Deep Reinforcement Learning for RIS-Aided Secure Mobile Edge Computing in Industrial Internet of Things
IEEE TRANSACTIONS ON INDUSTRIAL INFORMATICS(2024)
摘要
Mobile edge computing (MEC) has been regarded as a promising paradigm to support the compute-intensive and delay-sensitive industrial Internet of things (IIoT) applications. However, the nature of broadcasting in wireless communications may cause that the task offloading security is easy to be threatened from eavesdroppers. Aiming at improving the task offloading security, this article studies the benefit of deploying the emerging reconfigurable intelligent surface (RIS) in MEC-enabled IIoT networks with eavesdroppers, and forms the RIS-aided secure MEC system with time-division multiple access. In addition, we formulate a joint RIS phase shift, power control, local computation rate, and time-slot allocation optimization problem to maximize the weighted sum secrecy computation efficiency (WSSCE) among IIoT devices. To address this intractable problem, we propose a deep reinforcement learning (DRL)-based algorithm, where a deep deterministic policy gradient (DDPG) agent is adopted. Numerical results demonstrate that 1) deploying the RIS can improve the WSSCE performance; 2) the proposed DDPG-based algorithm can obtain higher WSSCE than other baseline methods.
更多查看译文
关键词
Industrial Internet of Things,Resource management,Task analysis,Wireless communication,Power control,Energy consumption,Mathematical models,Deep reinforcement learning (DRL),industrial Internet of things (IIoT),mobile edge computing (MEC),reconfigurable intelligent surface (RIS),secure offloading
AI 理解论文
溯源树
样例
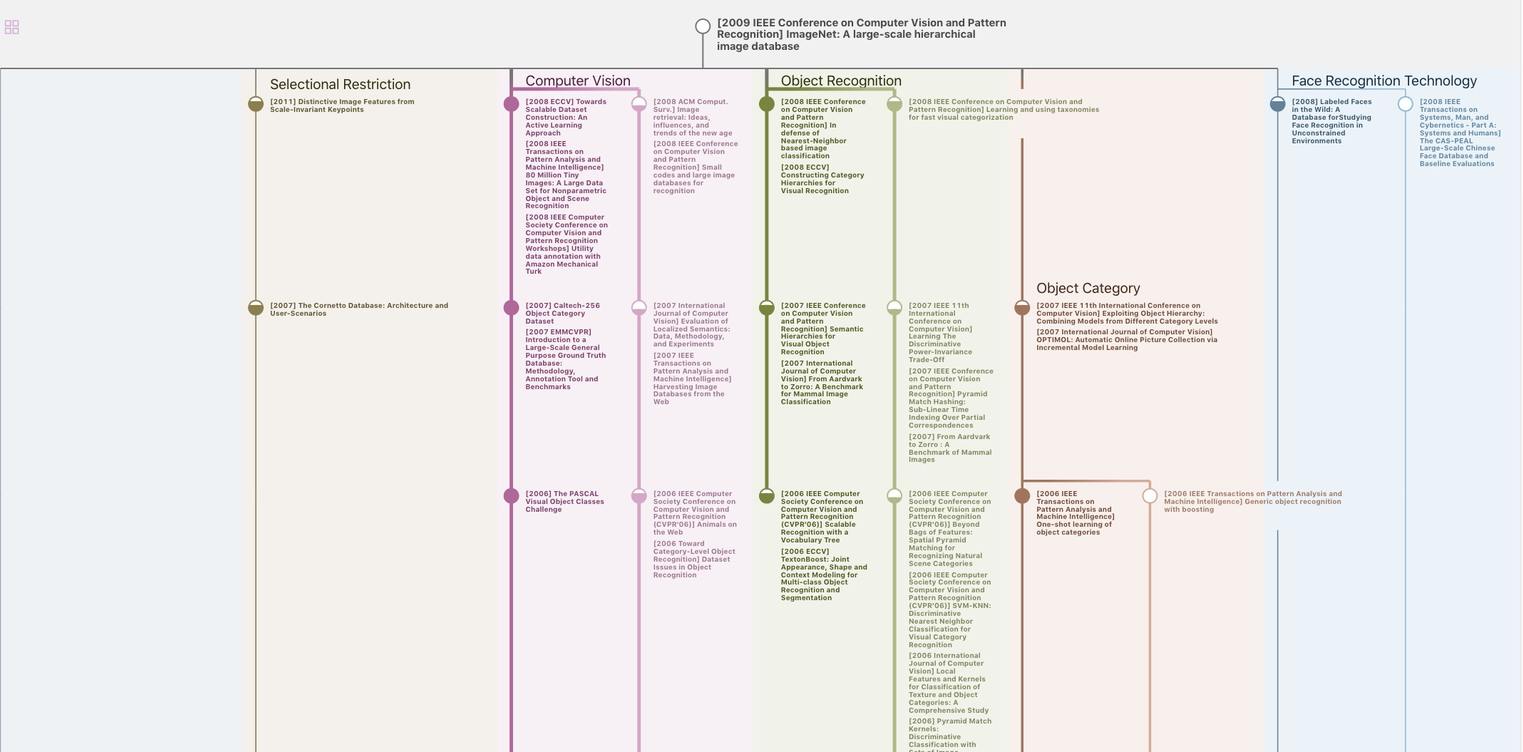
生成溯源树,研究论文发展脉络
Chat Paper
正在生成论文摘要