Poster 139: Utilization of 3D Statistical Shape Modeling of Anatomic Variation Across a Cohort of Patella Instability Patients
Orthopaedic Journal of Sports Medicine(2023)
摘要
Objectives: Patellar instability is a common condition that is responsible for 2-3% of all acute knee injuries in young athletes. Recurrent instability often requires corrective surgery. Surgical planning is challenging due to the wide array of anatomical risk factors associated with patellar instability. X-ray and magnetic resonance imaging (MRI) measures including the tibial tubercle to trochlear groove (TT-TG) distance, patellar tilt, Caton-Deschamps index (CDI), and lateral trochlear inclination angle (LTI) are used to characterize joint structure and guide surgical intervention. However, these 2D measures cannot fully describe the knee and face challenges in inter-rater reliability. Furthermore, these measures may be sensitive to patient positioning within the MRI scanner, leading to errors in treatment decisions. Statistical shape modeling (SSM) is a powerful computational method that quantifies complex 3D bone shapes. Previous research has used SSMs to predict the onset of osteoarthritis. While SSMs have been used to investigate trochlear dysplasia, these models have been based on a small number of asymptomatic knees. Thus, SSMs have not yet captured the variation seen in patellar instability cases. A 3D SSM of the bony knee anatomy, derived from a large cohort of patellar instability patients, is needed to accurately inform surgical planning. Our primary objective was to use data from a cohort of patellar instability patients to develop a statistical shape model of the bony knee anatomy and validate the model by its ability to explain variance in four clinical measures (TT-TG distance, patellar tilt, CDI, and LTI). Our secondary objective was to determine whether these measures correlate with uncontrolled knee position (extension and rotation) during clinical MRI. Methods: Dataset: Patients were identified from a published database that contained clinical confirmation of patellar instability. Proton-density weighted MRIs (Table 1) were acquired for 148 patients (14 ± 2 years old). Statistical Shape Model (SSM): We trained a 2D U-Net deep learning model to automate segmentations of the femur, patella, and tibia using 3730 slices from 11 manually segmented patient MRIs. After validating the model on 540 slices from 2 manually segmented MRIs, we generated surface meshes of each bone and found point correspondences between patients using our fully-automated, open-sourced algorithm. We applied principal component analysis (PCA) to the corresponding points to generate the SSM. PCA yields independent principal component (PC) shape features that explain variance in bone shape. Our analysis included the first 20 PCs, which explain 95% of the dataset’s variance. We scored each knee along every PC to quantify how many standard deviations its shape was from the average knee in our patellar instability dataset. Clinical Measures: Our dataset included measurements by one of two radiologists for each patient’s TT-TG distance, patellar tilt, and CDI. We measured LTI separately on each MRI using the 2- image LTI protocol. Statistical Analysis AIM 1: Explaining Variation in Clinical Measures to Test SSM. We used linear regression with backward elimination, using PC scores as input features. To interpret our findings, we calculated the R 2 values between the SSM-derived estimates and human measurements, and we compared these to R 2 values between the two radiologists. The latter was collected for a previous reliability study and served as an upper bound on our potential R 2 . AIM 2: Correlating Clinical Measures to Joint Positioning. We first identified which SSM PCs reflected gross knee position. To do this, we visualized each PC morphing from -3 to +3 standard deviations from the average knee in the dataset and visually identified which PCs maximally reflected knee position changes. We then calculated Pearson correlations between these identified knee position PCs and each clinical measure, with |r| < 0.3 poor, 0.3 - 0.6 fair, 0.6 - 0.8 moderately strong, and > 0.8 very strong. Results: Our final analysis included MRIs from 110 patients after removing 38 patients with automatic segmentation errors. Age and MRI sequence parameters of this subset did not differ significantly from the larger cohort (p > 0.49). AIM 1: Linear regression revealed SSM estimations that included 3 to 7 PCs as input features and explained a fair to moderate amount of variance in clinical measures (Figure 1). In all cases, the R 2 between the model estimates and human measurements was only slightly less (0.03-0.10) than the R 2 between two radiologists (R 2 ). AIM 2: We identified that PC 1 reflected knee extension, and PC 2 reflected knee rotation and trochlear dysplasia. TT-TG distance and patellar tilt were fairly correlated with both PCs (r = 0.37 - 0.57, Figure 2), likely due to lateral shifts of the patella and tibia throughout extension. LTI was also fairly correlated with PC 2 (r = -0.43), likely due to the shallowing of the trochlea. Conclusions: Our study implemented and tested an automated deep-learning-based segmentation and statistical shape model (SSM) pipeline to describe the bony knee anatomy of 110 patients with patellar instability. Our model demonstrated an ability to learn, unsupervised, salient features of patellar instability that can explain variance in image-based anatomic measures at levels similar to radiologists. Our model further demonstrated that TT-TG distance and patellar tilt are associated with varying knee position in the MR scanner, consistent with previous findings. Future work will test our model’s ability to control for position while predicting clinical outcomes. We will also utilize our validated SSM to guide future pre-surgical planning by using clinical outcome data from patients with specific 3D anatomic features. [Table: see text]
更多查看译文
关键词
3d statistical shape modeling,anatomic variation
AI 理解论文
溯源树
样例
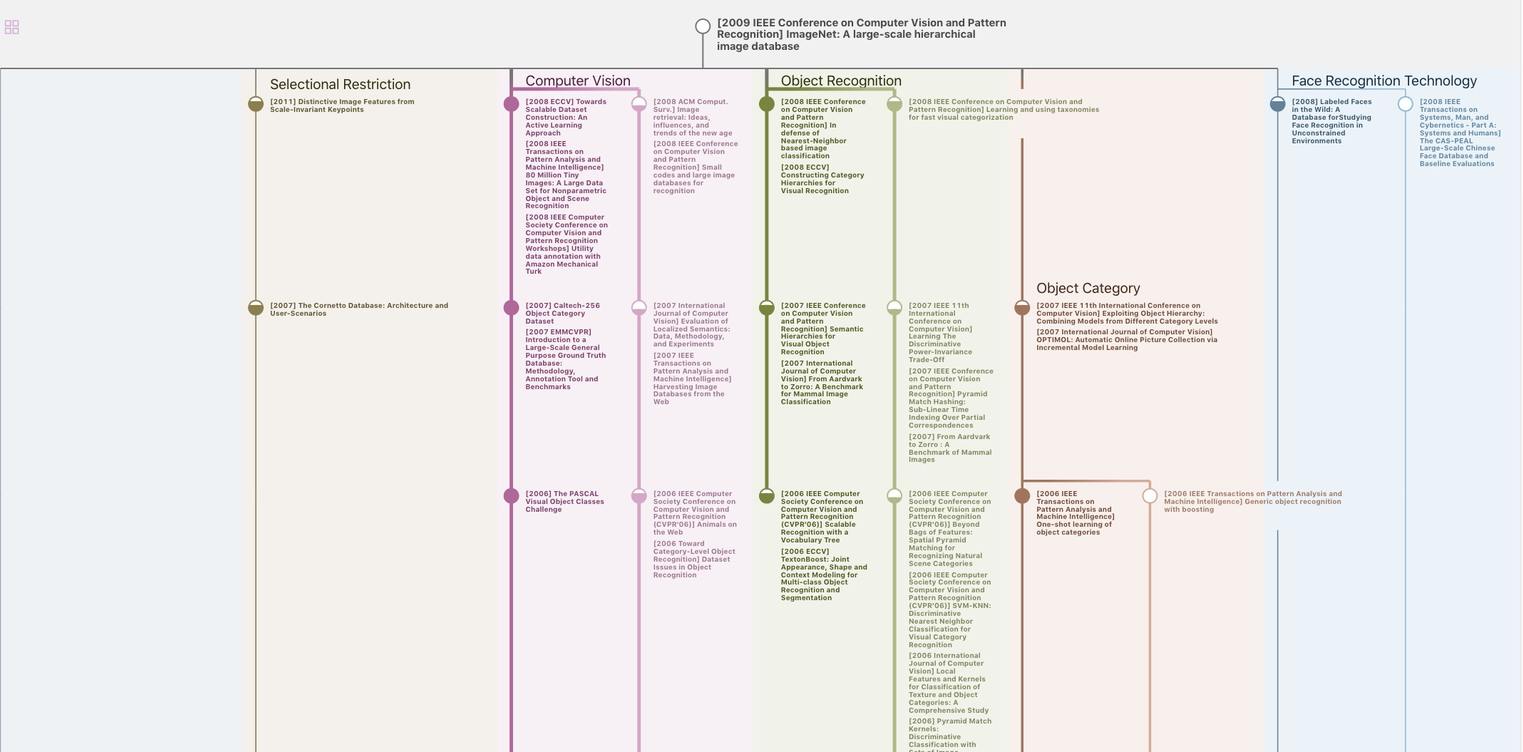
生成溯源树,研究论文发展脉络
Chat Paper
正在生成论文摘要