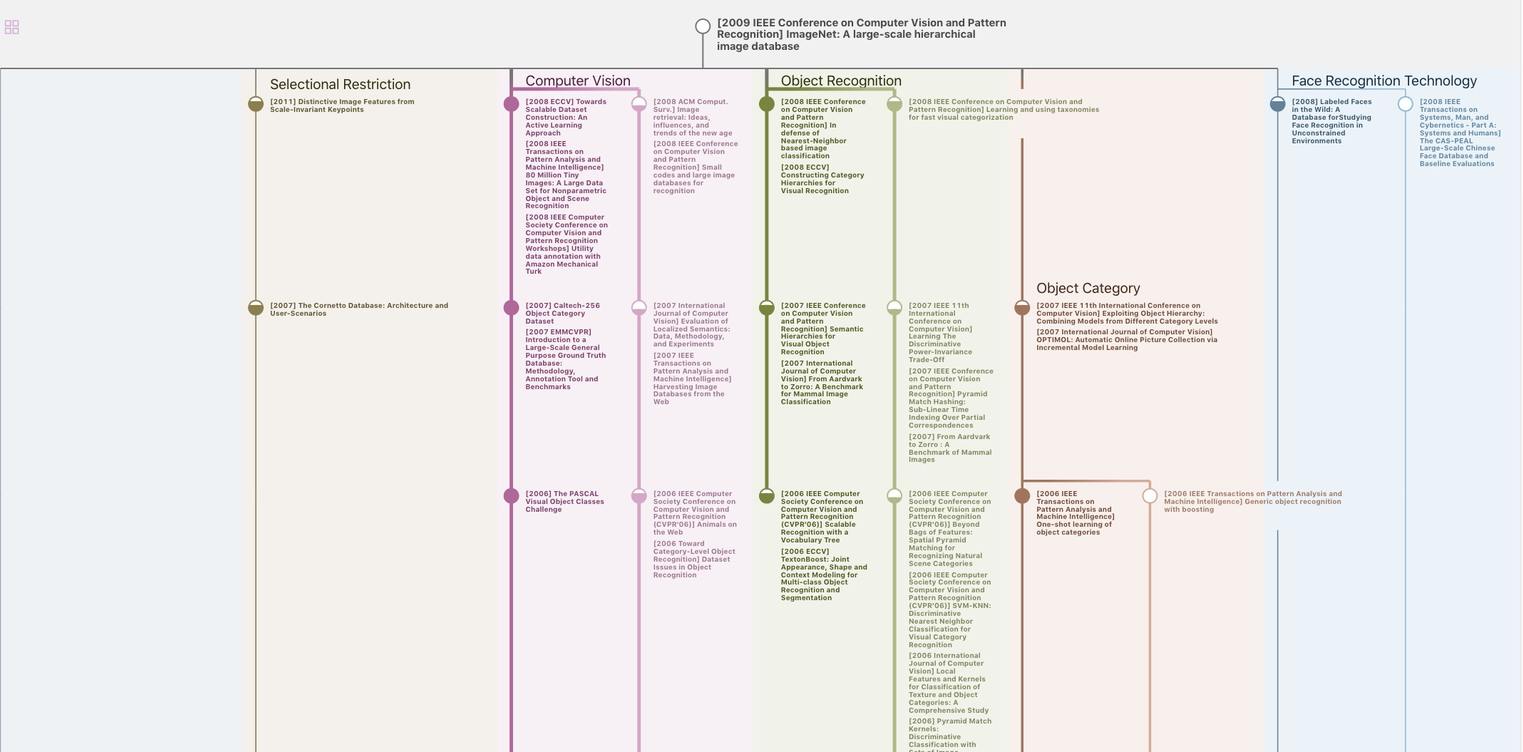
生成溯源树,研究论文发展脉络
Chat Paper
正在生成论文摘要