Multi-fidelity Bayesian Optimisation of Reactor Simulations using Deep Gaussian Processes
Computer-aided chemical engineering(2023)
摘要
Coiled tube reactors under pulsed-flow conditions have been shown to provide promising mixing characteristics. In order to validate performance in an industrial setting, and investigate the underlying physics of successful mixing, coiled tube reactors must be optimised. In this work, we apply a novel framework to locate optimal solutions to this nonlinear, computationally expensive, and derivative-free problem. Our optimisation framework takes advantage of deep Gaussian processes to learn a multi-fidelity surrogate model. We apply this model within a novel Bayesian optimisation algorithm, using faster, less accurate, and potentially biased lower-fidelity simulations to enable faster reactor optimisation. We subsequently investigate the physical insights into the swirling flows of these optimal configurations, directly informing the design of future coiled-tube reactors under pulsed-flow conditions. We demonstrate our design framework to be extensible to a broad variety of expensive simulation-based optimisation problems, supporting the design of the next-generation of highly parameterised chemical reactors.
更多查看译文
关键词
deep gaussian processes,reactor simulations,multi-fidelity
AI 理解论文
溯源树
样例
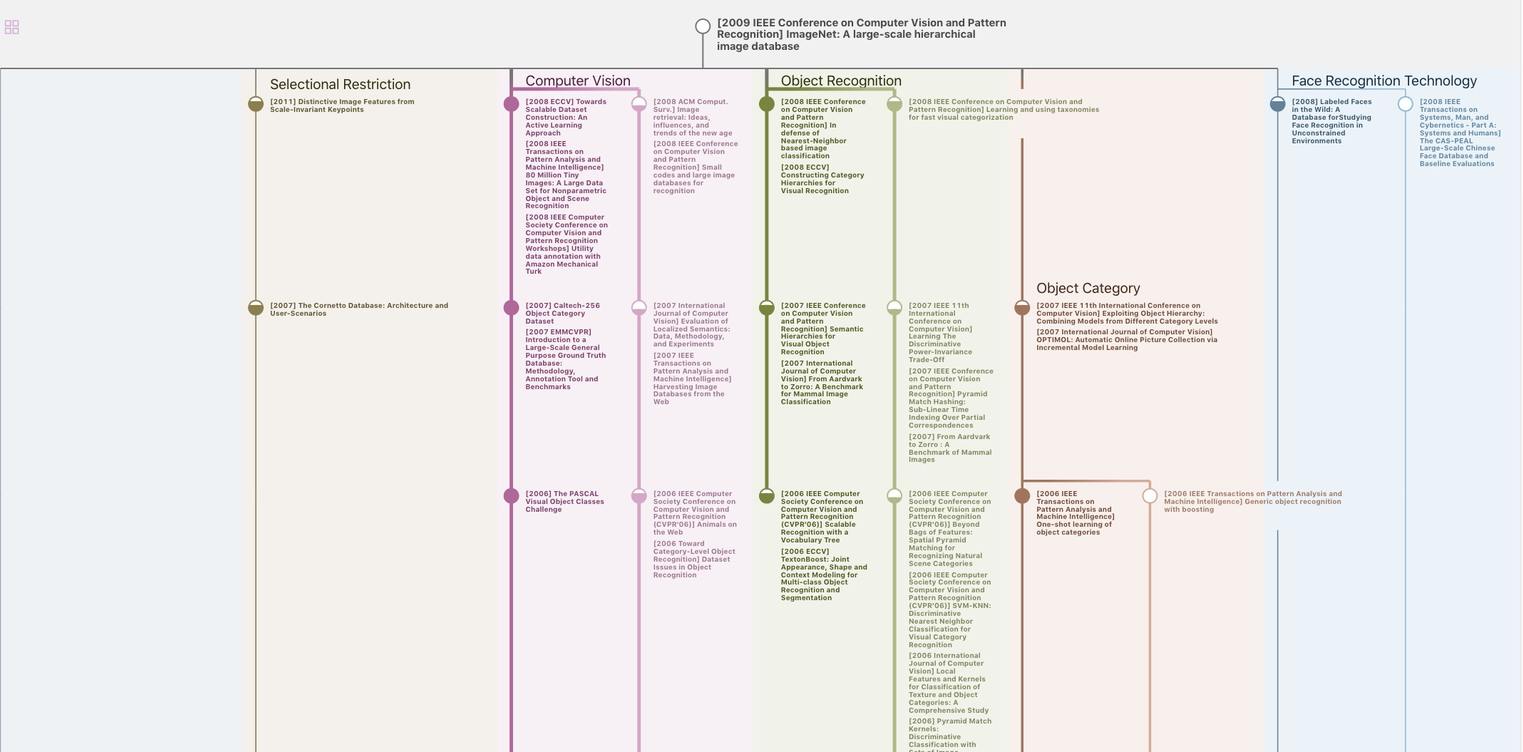
生成溯源树,研究论文发展脉络
Chat Paper
正在生成论文摘要