External Evaluation of a Mammography-based Deep Learning Model for Predicting Breast Cancer in an Ethnically Diverse Population
Radiology(2023)
摘要
“Just Accepted” papers have undergone full peer review and have been accepted for publication in Radiology: Artificial Intelligence. This article will undergo copyediting, layout, and proof review before it is published in its final version. Please note that during production of the final copyedited article, errors may be discovered which could affect the content. Purpose To externally evaluate a mammography-based deep learning (DL) model (Mirai) in a high-risk, racially diverse population and compare its performance with other mammographic measures. Materials and Methods The authors retrospectively evaluated 6,435 screening mammograms acquired from 2,096 female patients (median age, 56.4 years [SD: 11.2]) enrolled in a hospital-based case-control study between 2006–2020. Pathologically-confirmed breast cancer was the primary outcome. Mirai scores were the primary predictors. Breast density and Breast Imaging Reporting and Data System (BI-RADS) assessment categories were comparative predictors. Performance was evaluated using area under the receiver operating characteristic curve (AUC) and concordance-index analyses. Results Mirai achieved one-and five-year AUCs of 0.71 (95% CI: 0.68, 0.74) and 0.65 (0.64, 0.67), respectively. 1-year AUCs in nondense versus dense breasts were 0.72 versus 0.58 (P = .10). There was no evidence of a difference in near-term discrimination performance between BI-RADS and Mirai (1-year AUCs, 0.73 versus 0.68, P = .34). For longer-term prediction (2–5 years), Mirai outperformed BI-RADS assessment (5-year AUC, 0.63 versus 0.54; P < .001). Using only images of the unaffected breast reduced the discriminatory performance of the DL model (P < .001 at all timepoints), suggesting that its predictions are likely dependent on the detection of ipsilateral premalignant patterns. Conclusion A mammography DL model showed good performance in a high-risk external dataset enriched for African American patients, benign breast disease, and BRCA mutation carriers, and study findings suggest that its performance is likely driven by the detection of precancerous changes. ©RSNA, 2023
更多查看译文
关键词
deep learning model,breast cancer,deep learning,mammography-based
AI 理解论文
溯源树
样例
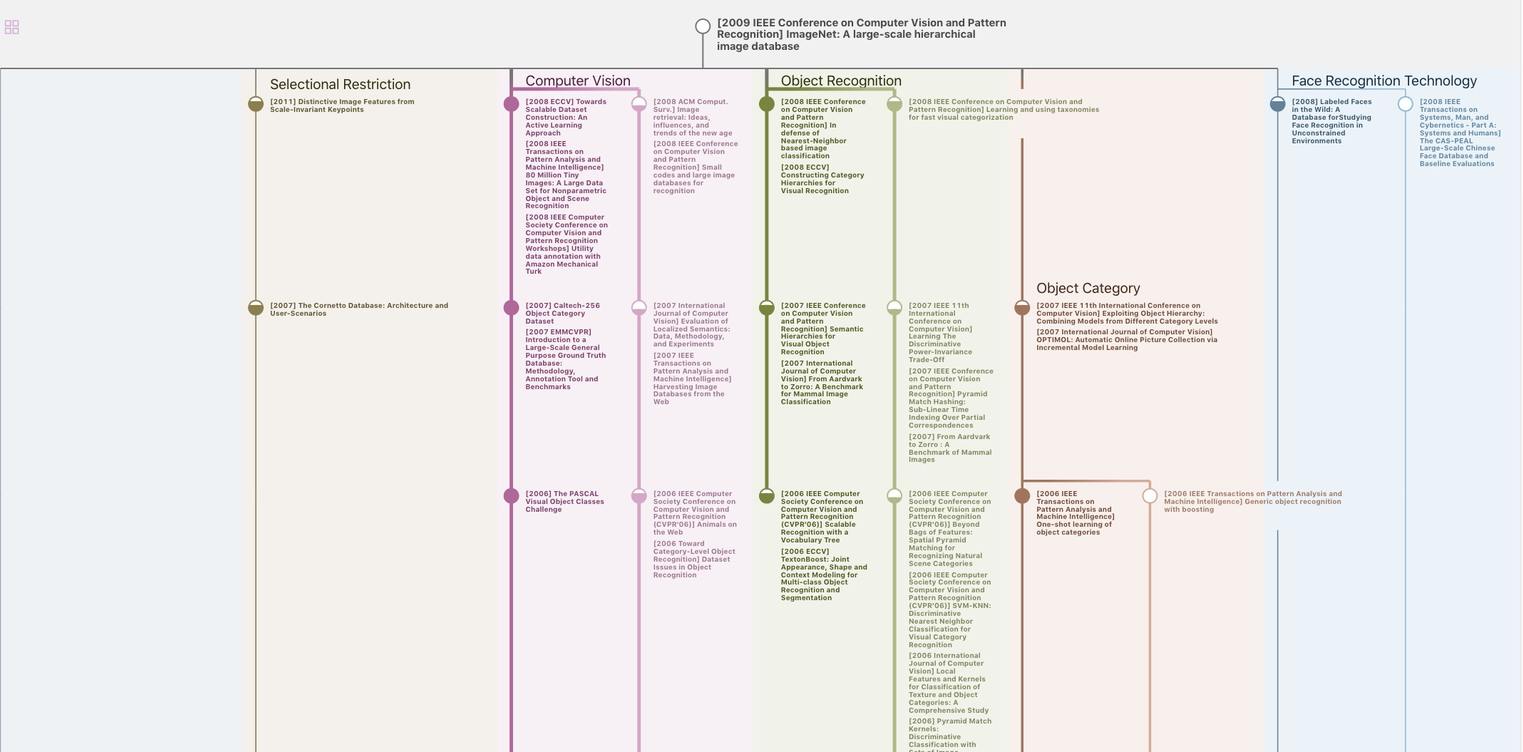
生成溯源树,研究论文发展脉络
Chat Paper
正在生成论文摘要