Application of an oversampling method based on GMM and boundary optimization in imbalance-bearing fault diagnosis
IEEE TRANSACTIONS ON INDUSTRIAL INFORMATICS(2024)
摘要
Synthetic minority oversampling (SMOTE) has been widely used in dealing with the imbalance classification in the mechanical fault diagnosis field. However, the classical SMOTE model generates poor quality data, which leads to a low diagnostic accuracy of the classification model. This paper proposes an oversampling generation model based on the Gaussian mixture model (GMM) and boundary joint optimization (BDOP-GMM-SMOTE). Firstly, GMM is utilized to cluster minority class bearing fault data, and weights of different classes should be distributed according to the cluster density distribution function (CDDF). Then, the minority classes should be resampled to balance intra-class. Thirdly, the data boundary is established by calculating intra-class and inter-class distances between the minority class and other failure-class samples. And penalty coefficient (PC) is introduced to optimize data generation boundary by minimizing intra-class and maximizing inter-class principle. Afterward, a generated fault dataset satisfied intra-class and inter-class balance is obtained. Finally, the generation effectiveness and robustness of the proposed method is verified by bearing experiment data, especially in diagnostic accuracy and processing speed.
更多查看译文
关键词
Imbalanced data,SMOTE,Cluster density distribution function (CDDF),Gaussian mixture model (GMM),Boundary optimization (BDOP),Fault diagnosis
AI 理解论文
溯源树
样例
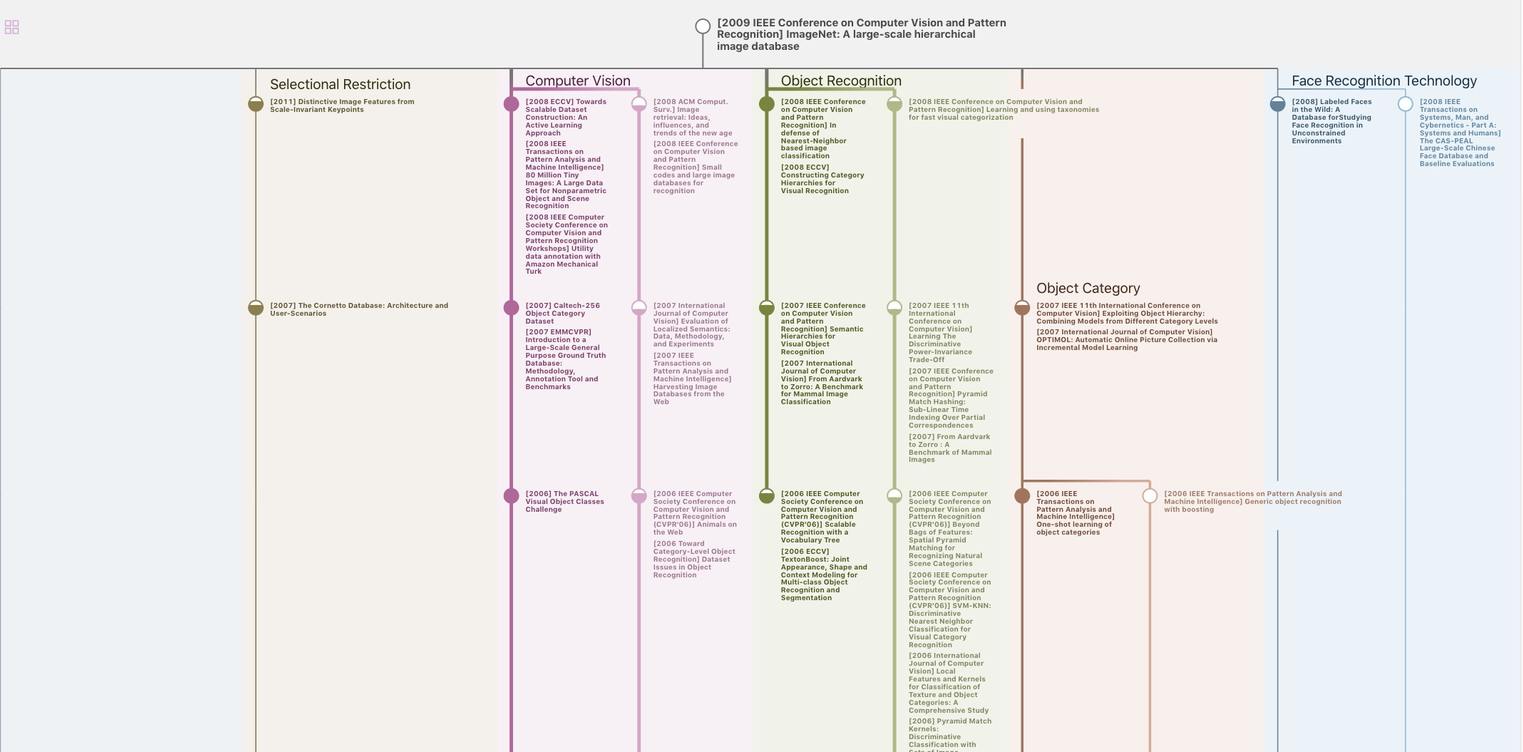
生成溯源树,研究论文发展脉络
Chat Paper
正在生成论文摘要